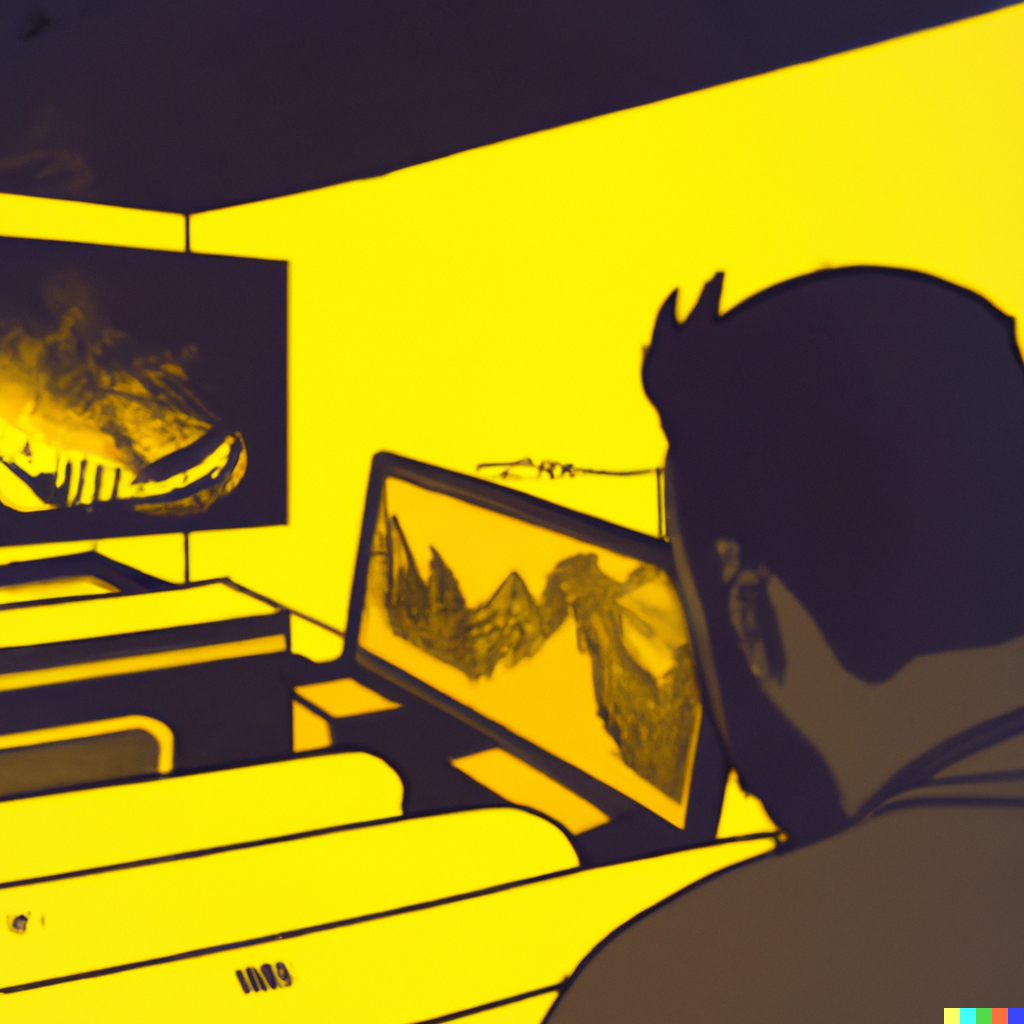
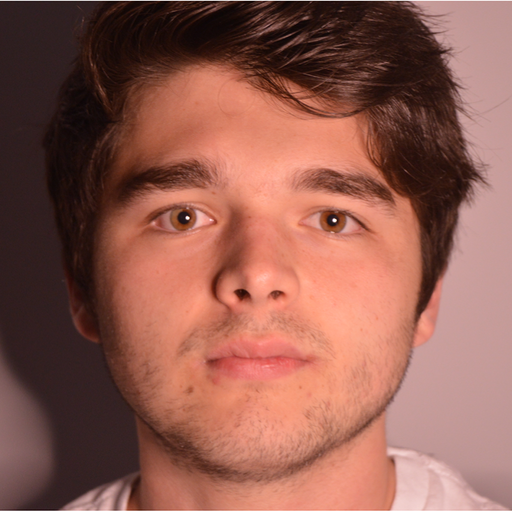
🔬 Research summary by Connor Wright, our Partnerships Manager.
