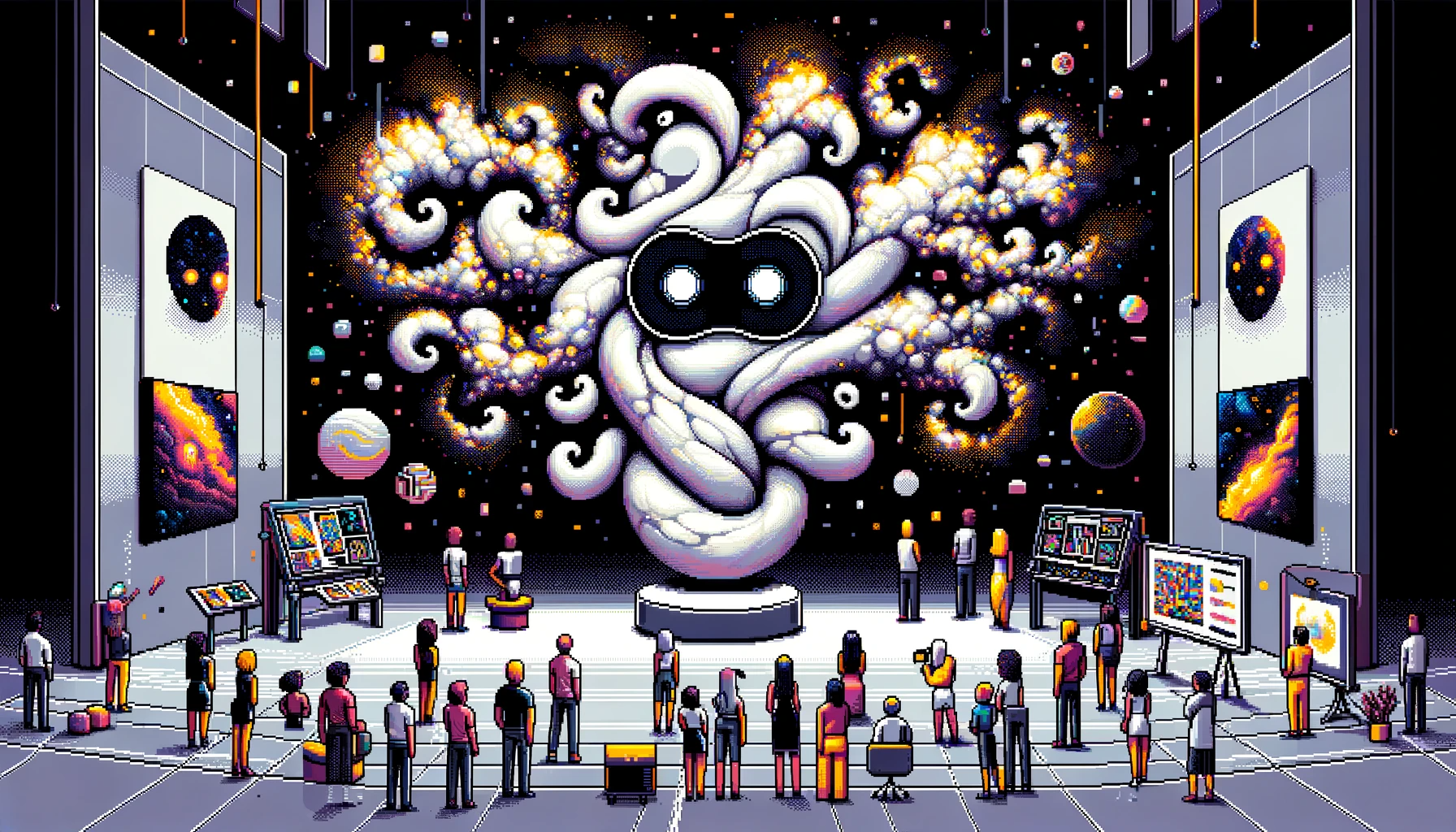
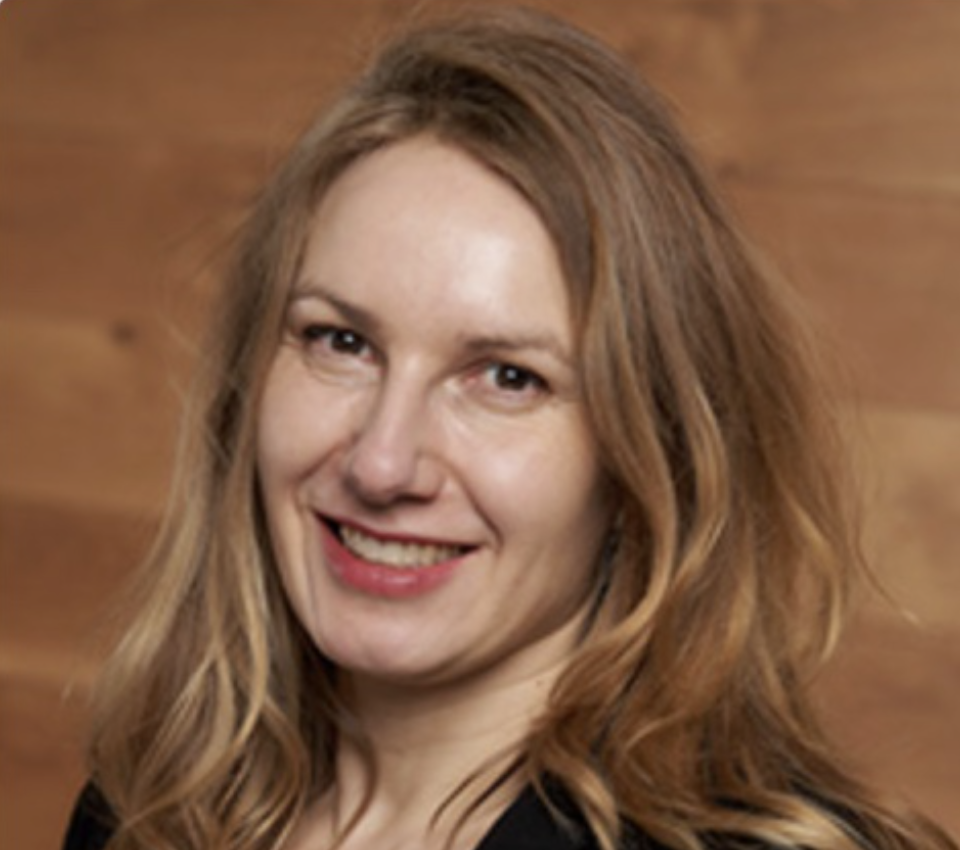
🔬 Research Summary by Jessica Hullman, the Ginni Rometty Associate Professor of Computer Science at Northwestern University, where she leads a research program to develop visualization tools, interactive protocols, and theoretical frameworks to address challenges and limitations that arise when people theorize and draw inductive inferences from data.
[Original paper by Jessica Hullman, Ari Holtzman, and Andrew Gelman]
Overview: As generative AIs like chatbots capture attention across domains, everywhere we seem to be witnessing the same kind of beauty contest, where the output of a generative model is held up to some assumed human standard and judged to be inferior to it. But what enables us to make such judgments so confidently? This article considers how our interactions with modern generative AI inherit tendencies when we attempt to read meaning in works of art, which are far from naturally endowed. We outline some of the kinds of incoherence in our interpretations that we should expect to run as a result.
Introduction
In the history of artificial intelligence (AI), the Turing Test represents one standard for judging progress: Can a human evaluator tell the difference between the conversational responses of a human and a machine? But these days, we frequently see a less formal mode of evaluation, where a kind of aesthetic judgment is brought to bear on the output of a generative AI to declare its significance as an artifact of human expression.
For example, a famous author recently considered ChatGPT’s attempt to explain his reasons for writing a book. Dissecting the chatbot’s comments, he concluded that despite the text appearing at first to be “convincing and true” or “noble sounding,” instead, its words were “vague,” “vapid pablum.” “[W]hen it comes to using language in a sensitive manner and talking about real-life situations,” he concluded, it makes no sense whatsoever to be using chatbots; in fact, the “artificiality of the creation runs counter to [his] lifelong belief system.”
This kind of aesthetic judgment is not unique to generative AI, though. Consider the frame of mind assumed when we visit a museum and stand before a work of art, attempting to take in its essence and determine its significance. We might think we are born understanding how to “read” works of art, but far from it. The idea of having good taste in art emerged over time as a reaction to new modes of creative production. Aesthetic judgments are a way of distancing us from scary new developments in our ability as a species to represent the world around us.
Our article connects overlooked features of our judgments of AI with what we know about aesthetic judgment from art history and critical museology. Looking at our interactions with generative AI through this lens of judgments about art exposes weaknesses in the new philosophical arguments we are working to build. For example, a fear of creative objects that might act in the world led to the birth of the idea of “taste” in art. We see a similar urgency in defining the right critical perspective on generative AI. However, as soon as an AI is shown capable of engaging in some behavior that was previously considered human or intelligent, we move the bar so that we can only define good AI as we can only define good art as what it is not. Ultimately, establishing cause and effect becomes impossible when our judgments of AI inherit from how our judgments of art combine multiple ways of reading objects (e.g., as metaphors for human essence versus as culturally distinct messages about their creators) in a single interpretation system.
