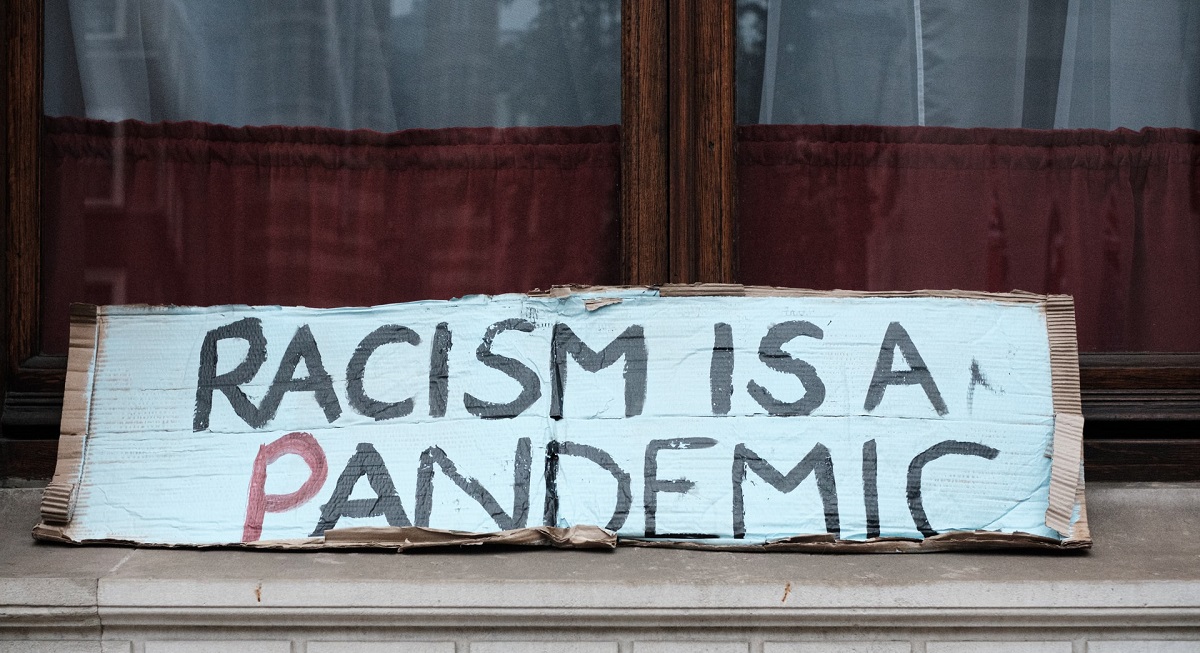
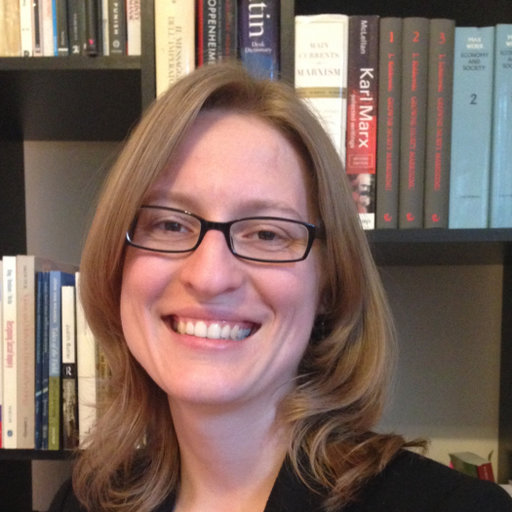
đŹ Research summary by Dr. Iga Kozlowska (@kozlowska_iga), a sociologist working on Microsoftâs Ethics & Society team where she guides responsible AI innovation.
âď¸ This is part 11 of the ongoing Sociology of AI Ethics series; read previous entries here.
[Original paper by Tressie McMillan Cottom]
Overview: Tressie McMillan Cottom brings together the concepts of platform capitalism and racial capitalism to study how modern-day economic changes wrought by digital technology are reshaping ethnicity, race, and racism. She explores how ideas of race and racial relationships and inequalities are produced and reproduced as more and more of our social lives are mediated online. She argues that by privatizing these interactions the Internet obscures much of these racialized relationships between producers and consumers and that the most vulnerable in society are brought into the fold but usually on exploitative terms.
Introduction
Is the Internet racist? Thatâs certainly not how Tressie McMillan Cottom would formulate her research question, but in short, the intersections of race/racism and digital society are her key research areas. In this paper, McMillan Cottom argues that the sociology of race has largely ignored the digital, and where the Internet is studied, it is often without a coherent theoretical underpinning of race, ethnicity, and racism. She proposes exploring this space through platform capitalism and racial capitalism and where the two meet. More specifically, she sees racial capitalism through two emergent phenomena: obfuscation as privatization and exclusion by inclusion. Letâs explore these concepts first and then apply them to the design of AI. Â
Platform capitalism tends to obfuscate the relationships between producers and consumers behind the digital screen. It hides the large amounts of data that it collects and locks them within walled gardens, making it difficult for consumers, the public, and researchers to access. By privatizing more and more social interactions through digital technologies, opaque commercial interests increasingly structure our relationships. Trade secrets and security are often reasons given for a lack of transparency.
