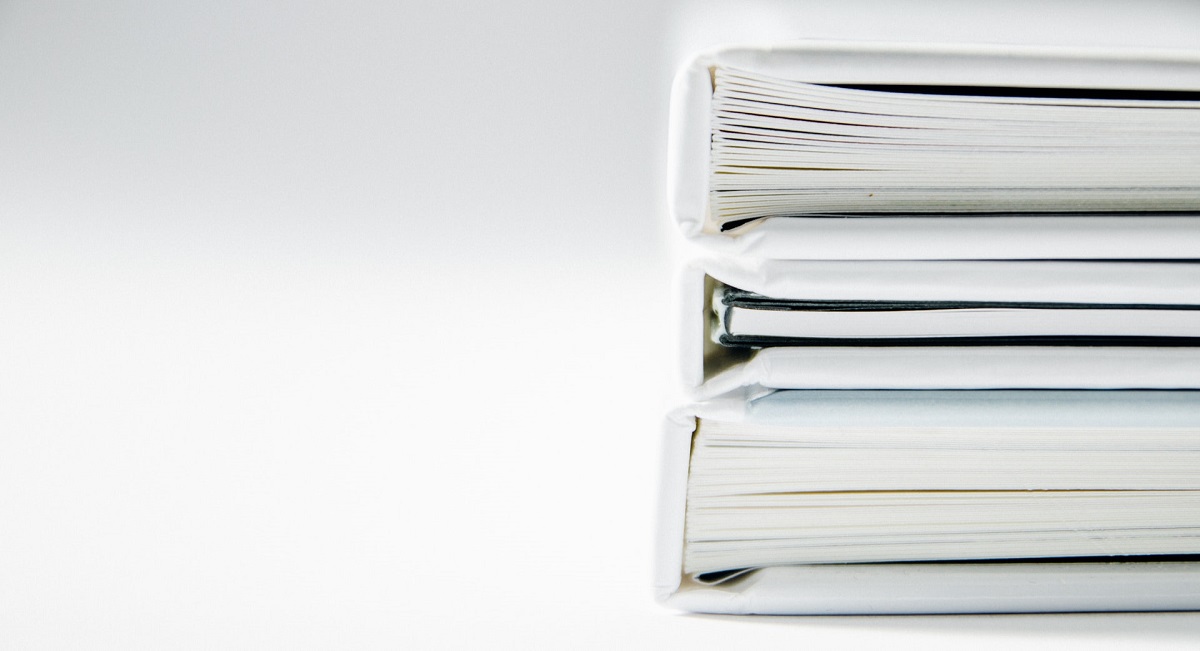
š¬ Research summary by the authors of the original paper: Daniel Schiff (@Dan_Schiff), Jason Borenstein, Justin Biddle (@justinbbiddle), and Kelly Laas.
Overview: While recent studies suggest that a global consensus about AI ethics is emerging, this paper finds meaningful differences across the public, private, and NGO sectors. Our team evaluated 112 AI ethics documents from 25 countries, scoring the presence or absence of 25 ethical concepts, the documentsā level of engagement with law and regulation, and how participatory the authorship process was. Overall, NGO documents reflect more ethical breadth, public sector documents prioritize economic growth and unemployment, and private sector documents emphasize client-facing issues and technical fixes.
Introduction
Is there global agreement on which ethical issues surrounding AI are most important and how these issues should be understood?
Itās an important question. How developers, policymakers, publics, and other key actors who are shaping the future of AI think about ethics determines which issues leading decision-makers emphasizeāand which issues they donāt. Agreement across different stakeholders could facilitate responsible innovation and better domestic and international coordination. Disagreements could lead to conflict over the best course for AI research, development, and policy. Yet agreement may be superficial or even misplaced, while conflict can be important and productive. Understanding consensus and dissensus is thus critical and some of the best research to date suggests a global consensus is emerging around 5-10 AI ethics issues deemed most important, such as bias, transparency, and privacy.
We put the question to the test, looking specifically at differences across public, private, and non-governmental approaches to AI ethics. To do so, pairs of two coders from a team of four researchers scored the importance of 25 unique ethical concepts across 112 AI ethics codes, principles, frameworks, and policy strategies, resulting in a new open-access dataset, the AI Ethics Global Document Collection.
We find that private-sector documents reflect less ethical breadth, are less participatory in their creation, and are less engaged with law and regulation. Public sector documents are disproportionally focused on economic growth and employment concerns. NGO sector documents reflect the most ethical breadth overall, addressing issues like cultural sensitivity and human-human interaction that are often neglected. Finally, there are other topics, like the psychological impacts of AI, that are rarely covered in AI ethics documents from any sector, a finding that may warrant further attention.
These differences may result from how organizations frame their own responsibilities, how they interpret and trade off different ethical concepts or goals, and whether they rely on individuals internal to their organization or more diverse voices. Yet regardless of the cause, understanding differences in ethical priorities and interpretations is important to shaping the future of AI ethics practice and policy positively.
A Summer for AI Ethics Principles
Those interested in AI ethics know that the last five years have seen a frenzy of action, as companies, governments, civil society organizations, and academics offered up their own vision of AI ethics. A wide variety of documentsāAI ethics codes, guidelines, principles, frameworks, and even policy strategiesā seek to define the problems and (sometimes) propose solutions to address AIās social and ethical implications.
