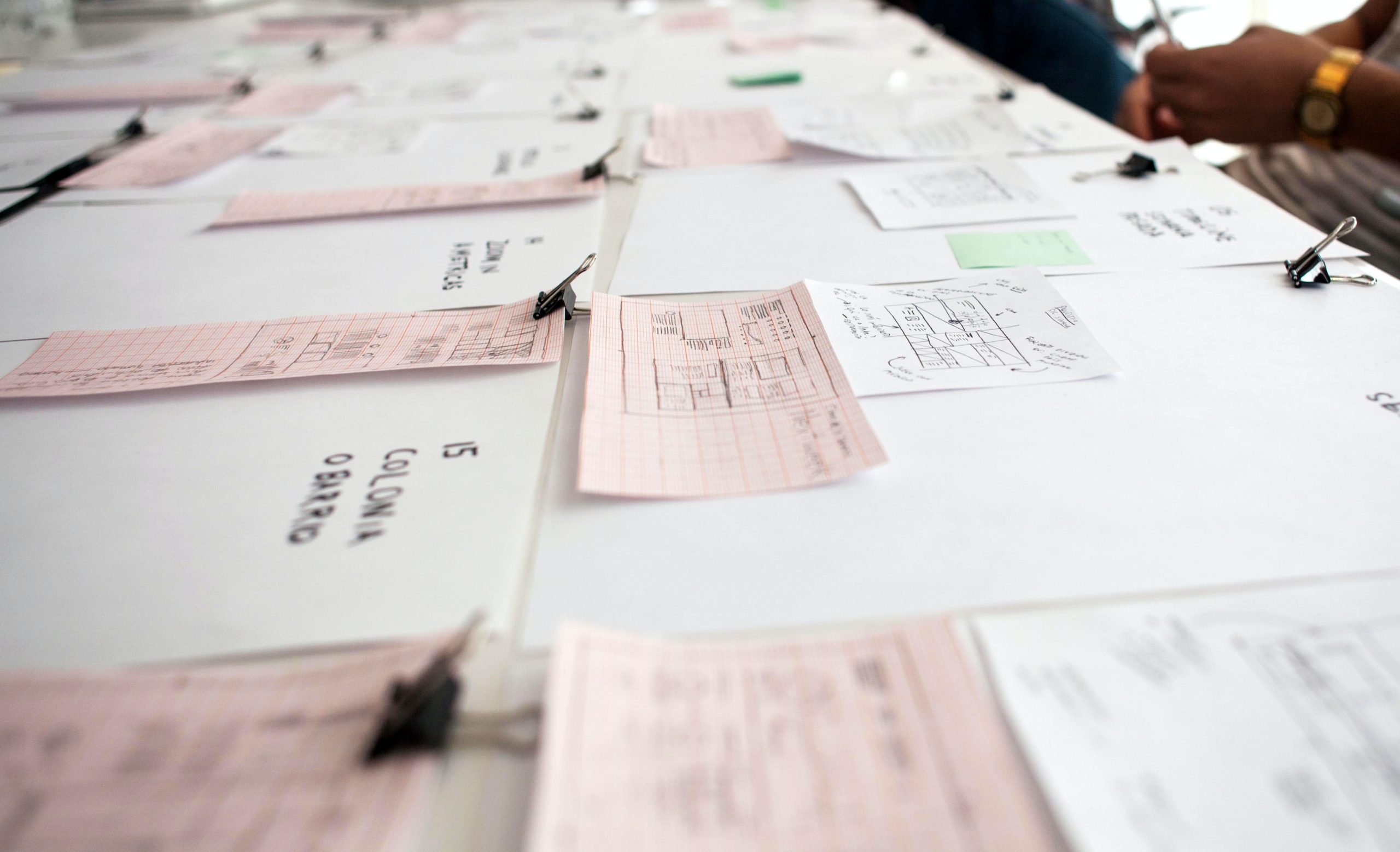
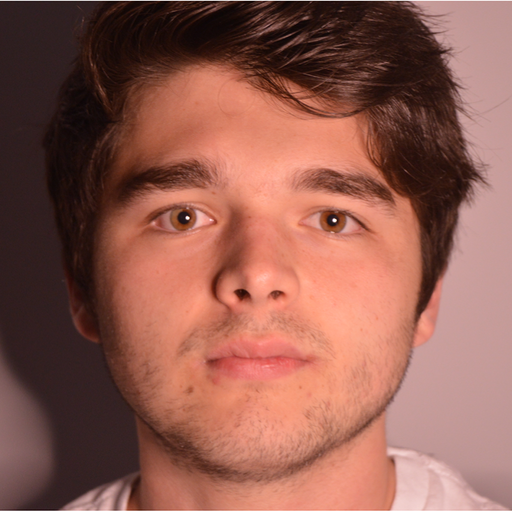
🔬 Research summary by Connor Wright, our Partnerships Manager.
