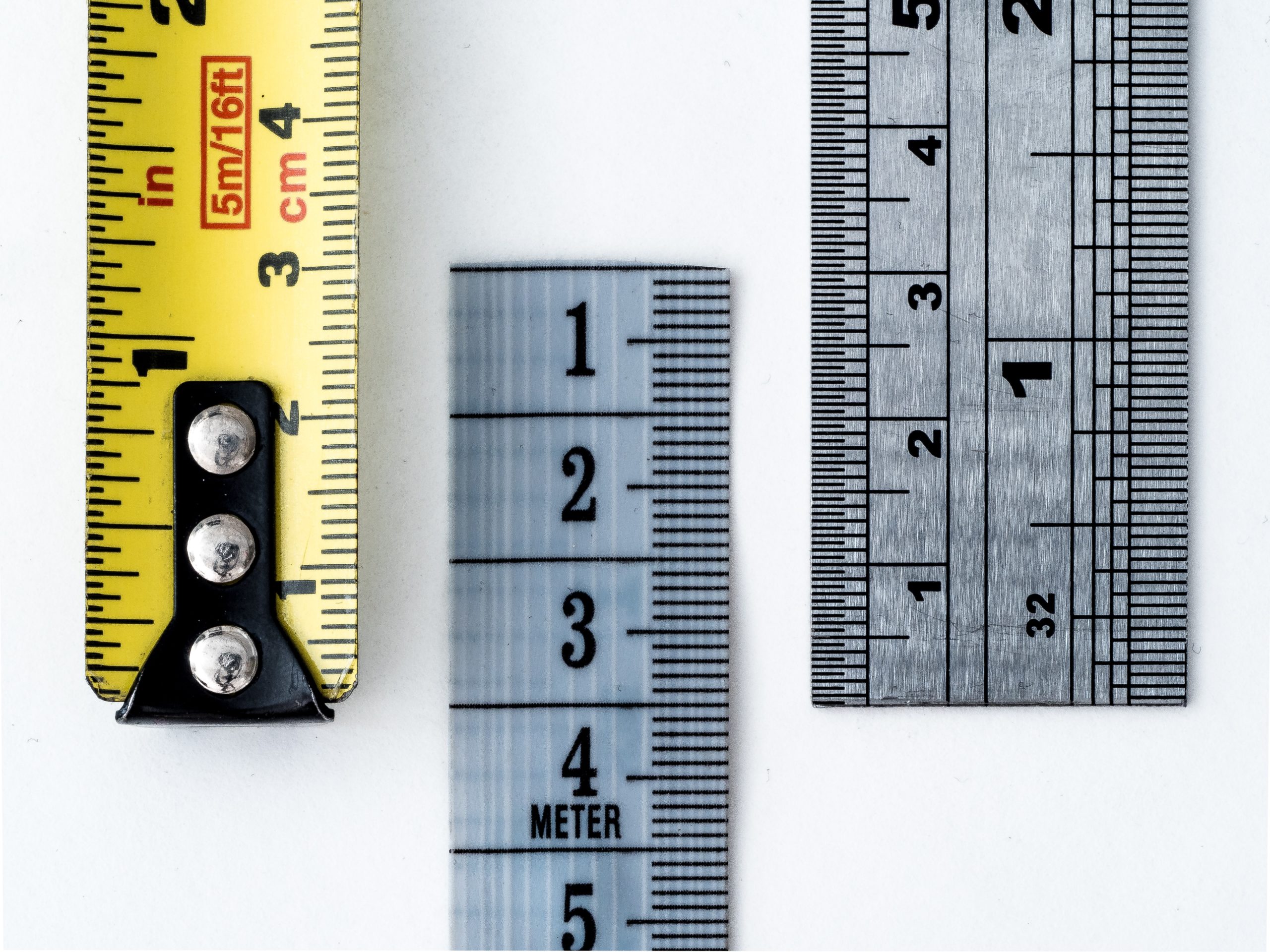
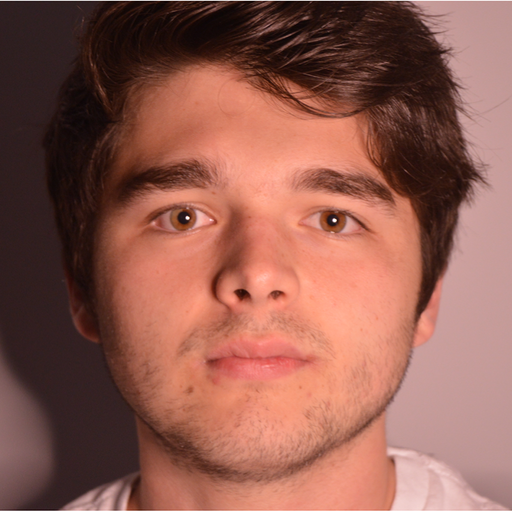
🔬 Research summary by Connor Wright, our Partnerships Manager.
