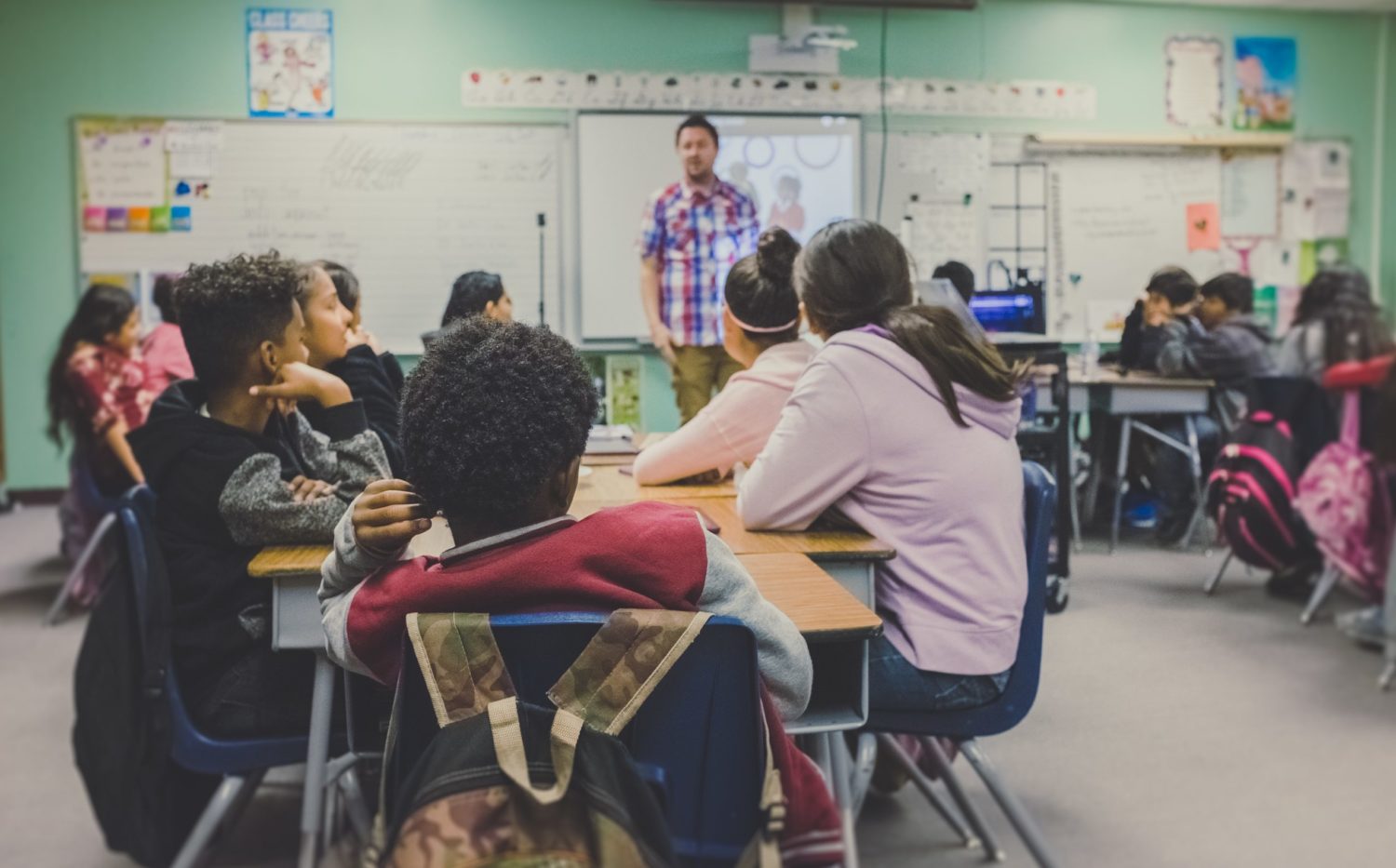
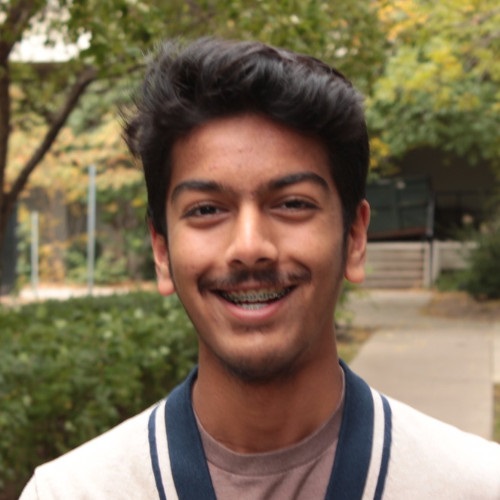
This guest post was contributed by Arnav Paruthi, a 16 year old student diving deep into the world’s biggest problems with the help of world class guidance from The Knowledge Society (TKS).
He has built many projects using reinforcement learning such as DQN’s to play Atari breakout and AlphaZero to play Ultimate Tic-Tac-Toe.
