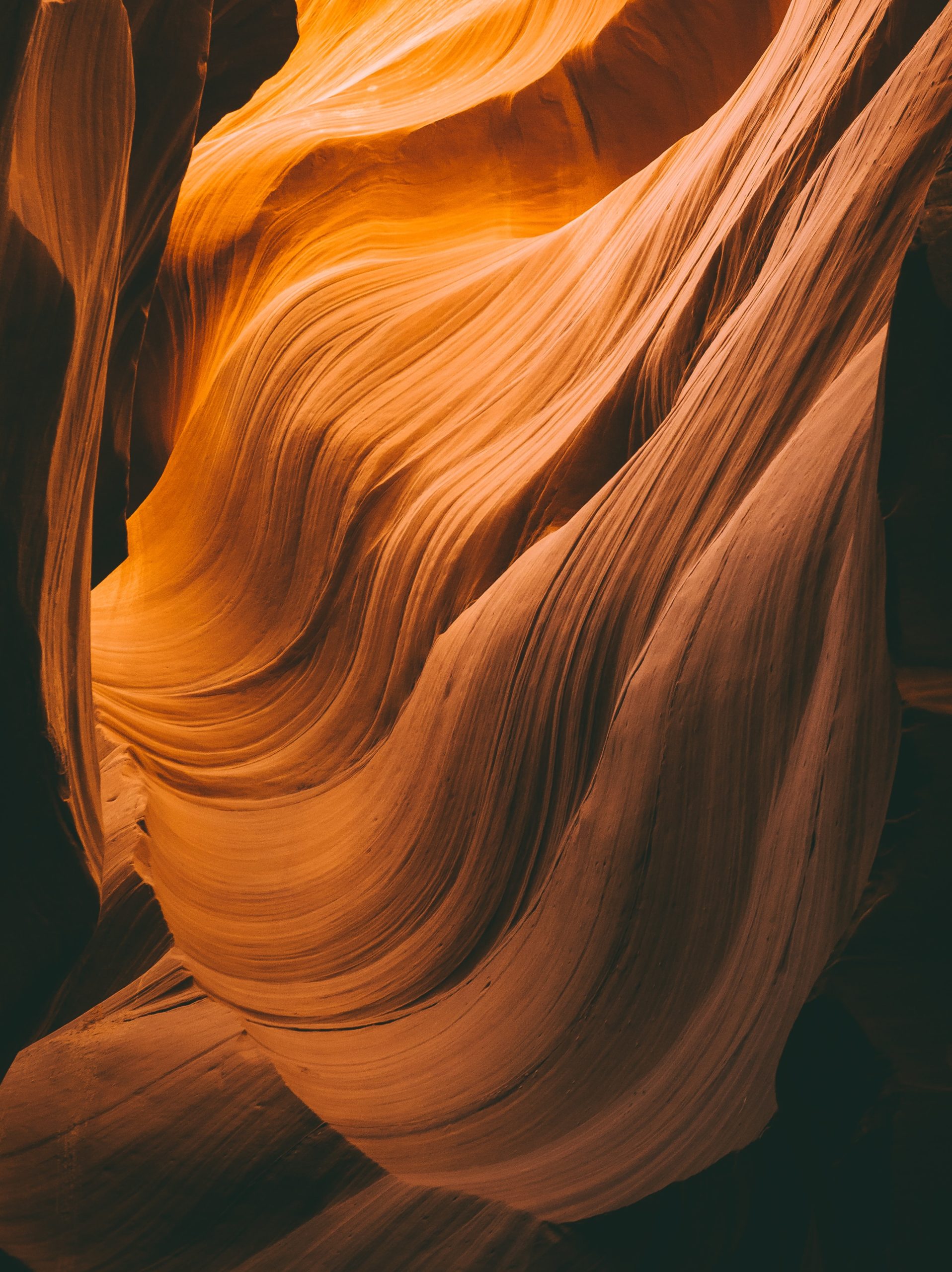
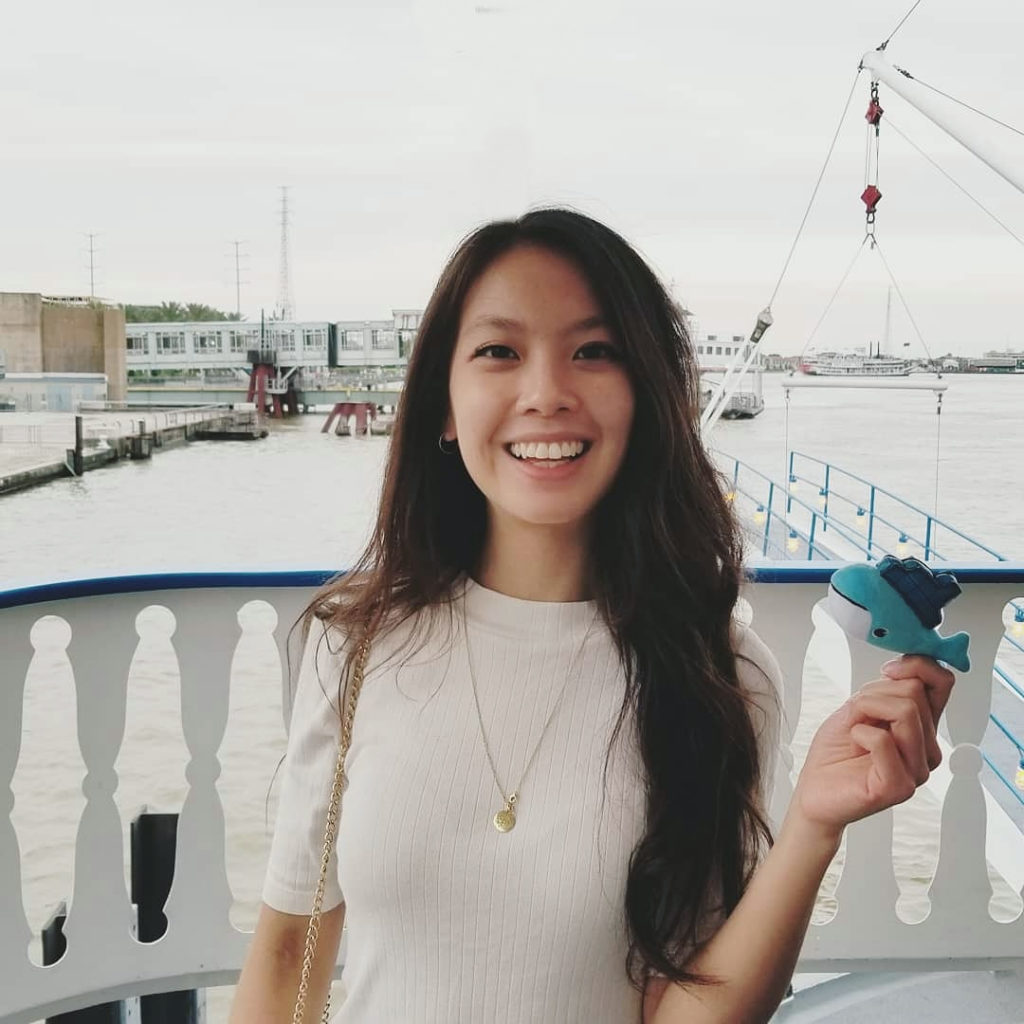
🔬 Research Summary by Helen Ngo, an affiliated researcher with the AI Index at Stanford HAI. She can be found on Twitter @mathemakitten.
[Original paper by Daniel Zhang, Nestor Maslej, Erik Brynjolfsson, John Etchemendy, Terah Lyons, James Manyika, Helen Ngo, Juan Carlos Niebles, Michael Sellitto, Ellie Sakhaee, Yoav Shoham, Jack Clark, and Raymond Perrault]
Overview: The 2022 AI Index report tracks, collates, distills, and visualizes data relating to artificial intelligence, enabling decision-makers to take meaningful action to advance AI responsibly and ethically with humans in mind. The 2022 edition includes a new chapter on technical AI ethics, highlighting metrics adopted by the research community related to the measurement of fairness and bias in artificial intelligence systems.
Introduction
AI systems are being broadly deployed into the world, but researchers and practitioners are also reckoning with their real-world harms, including systems that discriminate based on race, résumé screening systems that discriminate on gender, and AI-powered clinical health tools that are biased along socioeconomic and racial lines. These systems reflect and amplify human social biases, discriminate based on protected attributes, and generate false information about the world.
This year, the AI Index highlights metrics which have been adopted by the community for reporting progress in eliminating bias and promoting fairness. Tracking performance on these metrics alongside technical capabilities provides a more comprehensive perspective on how fairness and bias change as systems improve.
Highlights include findings that larger language models are more capable of reflecting biases from their training data, and a concrete shift in algorithmic fairness and bias moving from being primarily an academic pursuit to becoming firmly embedded as a mainstream research topic with wide-ranging implications, with researchers with industry affiliations contributing 71% more publications year over year at ethics-focused conferences in recent years.
Key Insights
Language models are more capable than ever, but also more biased
Large language models are setting new records on technical benchmarks, but new data shows that larger models are also more capable of reflecting biases from their training data. A 280 billion parameter model developed in 2021 shows a 29% increase in elicited toxicity over a 117 million parameter model considered the state of the art as of 2018.
