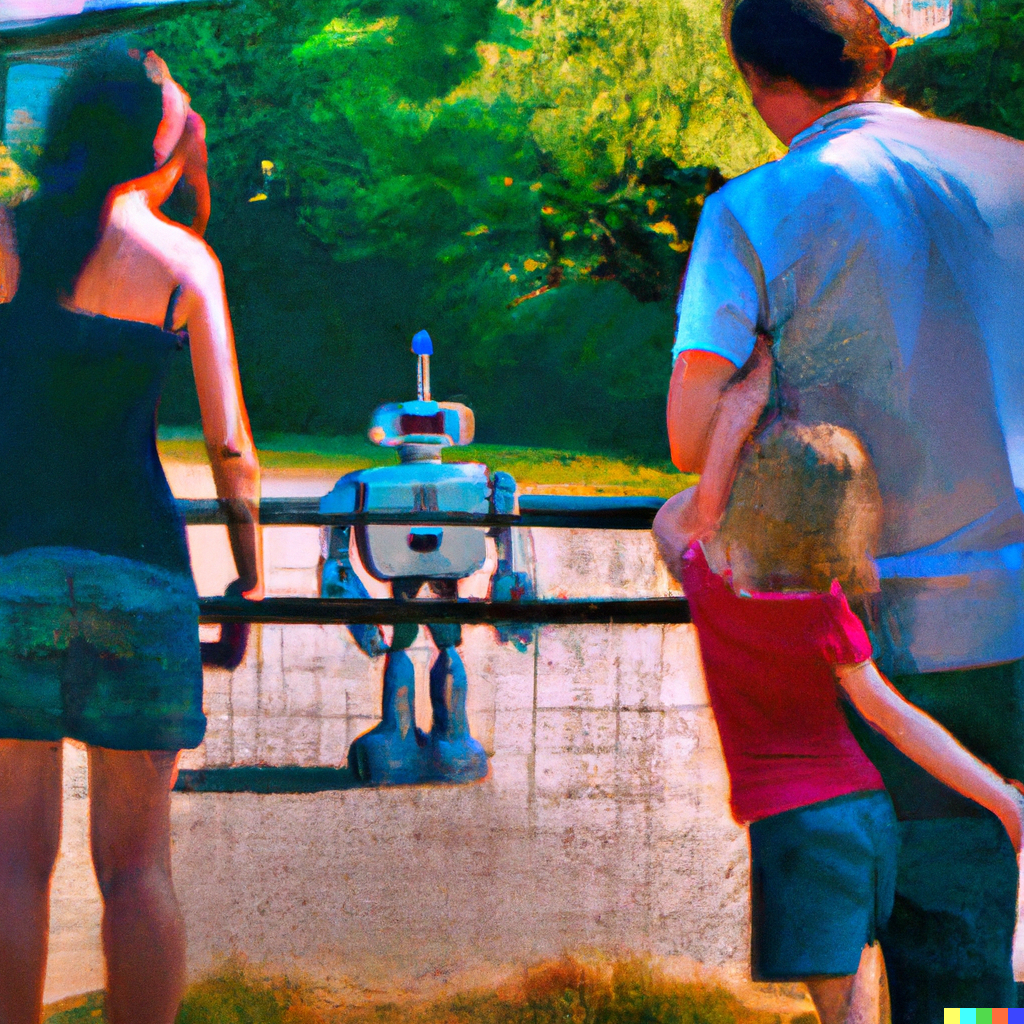
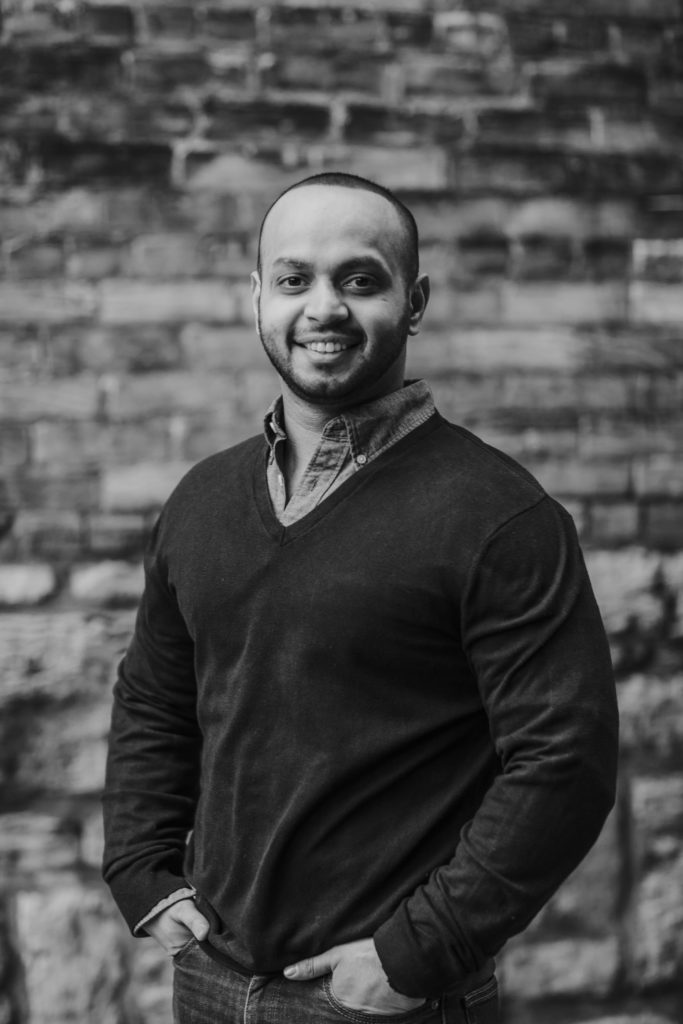
🔬 Research Summary by Devansh Saxena, a Presidential Postdoctoral Fellow at Carnegie Mellon University in the Human-Computer Interaction Institute. He studies sociotechnical practices of decision-making in the public sector and the social impacts of introducing AI in these contexts.
