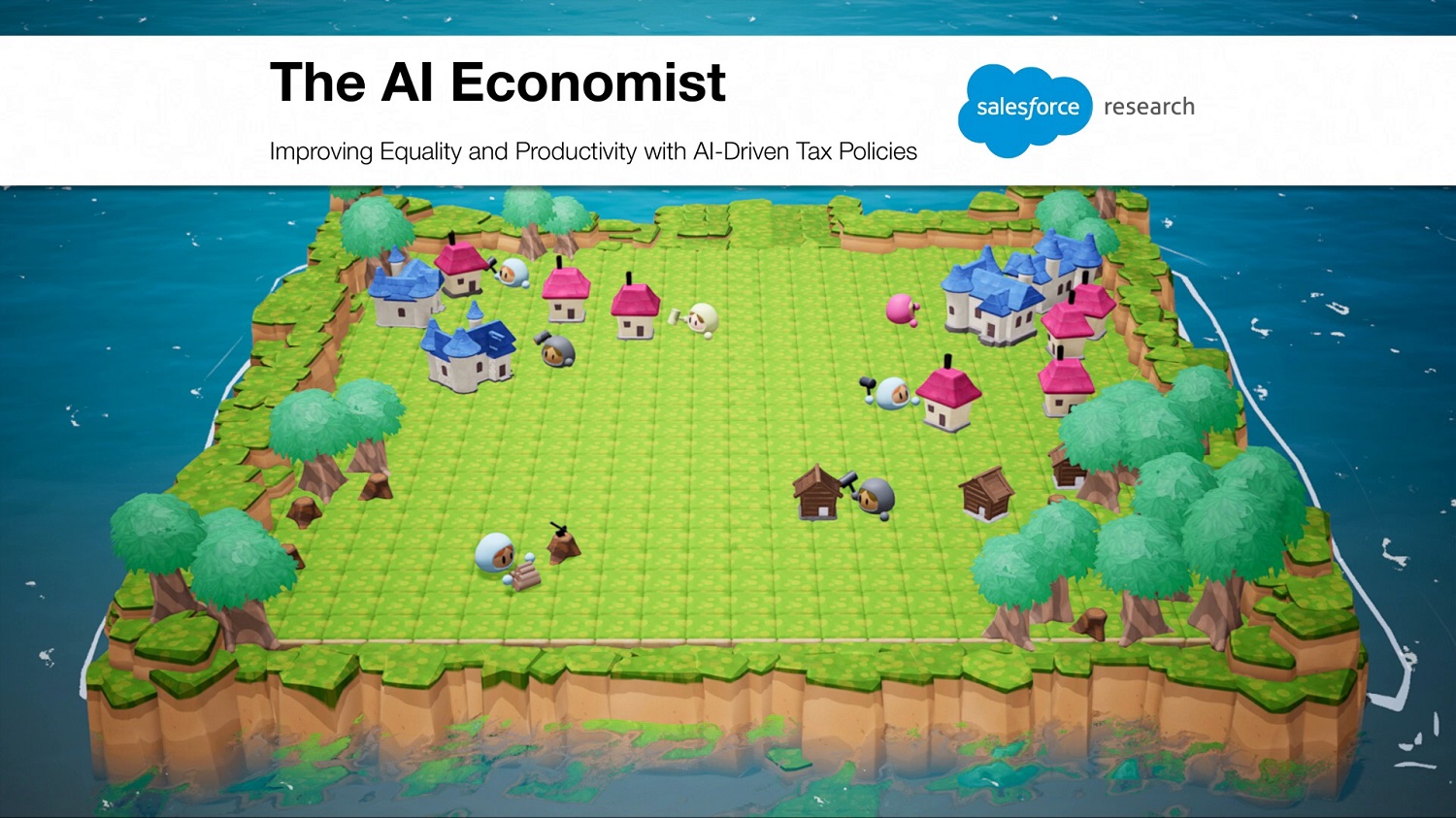
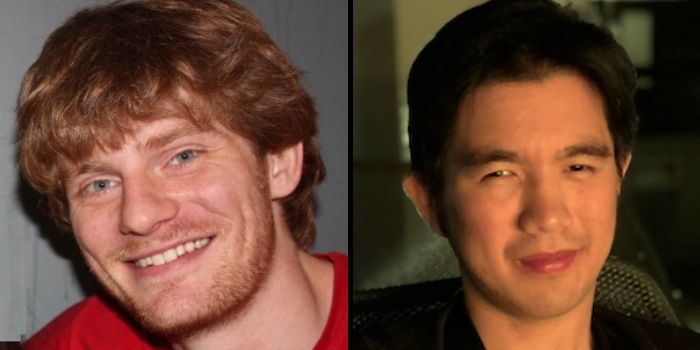
Long before pandemic-related lockdowns, economic inequality has been one of the most significant issues affecting humanity. A report from the United Nations in January 2020 found that inequality is rising in most of the developed world. With so much to lose or gain, itâs no surprise that bias can influence policymaking… often to the detriment those who need the most help. This underscores the potential that AI can have for good, and why itâs important to develop tools and solutions that are simulation- and data-driven to yield more equitable policies.
