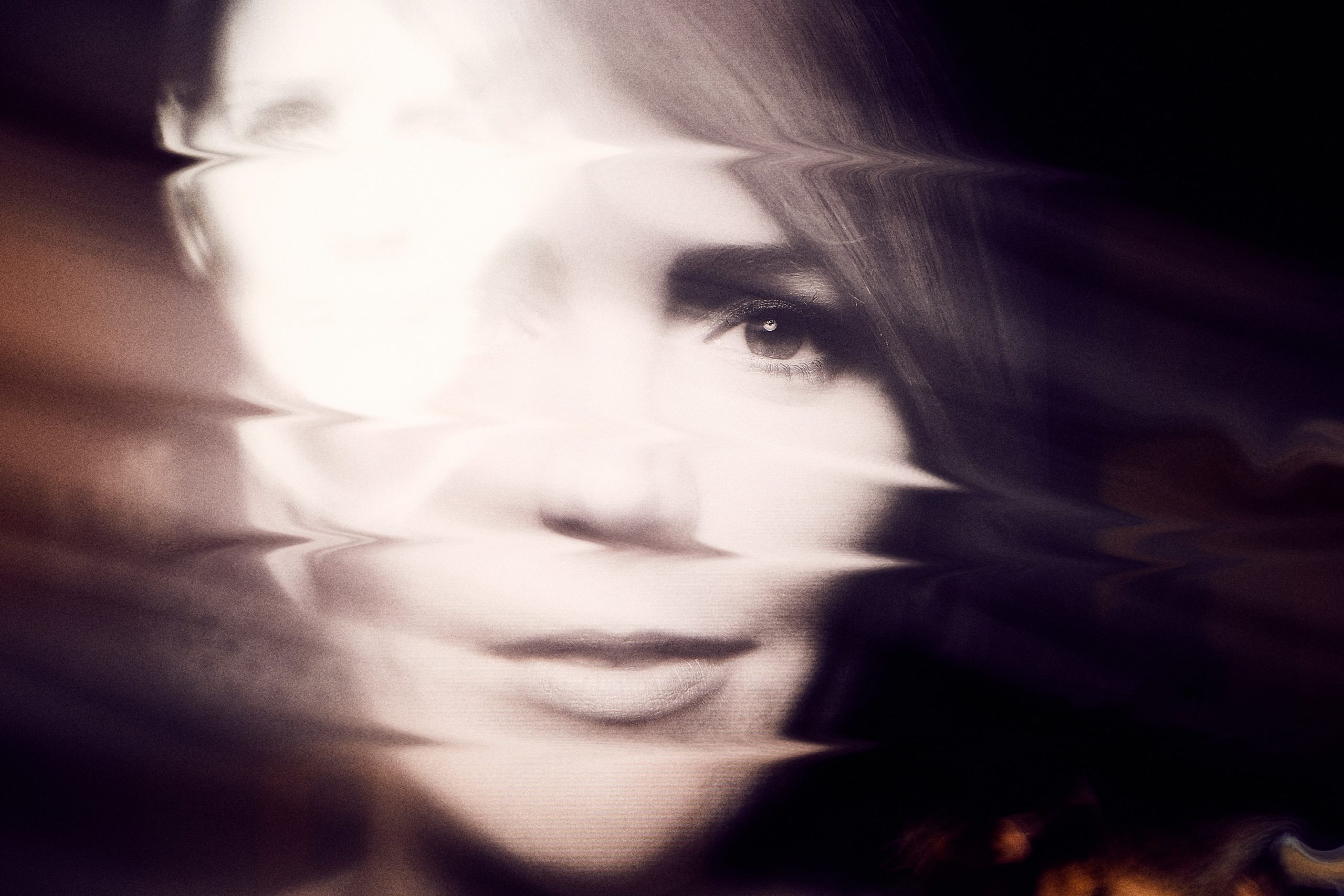
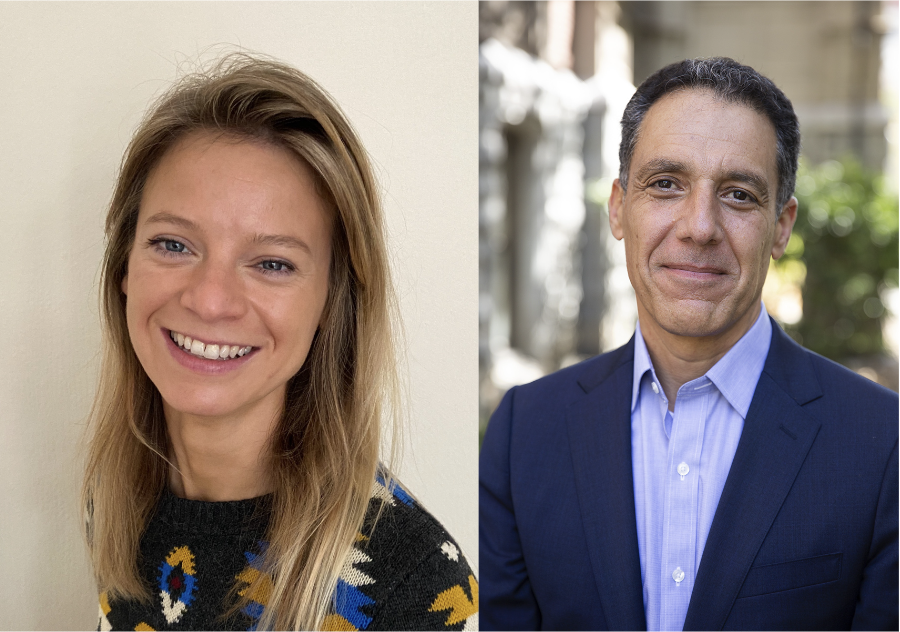
🔬 Research Summary by Sophie J. Nightingale and Hany Farid.