[Original paper by Devansh Saxena and Shion Guha]
Overview: Algorithmic decision-support (ADS) systems are increasingly being adopted by public sector agencies to make high-stakes decisions about citizens as they promise to allocate resources efficiently, remove human bias from the process, and lead to consistent decisions for all cases. We spent two years at a child welfare agency where we observed meetings where critical decisions are made and spoke with caseworkers to gather their perspectives on ADS systems. We learned that the interplay between systemic mechanics (i.e., policies, resource constraints, nuances of labor) and ADS systems can lead to process-oriented harms where they adversely affect the fairness of the decision-making process itself.
Introduction
ADS systems are increasingly utilized in various government sectors, including child welfare, criminal justice, public education, unemployment services, and homeless services. The aim is to enhance public service delivery and allocate resources more effectively to cases in greatest need. These algorithms influence decisions such as which child maltreatment cases to investigate, who should receive preventive services, where additional policing is required, and who is eligible for public housing and unemployment benefits. In child welfare, public scrutiny and media attention have intensified due to removing children from their parents’ care and cases where the system failed to protect children from abuse. This has increased the pressure on child welfare agencies to use algorithmic systems, even though evidence suggests that these systems disproportionately affect low-income and minority communities. Critics have gathered substantial evidence of the harmful consequences of haphazardly deploying ADS systems in the public sector. These algorithmic harms, often disparate in their impact, have led scholars to create a taxonomy of sociotechnical harms and challenge misconceptions about ADS system performance. In this study, we draw attention to harms that are harder to pin down in specific disparate outcomes, policy or design decisions, or the workers’ training but adversely impact the fairness of the decision-making process itself. We unpack process-oriented harms and their impact on professional practices, agency administration, and street-level decision-making in child welfare.
Key Insights
Some Context
In a two-year study at a child welfare agency, we discovered caseworkers heavily relied on algorithmic tools for high-stakes decisions, such as evaluating a child’s mental health needs, selecting foster caregivers, determining foster parent compensation, and assessing sex-trafficking risks. Despite lacking statistical training, caseworkers were legally obligated to use these algorithms. However, the algorithms often disregarded systemic constraints, rendering them impractical and frustrating for social work practice. Notably, the CANS algorithm assessed a child’s mental health needs and risks, influencing foster care placements and compensations. Caseworkers often manipulated CANS scores to boost foster parent pay due to a shortage of quality homes, eroding trust in algorithmic decision-making over time.
Algorithmic Harms to Social Work Practice and Child Welfare Agencies
The child welfare system faces persistent issues of inconsistent decision-making and high caseworker turnover, exacerbated by many caseworkers leaving within their initial two years. Research indicates that it takes about two years for caseworkers to become proficient in their roles, involving interactions with various stakeholders and understanding child welfare practices. Inexperienced caseworkers rely on algorithms like CANS, assuming their objectivity. However, studies show biases can seep into several of these systems through inherently subjective variables such as parents’ cooperation with the agency and stress level without their input or evaluating the effectiveness of the agency’s intervention. Ironically, algorithms contribute to caseworker turnover due to frustrations with such adversarial practices. The discourse on algorithms in the public sector often overlooks the extensive data collection efforts required from workers as a means to improve decision-making. Caseworkers also expressed frustrations with the CANS algorithm, which demands data collection while stripping them of decision-making power. Predictive systems in high-stakes domains are designed to extract discretionary power from workers, replacing it with probabilistic outcomes. Here, human-in-the-loop algorithmic solutions are often proposed, but inexperienced workers are equally likely to make mistakes.
At this agency, serious data provenance concerns exist regarding data collected about children through the CANS algorithm. This data is heavily manipulated by both the caseworkers and foster parents. Initially, CANS was repurposed to calculate foster parent compensations, aiming for fair resource allocation and cost reduction. However, data specialists uncovered cases of algorithm gaming, leading to continually increasing compensations. Additionally, inflated CANS scores send foster children to unnecessary services, straining an underfunded system. This exacerbates barriers to evidence-based decision-making and requires the caseworkers to provide added labor to address the disruption caused to decision-making processes. Caseworkers are obligated to support AI systems but lack control over critical processes. Ethical concerns arise when repurposing algorithms, like CANS, originally designed for mental health assessments, and here, public sector agencies may feel compelled to adopt such tools for innovation and political reasons, further complicating their decision-making ecosystem.
Between the Lines
In this study, we show how functionality issues in ADS systems can lead to process-oriented harms, adversely impacting the nature of professional practice and administration at the agency and leading to inconsistent and unreliable street-level decision-making. We show how caseworkers are forced to assume the added labor resulting from these algorithmic harms and must conduct repair work to address the disruption caused to administrative processes and street-level decision-making. This repair work is conducted within the bounds of organizational pressures, time constraints, and limited resources, making it difficult to properly locate and assess process-oriented harms.