The new AI Economist model from Salesforce Research is designed to address this kind of equality by identifying an optimal tax policy. By using a two-level reinforcement learning (RL) framework, training both (1) AI agents and (2) tax policies, it simulates and helps identify dynamic tax policies that best accomplish a given objective. This RL framework is model-free in that it uses zero prior world knowledge or modeling assumptions, and learns from observable data alone.
Our ultimate goal for the AI Economist is for it to be a stepping stone for future economic AI models that support real-world policy-making to improve social welfare. We believe a RL framework is well-suited for uncovering insights on how the behavior of economic agents could be influenced by pulling different policy âlevers.â
The initial results are incredibly promising and prove that itâs possible to layer a simulation and data-driven approach to optimize for any social objective to more quickly create equitable, and effective, tax policies. The AI Economist model achieved results that were at least 16% better than the baseline models used in the study.
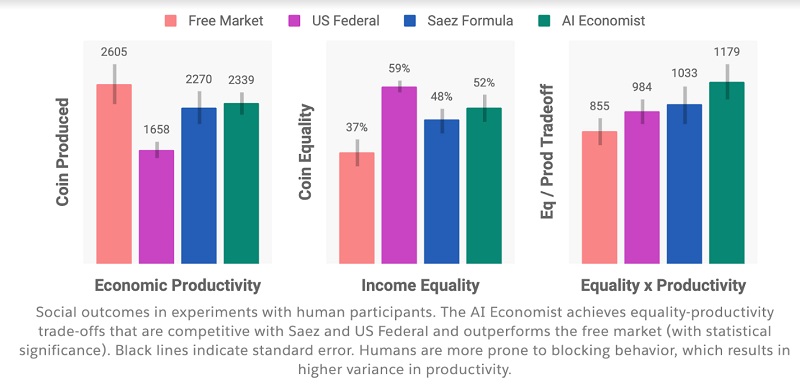
When we set out to build the AI Economist, we did so while recognizing the negative impact that inequality has on economic opportunity, health and social welfare. Economics includes numerous variables and considerations driving outcomes, but the AI Economist turns its focus to taxes. Why? Taxes are an important tool that governments use to redistribute wealth and resources â helping to address inequality. It is the first time anyone has used RL to directly learn AI tax policies in AI simulations.
A key difference between the AI Economistâs application of RL and most others is the avoidance of a âzero-sumâ outcome… itâs not trying to beat anyone. âVictoryâ is achieved when the outcome creates an optimal balance between equality and productivity.
