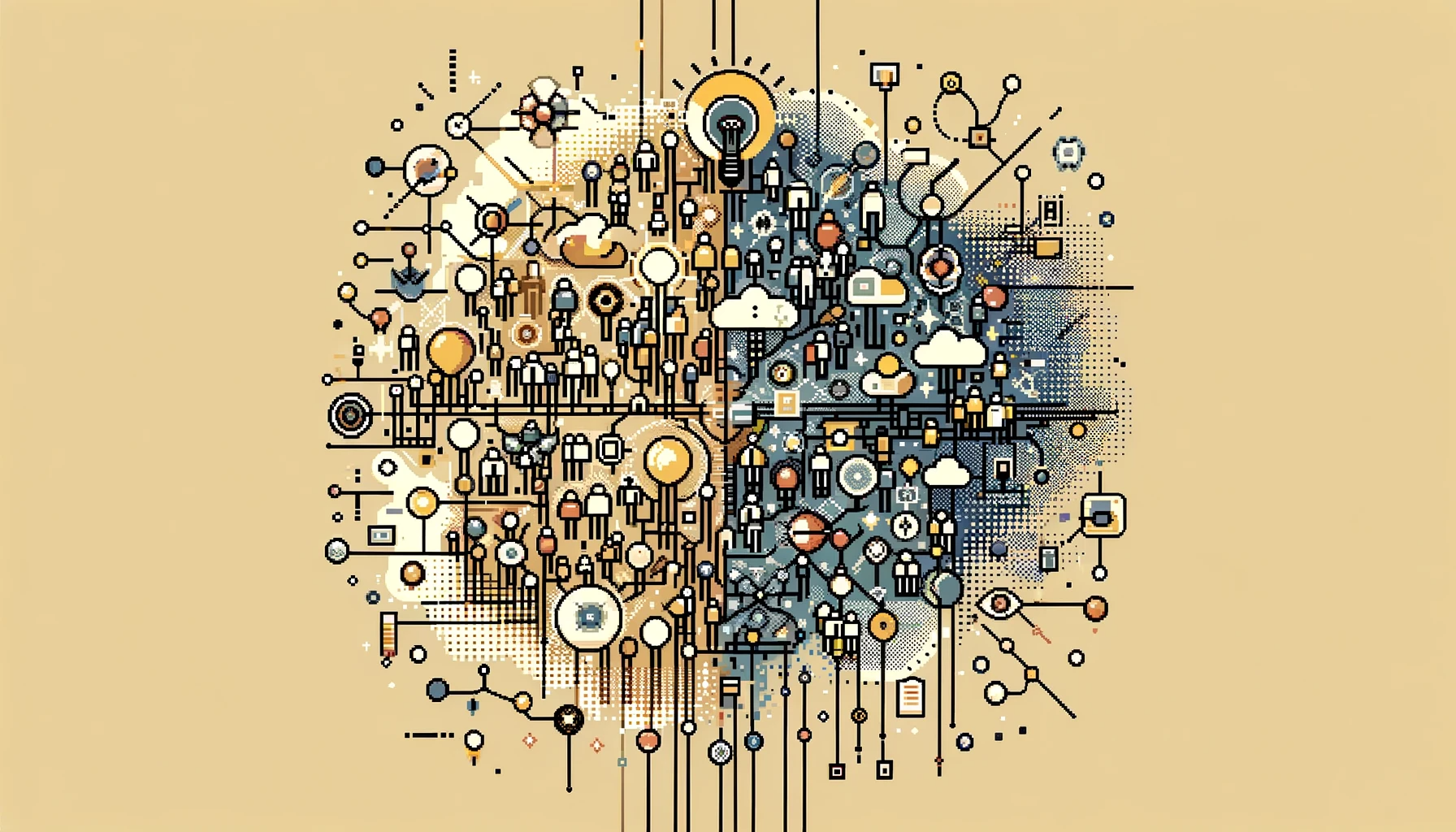
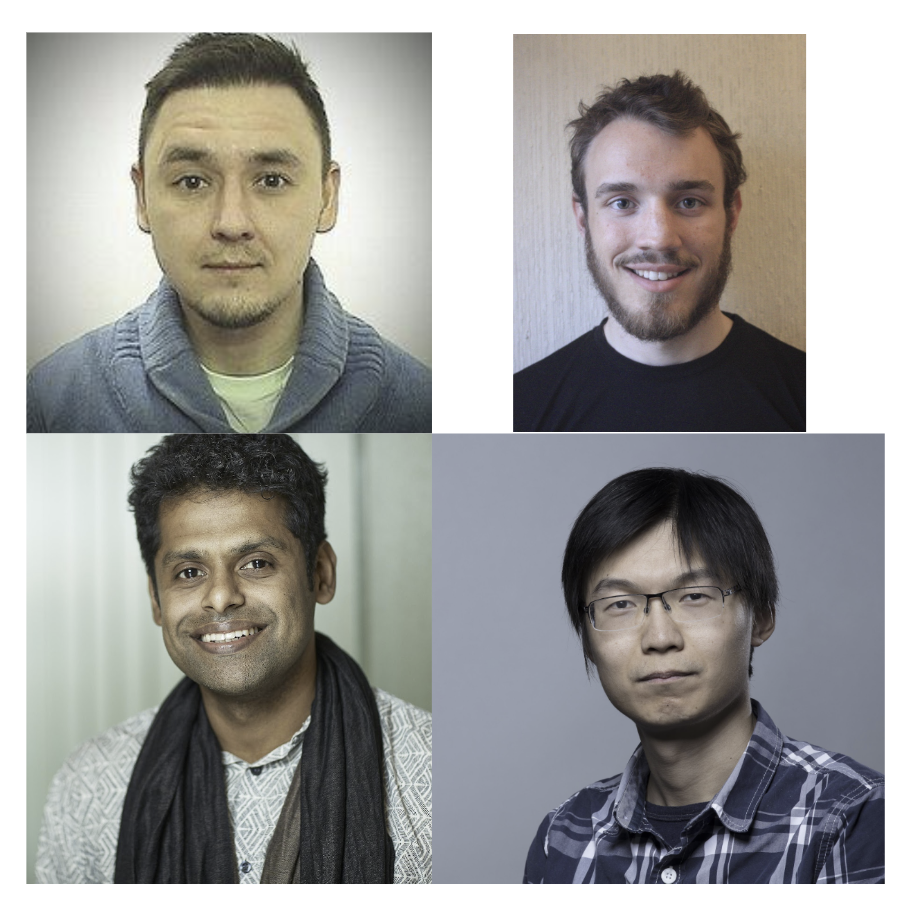
🔬 Research Summary by Rustem Dautov, Erik Johannes Husom, Sagar Sen, and Hui Song, researchers at SINTEF Digital (Norway) exploring the forefront of AI engineering within the IoT-Edge-Cloud continuum.
[Original paper by Rustem Dautov, Erik Johannes Husom, Sagar Sen, and Hui Song]
Overview: The growing dominance of major tech companies in the field of Generative AI has sparked a need for a transformative approach. This paper presents a conceptual architecture for community-driven Generative AI that emphasizes diversity, transparency, and collective decision-making. By fostering an ecosystem that encourages participation from diverse stakeholders. The research aims to democratize the development of AI models and shift the balance of power.
Introduction
Imagine an AI system that generates news articles mostly trained on data owned by a single tech corporation. This AI might unknowingly favor that corporation’s views and opinions, resulting in bias. It is not just about bias but also commercial interests. Technical giants might prioritize profit over fairness, shaping the AI to suit their needs rather than the wider community’s. Moreover, there are ethical concerns. A privileged group of entities controlling Generative AI means they decide what is right or wrong, which could lead to censorship and a lack of diversity in content.
The monopoly of giant corporations in Generative AI needs to be challenged to allow for a more balanced, transparent, and ethical AI landscape where various voices and perspectives can flourish. This research paper explores a fundamental shift in this field by presenting a conceptual architecture for community-driven Generative AI, challenging the dominance of major tech companies. The primary research question addressed is how to create an open and collaborative ecosystem for Generative AI that promotes diversity, transparency, and collective decision-making, in contrast to the conventional centralized approach.
Overall, the research underlines the importance of collaboration and diversity in shaping the future of Generative AI, emphasizing the need for the involvement of various stakeholders in building and moderating AI systems. It encourages researchers, technologists, content creators, policymakers, and civil society organizations to contribute to developing an open and inclusive ecosystem for AI, setting the stage for more transparent, accountable, and diverse AI models.