[Original paper by Yu-Leung Ng]
Overview: Does the trustworthiness of the politician as the subject of a deepfake video affect how trustworthy we think the video is? Would a description of a deepfake that accompanies a video help? This study explores just how testing deepfakes can be, with the crux of the learning found in the public perception of the politician.
Introduction
Deepfakes are digitally-tinkered videos, using neural networks to feed on video data to impersonate the video subjects. They have the potential to weaken a shared sense of social reality as well as be used in vengeful ways (such as in making sexual content). However, it has also been found that participants in deepfake studies are surprisingly adept at identifying the deepfake video. Hence, this study focuses on deepfakes aiming to impersonate politicians.
Comprising 631 participants, the study segmented them into four different areas. Here, 148 participants were faced with deepfake videos with a description (of what a deepfake is), 157 with deepfake videos without a description, 143 with real videos with a description, and 153 real videos without a description. For each one, a deepfake and real Trump video and a deepfake and real Obama video were shown. After each video, participants were asked about the fakeness of the video and to what extent the video is positive (trustworthy) and negative (dangerous). Participants were asked to rate on a scale of 1-7 (with 1 as strongly disagree, 4 being neutral, and 7 being strongly agree) the statements that came after each video, such as “This video is fake” and “What Mr. Trump said in this video is fake.”
Key Insights
Within the study, error management theory explains the four possible outcomes of a decision:
- person A makes a decision based on true information (true positive),
- person A does not make a decision as the information they have is false (true negative),
- person A makes a decision based on false information (false positive, type 1 error),
- person A does not make a decision even when the information is true (false negative, type 2 error).
The error management theory explains that the last two errors are generally made under uncertainty. The error management theory highlights how humans usually opt for the decision, bringing about the least costly error when unsure of the correct option.
With this in mind, the authors distinguished two types of error: a type 1 error consists of treating a video with negative content as true (false positive). A type 2 error involves not trusting a trustworthy source (false negative). Consequently, each error would be operationalized through 4 different hypotheses:
Type 1 errors
- Hypothesis 1: the more negative the content of a video (without a description) is, the more likely it will be perceived as fake in terms of a) its video and b) its message.
- Hypothesis 2: participants will view a negative video with a description as fake regarding a) its video and b) its message compared to positive videos.
Type 2 errors
- Hypothesis 3: positive video targets motivate the participants to perceive a) the video and b) the message as trustworthy, even when the video is a deepfake.
- Hypothesis 4: positive video targets motivate the participants to perceive a) the video and b) the message as trustworthy, even when the video itself is a deepfake and has a description.
Results
- The findings supported hypotheses 1, 2, and 3, but not 4.
- Participants can decipher a deepfake from a real video – participants were able to identify the fake Trump and Obama messages and videos. However, type 1 and type 2 errors are still made.
- Deepfake messages from Obama, alongside deepfake videos and messages from Trump, were accurately labeled as fake.
- Defining deepfakes aids participants in identifying fake videos and messages.
- Error management theory correctly predicted that participants would choose the least costly option when considering whether a message or video is fake.
- The perceived danger of a politician is a prominent factor in generating a deepfake label from participants.
Between the lines
With these points in mind, the most salient point comes as the following:
- Messages and videos are considered real when the video subject is perceived as trustworthy.
Despite the potential for deepfake description labels and knowledge of their perceived dangers, knowing that anyone can produce a deepfake, or have a deepfake created about them, is essential. The more trustworthy someone is, the greater the danger of a deepfake involving them has of being seen as authentic.