Despite optimism that this newfound attention holds promise for the future of responsible and ethical innovation and policymaking, some have cautioned that AI ethics principles are currently ātoothless.ā According to that view, AI ethics may be trapped in ethics-washing and window-dressing, perhaps serving as a strategy by the private sector to avoid costly regulation. As others have also argued, we suggest that what is needed is to translate the principles to practices and to ask deeper questions about the socio-technical possibilities for ethical AI beyond common ideas of algorithmic fairness and transparency.
In our related research, we considered how to measure the efficacy of AI ethics documents, what kinds of impacts they might have, and what motivations characterize their creation. We argued that motivations should not be evaluated in binary terms but are rather a complex combination of efforts to strategically inform internal and external changes, genuinely drive responsible outcomes or further competitive goals, or even signal oneās own leadership. Most importantly, we believe the discourse around AI ethics is worthy of study, including because it drives critique. Ethics principles are playing a fundamental role, for example, in how EU and US regulation is developing, and in how companies are devising impact assessments.
It is important to consider then, do members of the public, governments, different cultures and regions of the world, large and small companies, and so on, agree about what AI ethics means and what is important to prioritize?
Measuring AI Ethics in the Public, Private, and NGO Sectors
Our study sought to tackle part of this question, namely: Is there agreement or disagreement across various AI ethics documents produced by organizations in the public (e.g., governments), private (i.e., companies), and NGO (e.g., civil society, academic) sectors?
To help answer this question, pairs of members of our team of four (a policy scholar, two philosophers, and an information scientist) manually coded over 4000 pages of AI ethics documents published between 2016 and the middle of 2019. Our final sample of 112 English-language documents (including official and high-quality unofficial translations) comes from 25 countries. While prior research in this field has sought to reduce the complexity of AI ethics, for example, by arguing the discourse can be understood according to 5-10 ethical concepts, our work embraced the complexity by analyzing 25 distinct ethical topics ranging from concepts such as accountability and inequality to privacy and workforce diversity. Two coders scored each document and topic as either absent (0) or as a minor (1) or major (2) topic, allowing us to quantify the presence, relative priority, and overall ethical breadth and depth of ethics topics across documents and organizational sectors.
Consensus, Omission, and Disagreement in AI Ethics
This fine-grained approach confirms that some topics are shared priorities across sectors, including transparency, fairness, privacy, and safety. Notably, there is also a consensus in which topics are neglected or omitted, such as concerns about existential risk and cultural sensitivity. These omissions are worthy of attention and highlight the fact that paying attention to consensus alone can mask important dimensions of AI ethics.
We found that certain topics are ranked and prioritized differently across sectors. For example, public sector documents are disproportionately focused on issues related to economic growth and unemployment, while the private sector pays little attention to these issues. This may indicate that governments are disproportionally focused on innovation and economic issues, potentially to the exclusion of other important topics. Those concerned about how the financial incentives of the private sector influence AI ethics may thus also find it important to think more about the public sector, especially in light of forthcoming regulation.
Our results indicate that the NGO sector, as defined, has the broadest and most inclusive treatment of AI ethics. While private and public organizations pay little attention to how AI can lead to psychological impacts or influence human-human relationships, NGOs do raise these issues. NGOs also emphasize accountability, suggestive of their role as an external watchdog, attempting to influence the other sectors. Another key finding is that private-sector documents reflect the least ethical breadth overall, with greater attention to areas where there are ostensible technical āfixesā to AI ethics issues, like privacy and explainability.
Participation and Engagement with Law
We also coded documents for the degree to which there was diverse and public participation in the creation of documents, for example, through public hearings, workshops, and multiple authors. Public and NGO documents are far more participatory in terms of author representation. Approximately three-quarters of public sector documents are produced through an āopenā or āsemi-openā process according to our coding, whereas less than a quarter of private-sector documents are open or semi-open. Similarly, we coded documents for their level of engagement with issues of law and regulation, which may suggest whether organizations have considered how to implement formal regulation in practice, versus favoring self-regulatory strategies. Private sector documents are again less engaged with formal regulation and law.
Learning from Sectoral Differences
What can we learn from these differences?
To begin an answer, our paper draws on the notion of āethical frames,ā defined as a set of grounding assumptions about ethical problems and solutions. One explanation for our findings is that organizations may think that they are only responsible for a subset of issuesāsuch as private companies wishing to avoid harms to customers, or governments aiming to maximize the economic growth of a country. Organizations may also be pulled between pro-social and competitive goals and may make assumptions about who is worthy to define the problems and solutions surrounding AI ethics in the first place.
In our view, these beliefs, assumptions, and the ethical frames they constitute are worthy of examination. For example, the roles and responsibilities of organizations can be questioned, expanded, and informed by the collective need to shape AI ethically. It may be important to seek more voices and challenge our notions of what is desirable and indeed possible in AI ethics practice and policy. Further, whether ethics and innovation are necessarily in tension with one another is not a settled matter. In sum, we suggest the trajectory of AI ethics need not be locked in stoneāmore possibilities can be revealed by challenging the terms of the debate itself.
Between the lines
There is much still to understand. Our analysis looks only at AI ethics documents, not the broader body of work done by organizations, nor at differences across regions, for example. Further, there are many ways to aggregate, interpret, and define AI ethics concepts, and these subtleties can have important consequences, as our study shows. Going forward, much more needs to be understood about the kinds of impacts AI ethics documents and the principles articulated in them are having. Yet despite and perhaps even because of contention and criticism surrounding AI ethics principles, the associated discourse matters and will continue to matter as the AI community sets a course for action.