Previously he worked at fleetops.ai where he built a knowledge-based recommendation system which recommended truck loads to truck drivers, and built a data-pipeline using apache-beam + Google DataFlow.
A black man stole a car in Kentucky. He got caught for his crime, as he should, and ended up in court. As the judge went through the defendant’s profile, she factored a relatively new piece of information into her decision – the defendant’s criminal risk assessment. It’s a score generated by an AI algorithm which indicates the likelihood that the defendant will reoffend. The score is quite high for this particular case, so the judge gave the black man a two year sentence.
The next day a white man walks into the same court. He’s committed the same crime, and has a similar criminal history, so the judge concludes he should get a similar sentence as yesterday’s case. However, when she looks at the defendant’s risk assessment score, it’s much lower, so she lets him go with a $500 fine.
Obviously, the algorithm must have caught something the judge didn’t. Maybe the white man’s particular combination of criminal history, place of residence, and job lead to a lower risk of reoffending, but perhaps instead, the difference in risk assessments is due to AI bias.
AI isn’t just dangerous because someday in the future when we build an AGI, its goals won’t align with ours, or because bad actors could use its immense power to cause great damage. AI is dangerous today. It’s playing an increasingly important role in not only what ads you see, but who goes to jail, gets hired, or gets fined for fraud. These decisions have serious consequences on people’s lives, which is why it’s extremely important we make sure the systems making those decisions are fair and explainable.
I had the incredible opportunity to interview Abhishek Gupta, co-founder of the Montreal AI ethics institute, about his thoughts on how we should handle AI risk. These were my main takeaways.
Ethics guidelines aren’t enough
These days, everyone’s putting out AI ethics guidelines, from Google to the US government. These guidelines come from a good place, but the problem is that they’re not actionable. They don’t outline clear guidelines, and are open to interpretation. We can’t be extremely specific with AI ethics guidelines because much of it depends on the specific application, but we can be more specific than “Be accountable to people”.
Something Abhishek brought up which I hadn’t considered before, was how there is very little guidance on how dynamic systems should be maintained. For example, if we have a recommendation system which suggests content for kids, as it takes in new data and its predictions evolve, we want to ensure it doesn’t recommend violent content.
Abhishek suggested that governments mandate auditability of systems. That way they have to be explainable to normal people, and the audits would ensure the necessary steps were taken to remove bias from the system, in consultation with application specific experts.
We need cross-disciplinary collaboration
When engineers are developing AI systems, they ensure fairness of their system by looking at metrics like false positive rates, false negative rates, and counterfactual fairness. These measures are good, but they fail to capture application specific nuances. For example, if we have an algorithm which helps college admissions officers make decisions, it’s important we don’t take into account any demographic information in our decisions (race, gender, etc.). On the other hand with medical diagnosis, we probably just want the most accurate predictions, and use demographic information to make our decisions if it means the results are more accurate. In order to make these tradeoffs, engineers need to collaborate with industry professionals to make the right decision based on the specific application.
Along with fairness, another consideration is the transparency and explainability of a system. AI systems aren’t comprehensible by humans. What do all these weights and biases actually mean? Did the system output a low chance of cancer because it saw an anomaly in the CT scan, or because the person’s young and free from other illnesses? It’s very important for the users of these systems to understand how the system makes predictions, so they can use their own judgement in conjunction with the prediction to make the best decision. Herein lies another tradeoff — as we increase accuracy of a model, the complexity usually increases as well, leading to a decrease in explainability. Should the model be 1% more accurate if it’s half as explainable? It’s crucial to explain these tradeoffs to the users of these tools and consult them in making this tradeoff.
We need a cultural shift, along with policy changes
Policy helps, policy helps a lot, but what motivates and enforces policy changes is culture, both of the general people and those working on the technologies. There are loopholes in policy and ways to get around checks (think the Volkswagen emissions scandal), but if the public and people building these technologies understand and advocate for their responsible development, there’s a much better chance the technologies will be developed responsibly.
AI ethics and safety research is emerging from a niche field and becoming more mainstream. It’s important this continues so that more people learn about the dangers of the technology and become aware of the precautions they must take while developing systems.
The risk people aren’t talking about: Who’s developing these technologies?
When I asked Abhishek about the AI risk which people aren’t paying attention to, he brought up the lack of diversity of the people developing AI systems and AI ethic principles. They’re often white men living in the western world, and although they have good intentions, they have biases or blind spots, which will likely become embedded in AI systems which affect the entire world! These tools are used by everyone, they affect everyone, so the people who make them should be able to represent the interests of the entire world.
About two years ago, I watched a Ted Talk by Bill Gates where he warned the world about the threat of a pandemic. He encouraged countries to build reserves of medical workers, collaborate with the military to be able to quickly respond, and run germ simulations to find holes in their response plan. It seems that nobody listened to him, which is why COVID-19 is causing mass disruption to society. I fear that if we don’t take action soon, if we don’t make policy and cultural changes so that AI systems are fair and explainable, AI might do the same.