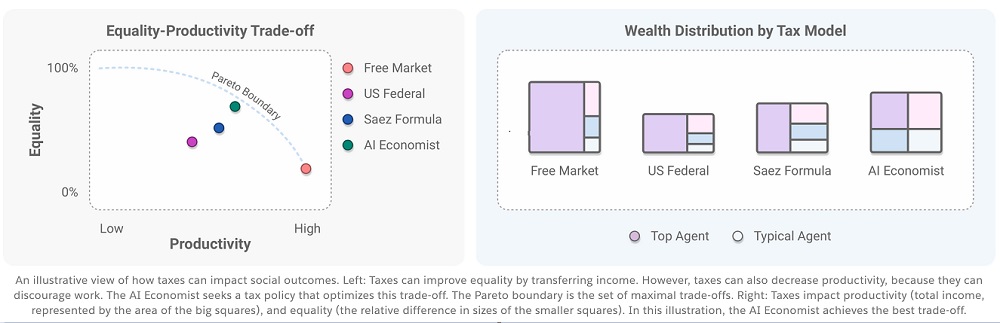
The simulation itself manifests into a two-dimensional world that is based on classic tax theory â modeling people who earn income by performing labor. A worker gains utility from income and incurs the cost of the labor labor effort. The simulated world features two types of resources: wood and stone. AI agents (or workers) gather and trade each resource, as well as earn income by building houses.
Each simulation also reflects different skill levels among workers. Higher-skilled workers earn more income, resulting in more utility. But the amount of effort it takes to build them reduces its utility. As learning progresses, workers develop more concrete roles based on their skill level and how each worker learned to balance income vs. effort.
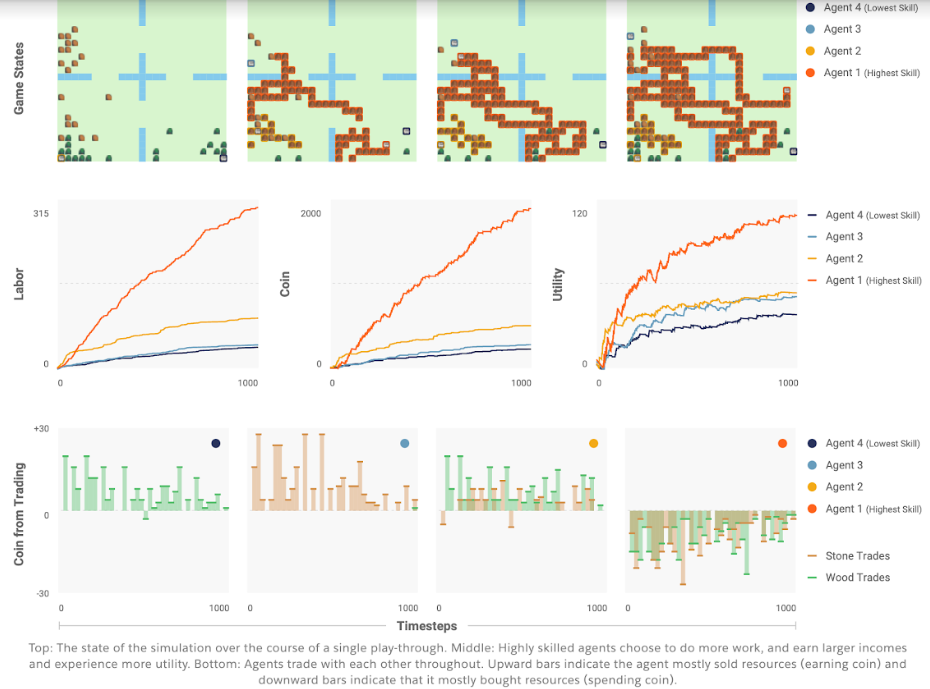
Results of the AI Economist simulations were compared with three different scenarios:
Every scenario included workers that face seven income brackets, corresponding to existing U.S. federal tax brackets. Mimicking human behavior, AI agents found ways to game the system, particularly with regressive tax models, by lowering their taxes by alternating between high and low incomes rather than sustaining one or the other.
- A free market with no taxes or efforts to redistribute via taxes
- A progressive tax that increase with income, similar to the 2018 U.S. federal tax schedule
- An analytical formula proposed by Emmanuel Saez that, in our specific simulation, establishes tax rates that decrease with income
Following millions of simulations, our RL approach produced effective dynamic tax policies that resulted in a better balance of equality and productivity than the other methods tested. The AI Economist achieved, at minimum, a 16% better trade-off between equality and productivity compared to the Saez model, the next best performing scenario. Compared to a free market, the AI Economist generated a 47% advantage in equality while only sacrificing 11% in productivity.
