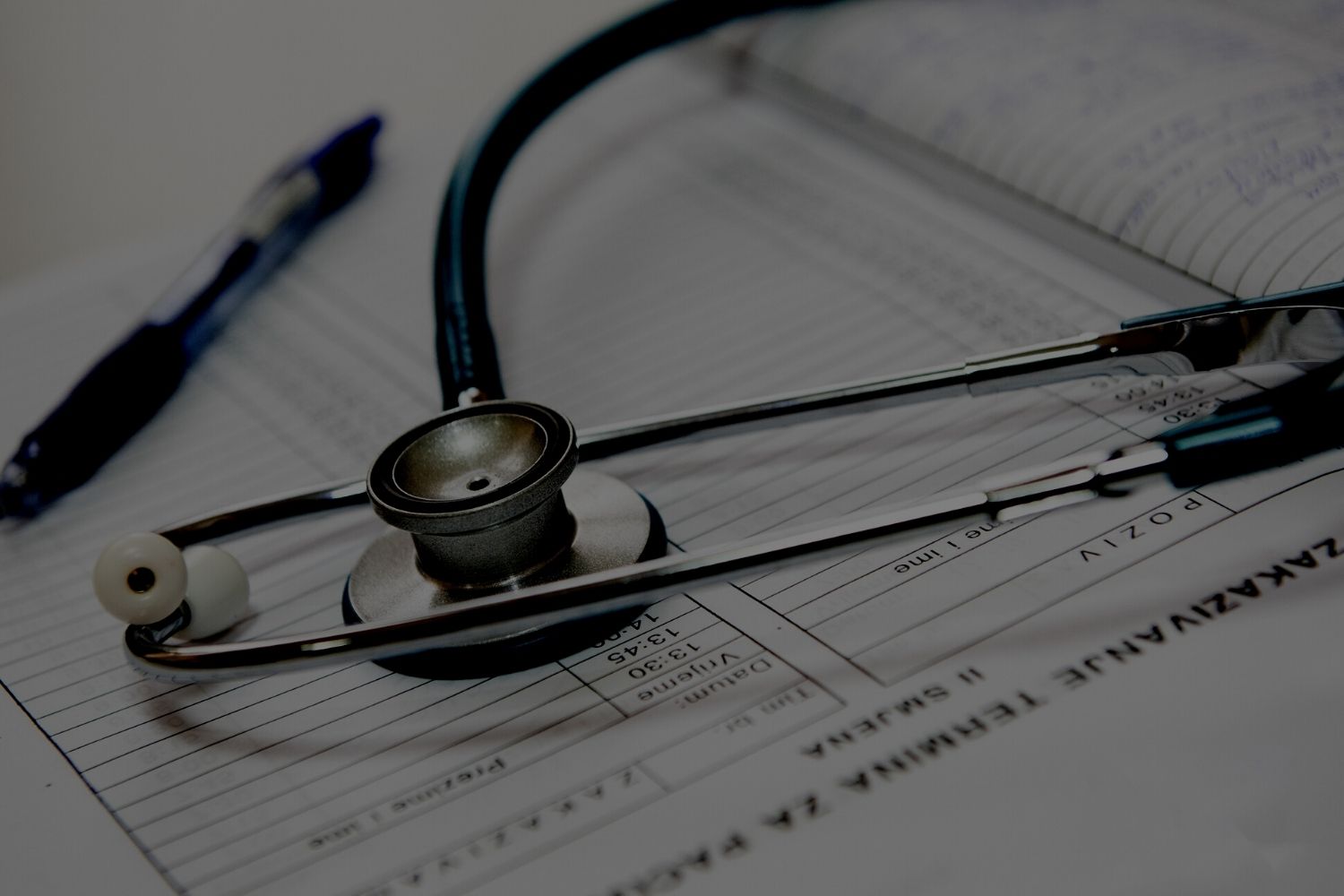
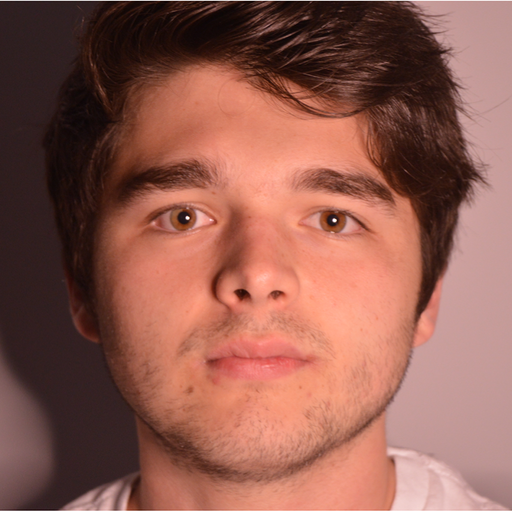
Written by Connor Wright, research intern at FairlyAI — a software-as-a-service AI audit platform which provides AI quality assurance for automated decision making systems with focus on AI equality.