Sophie Nightingale is a Lecturer in Psychology at Lancaster University.
Hany Farid is a Professor in Electrical Engineering & Computer Sciences and the School of Information at the University of California, Berkeley.
[Original paper by Sophie J. Nightingale and Hany Farid]
Overview: Recent advances in machine learning and computational power, paired with the availability of large datasets, have resulted in a new category of fake media—AI-synthesized content (so-called deep fakes). Generative adversarial networks (GAN) are used to synthesize images of people, cats, or landscapes, videos of people saying things they never did, or audio recordings in anyone’s voice. While these advances have led to impressive and entertaining applications, they have also been weaponized in the form of non-consensual sexual imagery, fraud, and disinformation. We will describe our recent research examining the perceptual realism and interpretation of synthetically-generated faces as well as some of the complex ethical issues underlying this type of imagery.
Introduction
In the run up to the 2020 US Presidential election, a 17-year old high school student created a fictional candidate running for Congress complete with a Twitter account and profile picture of a middle-aged white man taken from www.thispersondoesnotexist.com (O’Sullivan, 2020). Twitter verified the account, even issuing it a coveted blue checkmark. This is only one example of how highly-realistic synthetic faces can be misused. We wondered how effective these types of fake profile pictures are in convincing the average user that a real human is behind the account. Starting with 400 synthetically-generated and 400 matched (in terms of age, race, gender, and overall appearance) real faces, we asked 315 paid survey respondents to judge if a face – shown one at a time – is real or synthetic. Participants’ mean accuracy on this task was 48.2% close to chance performance of 50%.
In a second experiment, a new set of 219 respondents received a training session to raise their awareness of common synthesis artifacts, and received image-by-image feedback. Participants’ mean accuracy improved only slightly to 59.0%. In a third experiment, participants were asked to assess the trustworthiness of faces. Much to our surprise, synthetically-generated faces were rated as more trustworthy than real faces.
These results reveal that synthetic images are highly realistic and compelling. This is an impressive technological milestone, but also raises concern for how these images could be used for nefarious purposes.
Key Insights
A GiANt leap
The past few years have seen a rapid rise in the sophistication and realism of synthetic media. One reason for this is the advent of generative adversarial networks (GANs). A GAN works by pitting two neural networks—a generator and a discriminator—against each other. To synthesize an image of, let’s say, a face, the generator starts by splatting down a random array of pixels and passes to the discriminator this first guess. If the discriminator – with access to images of real faces – is able to distinguish the current generated image from a real face, it provides this feedback to the generator. The generator then refines its first image, feeding its second guess back to the discriminator. This process repeats until the discriminator is unable to distinguish the generated face from real faces. You can see for yourself the results of this process by visiting https://www.thispersondoesnotexist.com, where on each page reload you will be presented with a face of a GAN-synthesized face.
The technology is not limited to still images of faces and can be used synthesize and manipulate video and audio. A recent video of Ukrainian President Zelenskyy, for example, purportedly admitting defeat and urging his troops to surrender, was a recent example of a deep-fake video. Although this fairly crude video was quickly debunked, it is almost certainly just a matter of time before synthetically-generated videos join their image-based counterparts and become nearly indistinguishable from reality.
How might deep fakes be weaponized?
Deep fakes are already being weaponized in the form of non-consensual sexual imagery, in which one person’s likeness is inserted into sexually explicit material. Other nefarious uses include small- to large-scale fraud, marketing scams, and additional fuel to disinformation campaigns. In addition to these very real threats, perhaps the largest threat posed by deep fakes will come in the form of the liar’s dividend.
The liar’s dividend observes that when we enter a world where any video, image, or audio recording can be manipulated, then anything can be dismissed as fake. The existence of deep-fakes allows the liar to claim that any inconvenient recording is simply a fake: a video showing soldiers committing human-rights violations, an embarrassing image of a CEO, or an audio recording of a politician admitting to criminal activity – all can, somewhat plausibly, be claimed to be fake.
The inability to reason about basic facts of the world around us poses grave threats to our societies and democracies. This is perhaps the greatest threat posed by deep fakes.
Ethical concerns and considerations
Technologies to automatically synthesize and manipulate digital media are becoming increasingly more sophisticated and accessible. While there are entertaining and inspiring applications to these technologies, it is also clear that these same technologies can be weaponized against individuals, societies, and democracies. As such, we contend that special considerations should be given to the development and deployment of these new technologies, including:
1. Those developing new technologies should consider if the potential benefits outweigh the potential risk.
2. Those deploying new technologies should consider direct mitigation strategies including, for example, the addition of digital watermarks into synthetic media to make downstream identification easier.
3. The broader research community should establish guidelines for how and when these new technologies are developed and how they can be ethically and safely deployed or shared.
4. Beyond the creators of the underlying synthesis technologies, the giants of the technology sector should consider more carefully how their services are being mis-used in terms of the sharing and weaponization of everything from non-consensual sexual imagery to disinformation campaigns.
Between the lines
It is important for everyone from researchers to regulators to understand the applications, limitations, and harms that can arise from new AI-based technologies. Our investigation has only considered the photo-realism of still images. Techniques for synthesizing videos, audios, and even text continue to advance in quality and usability, raising additional concerns as to how this type of synthetic content may be misused.
Now that we understand the perceptual limitations of distinguishing real from fake images, we must turn our efforts to developing computational techniques for performing this important task. Along with these technologies, we must also consider reasonable policies and regulation that allow for an open and free – but also trusted and safe – online information ecosystem.