Figure 3.2.3a and Figure 3.2.3b from the Gopher paper show that larger models
are more likely to produce toxic outputs when prompted with inputs of varying levels of toxicity,
but that they are also more capable of detecting toxicity with regard to their own outputs as well as in other contexts.
The rise of AI ethics everywhere
Research on fairness and transparency in AI has exploded since 2014, with a fivefold increase in related publications at ethics-related conferences. Algorithmic fairness and bias has shifted from being primarily an academic pursuit to becoming firmly embedded as a mainstream research topic with wide-ranging implications. Researchers with industry affiliations contributed 71% more publications year over year at ethics-focused conferences in recent years. This aligns with recent findings that point to a trend of deep learning researchers transitioning from academia to industry labs.
Multimodal models learn multimodal biases
Rapid progress has been made on training multimodal language-vision models which exhibit new levels of capability on joint language-vision tasks. These models have set new records on tasks like image classification and the creation of images from text descriptions, but they also reflect societal stereotypes and biases in their outputs—experiments on CLIP showed that images of Black people were misclassified as nonhuman at over twice the rate of any other race. While there has been significant work to develop metrics for measuring bias within both computer vision and natural language processing, this highlights the need for metrics that provide insight into biases in models with multiple modalities.
Between the lines
The Technical AI Ethics chapter of the 2022 AI Index captures a small facet of work within the broader AI ethics community, and exists as part of a wider ecosystem including those working on topics such as governance and societal norms. The field is changing quickly, and it will become important to assess impact as more data emerges along other ethical dimensions, such as the environmental impact of training large models. It will also be important to track this data over time as benchmarks and metric adoption changes within the research community to understand how the landscape shifts over time.