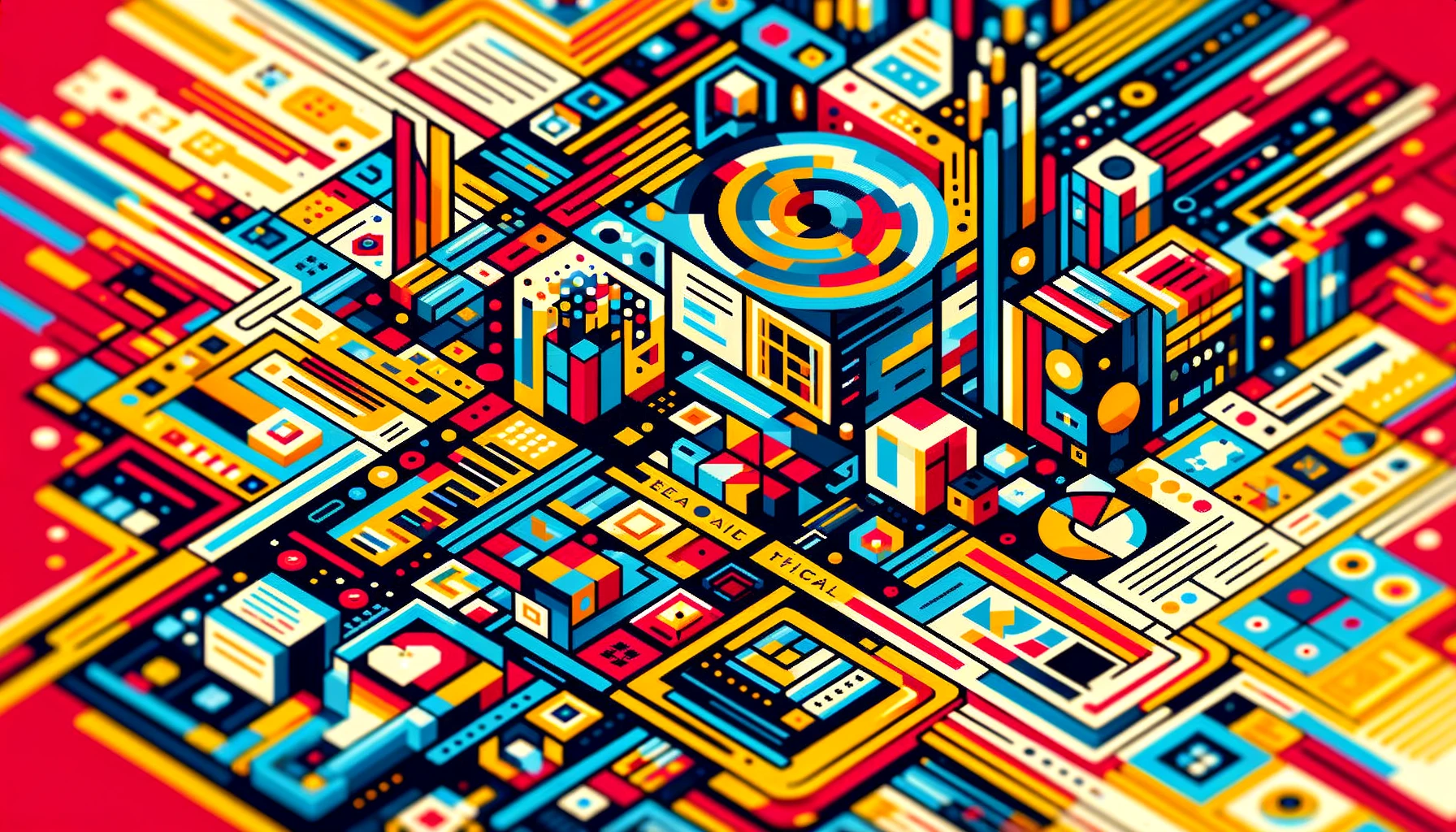
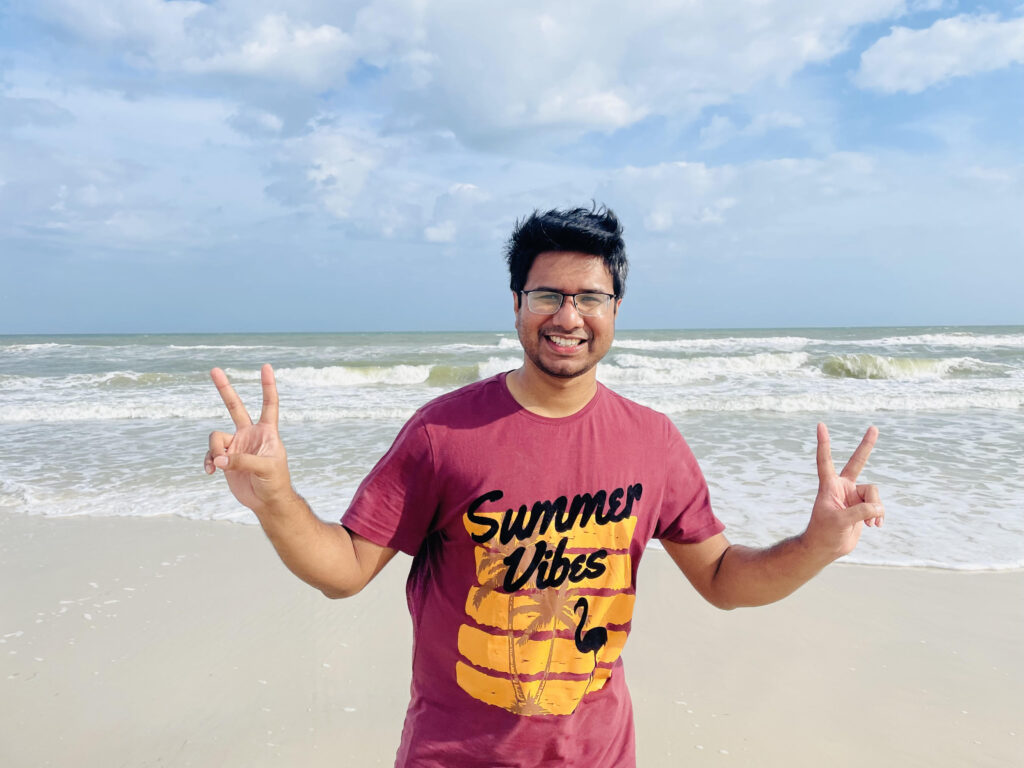
🔬 Research Summary by Forhan Bin Emdad, a third-year Information Science Ph.D. student at Florida State University, School of Information, actively researching Ethics, Fairness, Explainability, and Trustworthiness of AI in Healthcare.
[Original paper by Forhan Bin Emdad, Shuyuan Mary Ho, Benhur Ravuri, and Shezin Hussain]
Overview: With the increase in the use of large language models (LLMS), many ethical issues are rising. Big organizations like Open AI and Google compete with new technologies such as ChatGPT, Bard, and Gemini. However, there is a huge research gap in ethical considerations in this competition of Artificial Intelligence (AI). Therefore, there is a need for ethical AI implementation in every domain, especially in healthcare. For example, Google CEO Sunder Pichai emphasized hiring ethicists to develop LLM-based chatbots further.
Introduction
Ethics can be simply defined as how the world should act. Feelings, habits, religious beliefs, government laws, or cultural norms can achieve ethics. Researchers are delving more into the technological level of ethics to derive insights about implementing a robust ethical framework by analyzing the philosophy of ethics. Four orientations of the ethics lenses are mostly adopted: Kantianism or deontology, utilitarianism or consequentialism, contractarianism, and Virtue Ethics. In social science and computer science, utilitarianism is mostly used. Venkatesh’s unified theory of acceptance and use of technology (UTAUT) model is based on the utilitarian ethics philosophy.
Utilitarianism ethics mentions that AI actions should be for the greater good or happiness measured with utility. Utilitarianism is also known as consequentialism, meaning the action’s consequence should be for happiness. In utilitarianism, “utility” is the representation of individual goods. However, Societal good comes from the sum of individual utilities. In this paper, We investigated an important research question: “What ethical factors can influence the ethical AI design in healthcare?”