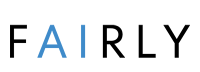
One of Artificial Intelligence’s (AI’s) main attractions from the very beginning was its potential to revolutionise healthcare for the better. However, while taking steps towards this goal, the implementation of AI in healthcare is not without its challenges. In this discussion, I delineate the current situation surrounding the use of AI in healthcare and the efforts by regulatory bodies such as the FDA to mitigate this emerging field.
I explore how this potential regulation may send the wrong signal to manufacturers (and best practices on how to make this process easier) and how while there have been AI-powered healthcare systems approved, this is by no means the beginning of a mass overhaul of the medical environment. I nevertheless maintain the positivity of how these approved applications are augmentative in nature and aren’t out to replace human medical practitioners. There are new signals being sent out through the arrival of AI in healthcare, but they are not to be frightened of.
Artificial Intelligence (AI) knows no bounds when it comes to being applied in different areas, and healthcare is no exception. One of AI’s biggest pulls is how it has the potential to revolutionise the healthcare landscape, stretching from helping doctors to better identify signs of cancer in patients as well as better analysing X-ray images. In this post, I aim to bring to light some of the most recent developments in the AI healthcare space to consider, which includes what risks AI poses, legislation, and how the current market signals affected by the legislation are being forced to send the wrong message to manufacturers. AI in the healthcare space will from now on be referred to as Software as a Medical Device (SaMD), which includes any software (a system not supported by hardware) that is meant to influence the diagnostic, treating or curing of a disease whether by informing the doctor’s opinion or returning a classification of its own. Interestingly, what I will not discuss are devices that are intended for the wellbeing of a human (such as fitness trackers) due to them being discounted as a SaMD under the 21st Century Cures Act.
SaMDs can be classified in two different ways, one of which I will discuss now. Here, SaMDs can be termed as “locked”, whereby it applies solely a fixed function to a problem (such as following a decision tree). In this case, once approved for market release by the governing bodies such as the FDA (through their 510(k) submission), an updated application for market approval for the algorithm does not need to occur. As a result, SaMD-led medical applications have been approved to market by the FDA, two of which I will detail now.
In 2018 the FDA approved software that was to detect above mild level appearances of the disease diabetic retinopathy in adults who are diabetic (to be found here).As the leading cause of blindness amongst those diabetic, early detection is pivotal for doctors especially when the patients don’t see their doctors on a yearly basis. Here, the IDx-DR software managed to achieve 87.4% accuracy with patients who had more than a mild case of the condition, and 89.4% for those who had less than a mild level. Likewise, Imagen OsteoDetect was approved by the FDA to detect distal radius wrist fractures in adult patients from X-ray images (to be found here). Practitioners found that their results improved with the use of the technology, increasing the likelihood of detecting such specific fractures. However, both these cases show where some of the main takeaways from current SaMDs lies.
Despite achieving the feat of being approved to market, both systems are incredibly specific on what they can be used for. Both these SaMDs carry a narrow application with very specific criteria to identify. Furthermore, both these systems are “locked”, and thus were easier to approve as they won’t change how they make decisions or produce outputs. This is where I can now introduce “adaptive” algorithms.
As you may very well know, the beauty of machine learning is that it can adapt as it learns over time and in terms of what inputs it receives. Hence, its outputs can change (both for the better and for the worse) depending on where it’s applied. This now proves a real regulatory conundrum. At this point in time, discussions are being had on how to approach adaptive algorithms, ranging from constant updates being supplied to the FDA on how it is evolving, to multiple 510(k) submissions being required. Furthermore, greater transparency is being implemented as a requirement by the FDA, whereby any 510(k) application must detail all the relevant stakeholders (doctors, patients, manufacturers, the potentially affected public etc.).