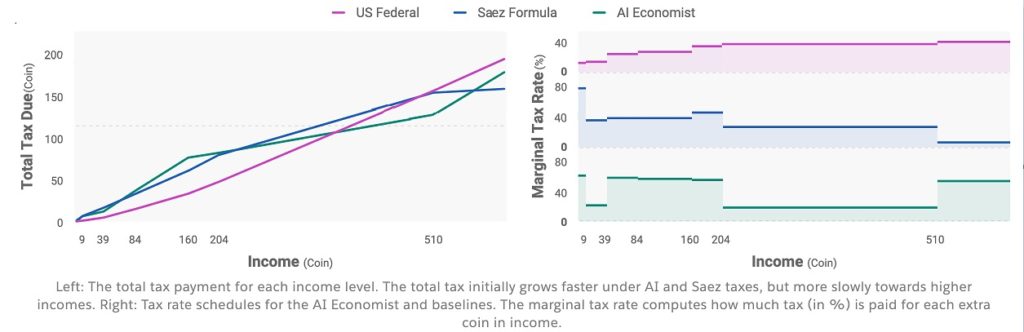
The AI Economist also proved to be more effective in accounting for and addressing aforementioned agents looking to game the system. This is especially significant because in reality, the standards for equality fluctuate over time. Modelers donât always have the luxury of having historical data to use. The ability to adapt quickly to current socioeconomic trends is crucial to sustaining policy effectiveness in the real world, and simulation-based systems can better test what-if scenarios while mitigating the potential of real side-effects.
Weâve already started testing the impact of the AI Economist with human participants. Although experiments with real people used a simpler ruleset, the AI Economist yielded similar performance improvements as the simulations with only AI agents.
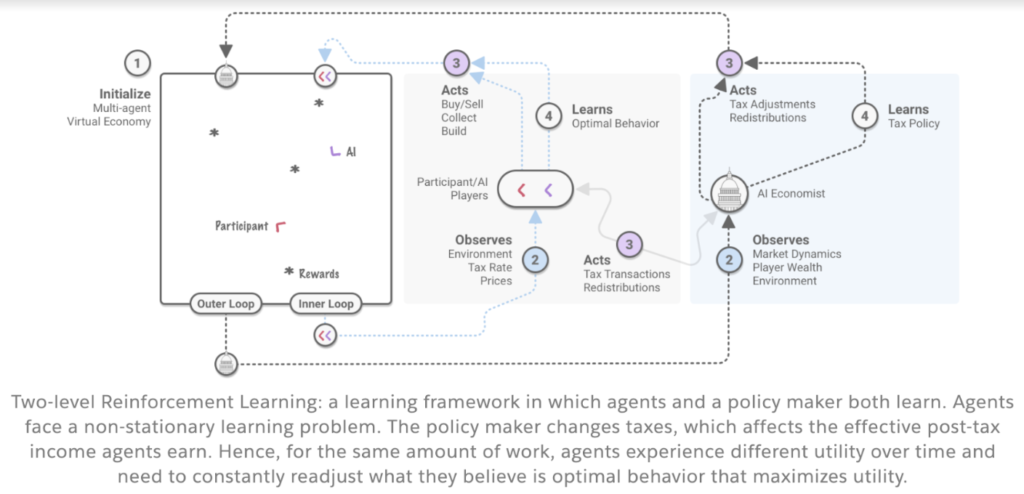
While the simulations represent a small economy for the time being, we feel it is huge step toward building a viable tool with advanced modeling capabilities for economists and governments to test and create effective policies. This iteration of the AI Economist is in some ways already more complex than other models used by economists today. As models evolve and RL grows more sophisticated, future simulations can include âsmarterâ agents and increase the scope of each simulation to account for more complex economic processes and variables.
We invite further discussion about the AI Economist from all communities, and would love to hear your input. Please reach out to us on Twitter at @RichardSocher and @StephanZheng.
Original Paper by Stephan Zheng, Alex Trott, Sunil Srinivasa, Nikhil Naik, Melvin Gruesbeck, Kathy Baxter, David Parkes and Richard Socher
Full paper: https://arxiv.org/abs/2004.13332