Platform capitalism excludes through âpredatory inclusionâ which is the âlogic, organization, and technique of including marginalized consumer-citizens into ostensibly democratizing mobility schemes on extractive terms.â For example, online degrees, in theory, expand access to higher education but they also prey on predominantly lower-income African-American women to take out predatory loans. This results in huge costs to the student, particularly if they default, and big profit for the for-profit educational institution and the private loan lenders. We see similar exploitation in the âgig economyâ (more from McMillan Cottom on The Hustle Economy).Â
Thus given these recent phenomena, McMillan Cottom argues that âthe study of race and racism in the digital society should theorize networked scale, the logics of obfuscation, and the mechanisms of predatory inclusion.â Here the theories of racial capitalism â how networked capitalism reshapes global racial hierarchies and desires â come in handy to better understand how our online and offline lives are shaped and reshaped in racialized ways. So how can the concept of racial capitalism help inform the work of those who design and build platform services?Â
Designing Racial Capitalism
As McMillan Cottom describes it, the availability of Internet communications in the last couple of decades has reshaped the economy, producing an informal economy of part-time, gig workers, consultants, freelancers and entrepreneurs who find and get paid for work online rather than through a traditional full-time state/firm employer-employee relationship. This is enabled through platforms that bring together service providers and buyers like TaskRabbit, Upwork, Instacart, Uber, Lyft, and Amazon. This ecosystem of digital employment and services provides those who are unemployed or underemployed or who simply canât make ends meet with a regular full-time job with an opportunity to make extra cash on a one-off basis without benefits and usually in extractive conditions (little control over scheduling, limited recourse to abuse on the job, digital surveillance etc.). This informal economy relies on the most precariously situated workers in the formal economy, often women, people of colour, and immigrants. This racialized capitalist structure, rather than providing economic opportunity, serves to exacerbate racial and economic inequalities and shift the burden and risks of work from employers onto workers furthering the divide between capital and labour.Â
Knowing this, how can technology designers avoid contributing to these processes? Particularly in the space of AI? While many of the solutions will be on a macro-structural scale requiring public policy interventions, there are some things that the technology itself and those that build it can change. Letâs consider some AI design examples at all points of the machine learning development lifecycle.
Model Training: When designing facial recognition technologies for ride-sharing apps, for example, the algorithm needs to be assessed on racial impact to ensure it does not bias against people of colour, since misidentification can lead to job loss or lost pay and aggravate racial economic inequality. Preventing such harms may require retraining the model on better data, which may mean collecting a new dataset.
Data Collection: When collecting data to improve AI algorithmic accuracy, care must be taken to ensure that the data is racially representative of the problem being solved by the technology. The data collection must match the purpose for which the algorithm trained on that data will be used. The process of data collection must also be culturally sensitive and non-exploitative. This means issues like transparency, meaningful consent, data subject rights, and appropriate remuneration given the cultural and economic context must be considered. While the inclusion of people of colour into training datasets is important so that models can be trained to avoid racial bias, this inclusion must not be predatory, for example taking someoneâs image without their consent.
Model Deployment: Finally, any time algorithms that are to be used for performance evaluation or hiring/firing decisions, at a minimum, must not be racially biased. Because of the sensitivity and impactful consequences of this algorithmically-based decision-making, a human in the loop approach must be considered to avoid automated actions without human review. Additionally, workplace conditions should not be deteriorated through the use of technology (e.g. surveillance mechanisms) that diminishes workersâ freedoms, privacy, and dignity. For example, driver monitoring systems or warehouse worker tracking systems should consider issues around notice and consent, minimization of data collection, time and place of personal data storage, right to object to automated processing, and the right to contest automated decision-making etc. Technology designers and builders should speak up when there is no way to design a system that is not racially and/or economically exploitative given the socioeconomic context in which that technology will be deployed.
Between the lines
Just as sociologists of digital society must consider race and racism so race scholars must no longer relegate the Internet to the theoretical periphery. The same goes for practitioners. AI/ML researchers, data scientists and engineers, and UX designers can no longer put questions of race/racism and economic inequality to the side. Questions of racial and economic inequality in the age of digital transformation and platform capitalism cannot be peripheral as these very social institutions are shaped and reshaped by the technology we build. The story doesnât end at âbuild it and they will come.â Tech builders must ask the inevitable next question: “and then what?”