Currently, both the European Union and the FDA are working off different classification systems (from I being low risk to III being the highest risk in both cases) whereby the higher the risk classification, the more regulatory measures need to be met. This system aims to try and reduce the likelihood of requiring such high regulatory requirements, with the FDA in particular wanting to leave lower-level risk items without too much bother. Likewise, in a discussion group hosted by Deloitte (to be found here), the group agreed that manufacturers’ SaMDs being classed as low risk (like hearing aids) are to be practically untouched by regulation, while manufactures of higher risk SaMDs (like insulin dispensers) are to be liable to more data requirements and more stringent regulation. As a result, this could potentially be sending the wrong message to the manufacturers involved, which I shall now explain.
Instead of adopting the more innovative and potentially more rewarding adaptive algorithm development, manufacturers could now maybe start leaning towards solely manufacturing locked algorithms to save themselves the regulatory hassle. As a result, the FDA have devised some machine learning best practices to try and avoid this tendency. For example, there is to be an appropriate separation between training, tuning, and test datasets rather than all being combined into one. Furthermore, the data being collected by the SaMD must be relevant to the clinical purpose it strives to achieve. Amongst other best practices, these two pieces of advice can provide good food for thought when looking towards the future of what the entrance of SaMDs in healthcare means for the discipline.
One thing to bear in mind for manufacturers wanting to implement a SaMD is how the device may perform as expected in training, but may misbehave when employed in different populations. Hence, the advice of having a diverse data set is still as important as ever to help prevent this. Furthermore, the current SaMDs that are being approved are very narrowly focused and with good reason. Trying to produce wider-ranging SaMDs will prove quite the challenge, but this should still possess the augmentative aspect that we have seen in this discussion, and should not aim to replace the practitioners entirely. Hence, while AI in healthcare is making new waves, there still remains a decent amount of time before we’ll be able to surf them.
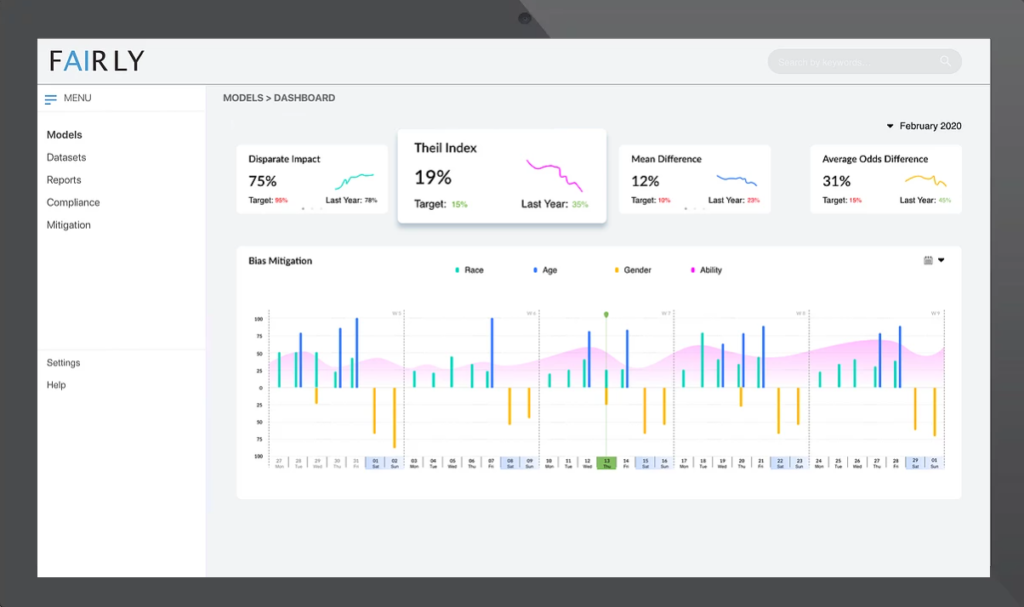
Fairly’s mission is to provide quality assurance for automated decision making systems. Their flagship product focuses on providing an easy-to-use tool that researchers, startups and enterprises can use to compliment their existing AI solutions regardless whether they were developed in-house or with third party systems. Learn more at fairly.ai.