[Original paper by Sasha Costanza-Chock, Inioluwa Deborah Raji, Joy Buolamwini]
Overview: The AI audit field is now larger than ever as a response to the variety of harms AI can cause. However, while there is consensus within the field, operationalizing such an agreement and the general commitment to the cause is questionable.
Introduction
The AI audit environment has expanded as a result of the harmful effects of AI, emerging as “one of the most popular approaches to algorithmic accountability” (p. 1). Hence, in this study, 10 individuals (deemed as experts in the field) were selected for interview from a pool of 438 individuals and 189 organizations, including first, second, and third-party auditors (p. 3). Here, first-party audits are done by internal experts, second-party audits are conducted by “external contractors” (p. 2), and external parties do third-party audits with no relationship to the company. Of the 189 organizations involved, 157 responded to the survey they received from the auditors. The authors then draw on this information to charter their observations of consensus and obstacles within the field, as well as formulate their policy recommendations.
In what is to come, I will touch upon the current AI audit landscape before detailing the paper’s main findings. I will then describe the common threads, obstacles, and policy recommendations observed and made by the authors. I’ll then conclude with my thoughts on the future of these audits.
Key Insights
The realm of AI audits
The AI audit landscape lacks consensus, standardized practice, and willingness to share system information and audit results. For example, while first-party audits generally have access to the entire internal system, these results are not usually publicly available. Hence, auditors are left without sufficient access to appropriately audit an AI system and an inability to help hold companies accountable for implementing the recommendations they provide.
Despite the lack of consensus on what it means to audit an AI system, this has not stopped legislation from being passed. For example, at the municipal level, New York City passed a requirement for all AI systems to be evaluated by a third party in 2021 (p. 3). With this in mind, I detail below the main findings established by the authors, with regulation forming the top priority on their list.
The main findings
- Regulation is needed to drive the AI audit space forward. Only 1% describe the current standards as “sufficient” (p. 6).
- However, currently, quantitative methods (such as assessing the robustness of an AI system) are preferred over qualitative methods (such as examining the effect of bias on the lives of stakeholders). Consequently, evaluating the context in which the technology is deployed is avoided, and the relevant stakeholders are not consulted.
- One explanation for the above is how it is hard to audit an AI system’s impact on a protected class of people due to the lack of sufficient data to audit.
- Helping to explain this is how, as a result, audit systems included in the study are found to be overwhelmingly bespoke; only 7% use a standardized methodology (p. 5).
- Within this, most auditors do not publicly share the findings of their audits. Consequently, this lack of transparency makes it hard to create generalized standards. Moreover, even when these standards are agreed upon, they become difficult to operationalize.
Nevertheless, I will now touch upon how the authors still managed to find common threads of standards and best practices agreed upon by the participants involved:
- The audit needs to be an interdisciplinary effort, focusing on the quantitative and qualitative aspects.
- There is consensus on enshrining AI audits in law, but also disagreement over what this entails (for example, the level of disclosure of the audit results).
- There is agreement that people subjected to automated decision-making must be notified.
- All of these audit approaches should be standardized and widely applied. If not, audits risk being too contextualized and, thus, ineffective.
While there is consensus in the AI audit space, I draw on the authors’ presentation of the main obstacles facing the field of AI auditing:
- The cost of conducting an AI audit and the company’s willingness to be audited are two major stumbling blocks.
- The next challenge is that the commitment to implement the audit recommendations is not widely shared.
- Second and third-party auditors find getting full access to the system they are auditing an issue, which first-party auditors do not.
- Consequently, reporting the results of the audit is still an issue.
- Above all, AI auditors are not prioritizing stakeholder involvement.
With these shared ideas and obstacles in mind, I detail below the policy recommendations made by the authors:
- Owners and practitioners should welcome external audits of their AI systems as part of necessary business practice.
- This can lead to a more significant effort in formalizing the evaluation and accreditation of AI auditors.
- Consequently, key findings of the audit should be made transparent for peer review.
- Increase the focus on qualitative aspects of AI systems. As part of this, stakeholders should be alerted when they are subjected to an automated system.
- In this way, businesses can prioritize stakeholder involvement.
Between the lines
It is noteworthy that the authors acknowledge that there are limitations to their study (such as their geographical focus is mainly in the Global North). However, I believe their report eloquently captures and exposes the agreements and struggles currently present in the realm of AI audits. For me, providing the incentive for companies to be audited will play a key part in making the space successful alongside stakeholder engagement. Whether it be beneficial accreditation or law-making, consultations, or grass-roots research, businesses can fortify this required field to benefit all stakeholders. In this way, an AI audit is not only to evaluate the AI system itself but the extent to which businesses prioritize their stakeholders.