[Original paper by Reva Schwartz, Leann Down, Adam Jonas, Elham Tabassi]
Overview: What does bias in an AI system look like? Is it obvious? How can we mitigate such threats? The NIST provides a 3-stage framework for mitigating bias in AI, with it being seen as key to building public confidence in the technology. Not only can such mitigation help us better reduce the effects of AI, but it can also help us better understand it, and the NIST wants to do just that.
Introduction
What does bias in an AI system look like? If we saw it, would we be able to mitigate it? The National Institute of Standards and Technology (NIST) tries to answer both of those questions as part of their pursuit for a framework for responsible and trustworthy AI. Mitigation, transparency, and public engagement are widely accepted as popular notions for building public trust in AI. For me, the most exciting points in the NIST’s draft are their interaction with bias as a concept and their 3-stage framework. With bias proving one of AI’s biggest problems, such frameworks can better expose this problem and better understand it.
Key Insights
The problem of bias
It’s important to note how automated biases can spread more quickly and affect a wider audience than human biases on their own. Rather than being confined to those you interact with, the presence of AI systems that stretch across the globe means that those affected by its negative consequences are more numerous. Its effects are then heightened through AI’s presence (and further potential presence) in our lives. For example, the proliferation of facial recognition technology and AI being used in job screening. As a result, the NIST finds it necessary to investigate how this can come about, and I wholeheartedly agree.
Why is this the case?
Bias can be seen to creep in when the object of study can only be partially captured by the data, such as a job application. Here, aspects such as the value gained from work experience and how it translates into the new role cannot be accounted for by just a simple keyword search.
At times, bias also enters into the fray through AI decisions being made using accessible rather than suitable data. Here, researchers are said to “go where the data is” and formulate their questions once they get there, rather than taking complete account of the necessary data for an informed and representative AI system. For example, it would be as if you were to look at a college application and solely focus on the academic data (grades) available, rather than also looking at the extra-curricular activities the candidate has undertaken.
To try and tackle this, the NIST proposes a 3-stage lifecycle to better locate how AI can enter the picture.
Stage 1: Pre-design
Here, the technology is “devised, defined and elaborated, “ which includesto involve then framing the problem, the research, and the data procurement. Essential notions to consider can then be seen in identifying who’s responsible for making the decisions and how much control they have over the decision-making process. This allows for a more evident tracking of responsibility in the AI’s development and exposes the presence of any “fire, ready, aim” strategies. What is meant by this play on words is how, at times, AI systems are often deployed before they’ve been adequately tested and scrutinised. The second stage then becomes even more relevant.
Stage 2: Design and development
Usually involving data scientists, engineers and the like, this stage consists in the engineering, modelling and evaluation of the AI system. Here, the context in which the AI will be deployed must be taken into account. Simply deploying an accurate model does not automatically mitigate any problem of bias without this essential component. This is to say, a facial recognition system could be 95% accurate in identifying the faces of children between 5-11 years old, but being deployed in an adult context will render it useless.
In this sense, techniques such as “cultural effective challenge” can be pursued. This is a technique for creating an environment where technology developers can actively participate in questioning the AI process. This better translates the social context into the design process by involving more people and can prevent issues associated with “target leakage”. To explain, “target leakage” is where the AI trains on data that prepares it for an alternative job than the one it initially intended to complete. To illustrate, training on past judicial data and learning the decision-making pattern of the judges and not the reasons for conviction. If such problems can then be avoided, the deployment stage will be less likely to run into any issues. However, this is not always the case.
Stage 3: Deployment
The deployment stage is probably the most likely stage for any harmful bias to emerge, especially given how the public now starts to interact with the technology. Given AI’s accessibility, such interaction can also include malicious use on behalf of an unintended audience, such as using chatbot technology to spread fake news online. Even if this wasn’t intentional, the general interaction by the public could also expose any problems to do with the technology.
This shouldn’t be the case, however. Any such problems should instead be dealt with in the 2 previous stages, but the current AI ecosystem is geared towards treating the deployment phase as the testing phase. While this continues to be the case, the response to AI bias will not be mitigation but rather a delayed reaction.
Between the lines
For me, generating this kind of framework is definitely the right way to go. Having defined stages of the AI lifecycle can make the identification of responsible parties easier to manage and better expose how bias enters into the process. In my view, any approach to mitigating bias has to then involve the members of the social context in which it will be deployed. Such involvement can then lead to a more elaborate and deeper understanding of the societal implications of AI, rather than leaving that up to a select few in the design process. This technology is at its best when it’s representative of all, rather than simply trying to represent all through the eyes of the few.