Key Insights
Community-Driven Generative AI: Leveraging Crowd-Sourcing and Federated Learning
The heart of the proposed architecture lies in crowd-sourcing and federated learning. Crowd-sourcing serves as a method to collect training data from a wide range of contributors, ensuring diversity and comprehensive datasets. Drawing on a broader pool of perspectives and expertise makes it possible to achieve the “wisdom of the crowd.” This collective intelligence arises from aggregating individual opinions, perspectives, and experiences, mitigating errors and biases. Federated learning complements this by preserving data privacy. Instead of sending data samples to a central server, individual clients conduct local training on their data. Only the updated model parameters are aggregated and shared, ensuring data control and security. These two pillars, crowd-sourcing and federated learning, underpinned by incentive mechanisms to motivate active participation and collaboration among diverse stakeholders, offer a unique approach to developing Generative AI models.
Challenges and Considerations: Intellectual Property Rights, Epistemological Relativism, and Moderation
Several challenges and considerations arise in the transition to community-driven Generative AI. One critical aspect is intellectual property rights (IPR). Participants in this ecosystem typically retain ownership of their data. Ownership of the global model may vary, and the paper highlights the need for clear guidelines and agreements regarding ownership and use of trained models. Copyright issues related to AI-generated content and derivative works are also significant considerations. With no single actor responsible for AI content, the community must determine applicable copyright ownership policies.
Another challenge involves reconciling the wisdom of the crowd with epistemological relativism. The crowd’s wisdom relies on aggregating diverse perspectives to ensure accuracy, but epistemological relativism posits that knowledge and truth are subjective and context-dependent. The paper stresses the need for a community-driven moderation framework to address biases and limitations in AI outputs. This framework ensures that diverse perspectives are considered, and moderation becomes an essential component of the proposed architecture.
Envisioning the Future: Bio-Inspired Decision Making and Federated Machine Unlearning
To overcome these challenges, the paper explores the concept of bio-inspired decision-making and federated machine unlearning. The human brain serves as an analogy for bio-inspired decision-making. The decision-making process in a human brain involves a global neuronal workspace, where different parts of the input are continuously analyzed and shared with other local processors. This process bears similarities to the majority and plurality principles observed in modern democracy. The paper suggests that applying this bio-inspired global workspace theory to community-driven Generative AI could be an intriguing and promising direction, albeit one in its early stages of exploration. Challenges such as effectively broadcasting local results and attracting the attention of relevant agents must be addressed.
Federated machine unlearning becomes a crucial component in community-driven AI. The paper emphasizes detecting and unlearning undesirable content from AI models. Sources of undesirable content include adversarial data, errors, biases, and sensitive information. Consensus-building and documentation throughout the unlearning process are essential to ensure transparency and accountability. Additionally, the level of unlearning should be quantified to evaluate the model’s effectiveness in forgetting sensitive information.
Conclusion: A Vision for Community-Driven Generative AI
In conclusion, this paper presents a visionary conceptual architecture for community-driven Generative AI. It advocates for a paradigm shift away from the dominance of the tech giants and toward a diverse, transparent, and collaborative approach. The proposed architecture leverages crowd-sourcing and federated learning to address intellectual property rights reconciles the crowd’s wisdom with epistemological relativism, explores bio-inspired decision-making, and underscores the importance of federated machine unlearning. The shift to community-driven Generative AI requires the collective effort of various stakeholders, including technologists, content creators, policymakers, and researchers. This paper offers a fresh perspective on the future of Generative AI, emphasizing the importance of transparency and diversity in creating AI models that benefit a broader range of stakeholders.
Between the lines
Having an open-source, community-driven approach to Generative AI is crucial because it promotes diversity, fairness, and innovation in the field. When only a few corporations hold a monopoly, they can intentionally and unintentionally introduce biases, commercial interests, and ethical concerns into AI systems. These biases can lead to unfair treatment, discrimination, and an unequal representation of different perspectives in AI-generated content. In contrast, a community-driven model involves contributions from various individuals and organizations, ensuring a broader range of voices and experiences are considered. This approach allows for collective intelligence, where the wisdom of the crowd can help mitigate individual biases and ensure more accurate and balanced AI outcomes. It also encourages transparency, as open-source AI models are accessible for inspection and scrutiny. Ultimately, an open, community-driven approach fosters collaboration, innovation, and collective decision-making rather than concentrating power in the hands of a select few, making Generative AI more accountable and beneficial for society as a whole.