Key Insights
We would like—or perhaps more accurately, we feel we need—to make AI-generated media legible to current interpretive modes, the way governments insist on predefined categories
for businesses or marital relationships to make human organizations legible to the state. But the kind of reading we seek is more than what we can get from simply reading the text generated by a chatbot. Around us is an urgency to understand the significance of these impressive new machines.
Reflect for a moment on how we “read” a piece of art that we stand before in a museum setting. Many of us dutifully read the placards next to each piece, which help us contextualize the work by locating it and its author within a broader artistic genealogy. At the same time, we are encouraged to see beyond the specific materials and their historical relevance to the work’s less tangible “essence” to sense the elusive truth that renders museum-worthy works that achieve a certain authenticity of expression. This dual orientation, where meaning is read into works of art via metonymic and metaphorical relationships, is so ingrained that we hardly stop questioning it.
What happens when we bring the same dual orientation we bring to works of art to our judgments of generative AIs?
It has become common to contrast differences between the “origins” of models created at different times by different groups—in the form of details about their training data and its scale (Denton et al., 2021), training process (Bengio et al., 2013), or model size in parameters (Bommasani et al., 2021)—to locate them in the broader landscape of generative AI progress, and to prescribe processes that will render them more legible (e.g., transparency). Similar to how art historians interpret the properties of artistic techniques as evidence of the mentality of a culture, e.g., the subdued color schemes and sharp finish of Dutch still life paintings, critical scholars develop theories of what general properties of models pursued in deep learning research, like scale and efficiency, say about the values of the cultures that create them (Birhane et al., 2022).
At the same time, just like it would seem unnatural to take in a piece of art without trying to judge how well it has achieved some exalted human “essence,” so too it would seem unnatural to try to separate our natural orientation to look for some greater meaning in our interactions with the latest generative AIs. Today’s encounters with generative AIs would often seem to be a kind of aestheticized Turing Test: rather than merely asking, “Could a human produce something like this?” we look at the output of a generative AI for signals of ”humanness” as defined some internal idealization we presume ourselves to have access to. We can’t help but expect the exaltation of human intelligence from AI like we expect the exaltation of the human spirit from art. When what we see falls short, we feel the same sort of aesthetic dismay that the art critic feels when faced with something tacky.
However, there are consequences beyond a sense of tackiness when our interpretations of generative AIs inherit from these two very different approaches to interpreting objects. While stories of how AIs express values and emergent human qualities may be productive for driving scholarship and popular debate, they present us with a conundrum of causal inference. Drawing the boundaries of what belongs in the story of a generative AI’s meaning and what does not is a hopeless task. If the enabling assumption of art history and museology is that “changes in form (or a lack thereof) are taken to correspond and reflect or embody changes (or a lack of change) in beliefs, attitudes, mentalities, or intentions, or to changes (or not) in social, political, or cultural conditions” (Preziosi, 2003), then the aesthetic object can be read as a symptom of anything and everything that plausibly be thought to contribute to its appearance, at the same time that it invokes a seemingly natural reading of it as emblematic of some universal essence. Consequently, we find ourselves reliant on what appears to be a science of cause and effect, yet one that resists any attempts to fix a causal interpretation.
Between the lines
By recognizing where our interactions with novel technologies borrow from much older ways of reading objects, we can reflect on where our need for aesthetic judgment might take us when it comes to generative AI. For example, one implication of our analysis is that our appraisals of the significance of the latest progress in generative AI are unlikely to “price in” even in the near future with the same value that we believe them to hold today.
Despite the urgency to put modern AI in its place in some larger unfolding story of humankind and its creation, we should expect the nature of our aesthetic judgments to change with the technology. In the history of art, this has always been the case. But how exactly our judgments will change with the technology is an open question. What happens, for example, when the critic–the one prompting the generative model–no longer needs the artist to fuel aesthetic judgment? Who will be blamed for the content that gets produced in bad taste, the “artist” or the critic? Does the science of generative artificial intelligence need the equivalent of professional art critics, similar to the value of professional critics of science in general? Or is this a temporary moment because our interactions with state-of-the-art AI occur through “chats” that are mostly removed from the rest of life (similar to an art museum)? Whatever the case may be, we should expect our tendency to fall back on aesthetic judgment to carry philosophical consequences.