Key Insights
Study Method
We obtained a copy of the survey study the Pew Research Center (2020) conducted. This survey study included surveys and interviews with 36 healthcare domain experts from June 30 to July 27, 2020. The results of this study identify the major principles and challenges that will help the researchers to mold their healthcare AI design with proper ethical principles. From the query and analysis, the study identified that justice, privacy, bias, and lack of regulation are the principles with the most potential to help achieve ethical AI.
Need for a Unified Ethical Framework
Many researchers discussed the need to create a unified framework containing all the basic constructs of ethics (also referred to as bioethics principles), such as beneficence, non-maleficence, autonomy, and justice. A prominent researcher named Goirand et al. (2021) divided ethical challenges into different levels, such as ethical principle level, design level, technology level, organizational level, and regulatory level.
AI Challenges and Ethical Principles
This study’s data analysis, queries, and data pattern findings compelled us to reevaluate the Goirand et al. (2021) ethics level categorization (ethical principle, design, technology, organizational, regulatory) of healthcare AI challenges and ethical principles. To practically implement AI in healthcare, this study divided the technology level into data access-, algorithm-, and system-level. Algorithms can be the model that learns from data patterns to make predictions. The systems-level contains risks, accountability, and reliability, as system transparency can bring accountability and reduce risks. Similarly, there is a need for transparency in the algorithm, which can be implemented through interpretability and fairness, then the algorithm will be more useful in healthcare. Therefore, the algorithm-level consists of beneficence, bias, interpretability, justice & solidarity, and the data access level contains privacy. In addition, we categorized the principle of “lack of regulation” at the policy and organizational levels.
Proposed Utilitarian Ethics-Infused Framework
In medicine and healthcare, rule-based utilitarianism is better than act-based utilitarianism as pre-formed rules based on evidence assist in better decision-making, and there is no prediction or calculation of harm. Similarly, when technology assists decision-making, AI technology is developed from evidence derived from previous health record data. Our proposed model is a utilitarian approach for designing an ethical framework with the influence of variables derived from this study at different technology levels.
Potential variables are beneficence, justice, bias, interpretability, reliability, risks, privacy, and accountability. Moreover, our framework comprises the “lack of regulation” principle at the organizational policy level. In the AI4people framework proposed by Luciano Floridi, the ethical framework consisted of autonomy, beneficence, non-maleficence, justice, and a new variable, explicability, which represents “accountability,” meaning how AI can be responsible for the work. The goal of the AI4people ethical framework was to build a good AI society. However, challenges faced due to the appearance of new AI technologies and the frequent use of AI in healthcare have moved researchers’ focus toward some new important principles in the ethical framework.
Usually, in utilitarianism, the consequence of the actions should bring maximized happiness. Ethical principles influence these consequences of actions and require reporting guidelines. Our proposed ethical framework comprised important ethical principles as an influencer of actions at different technology levels in the utilitarianism approach for generating better healthcare outcomes.
Between the lines
Ethics is usually misrepresented as the ethical framework varies from domain to domain. Researchers usually mix different ethical principles and their application levels. However, application at the technology level has not been demonstrated deeply as ethical principles of data access, algorithms, and systems are quite different. Our paper provided a broader view of the technology-level application of ethical principles. However, this study has some limitations as we only analyzed small sample-sized data with only three coders. A more robust study can be conducted on the ethical AI framework by adding a survey study to quantify the results in the future.
The overall finding of this study suggests that AI experts are concerned about the successful ethical AI design in healthcare. Designing an ethics-infused framework of AI by mitigating problematic issues such as privacy issues, misuse of data, and interpretability can only result in greater trustworthiness of the system and an increase in the use of AI in the healthcare domain by clinicians, physicians, healthcare professionals, and other stakeholders. Adherence to the reporting guidelines can be the stepping stone toward the successful design of ethical AI, which can eventually lead to the actual use of AI in healthcare. Our study will pave the way to encourage future researchers to dive deeply into the ethical challenges presented by AI and find more efficient solutions to resolve them. Future work will provide examples of actions that fulfill the conditions of the approach based on the proposed framework.