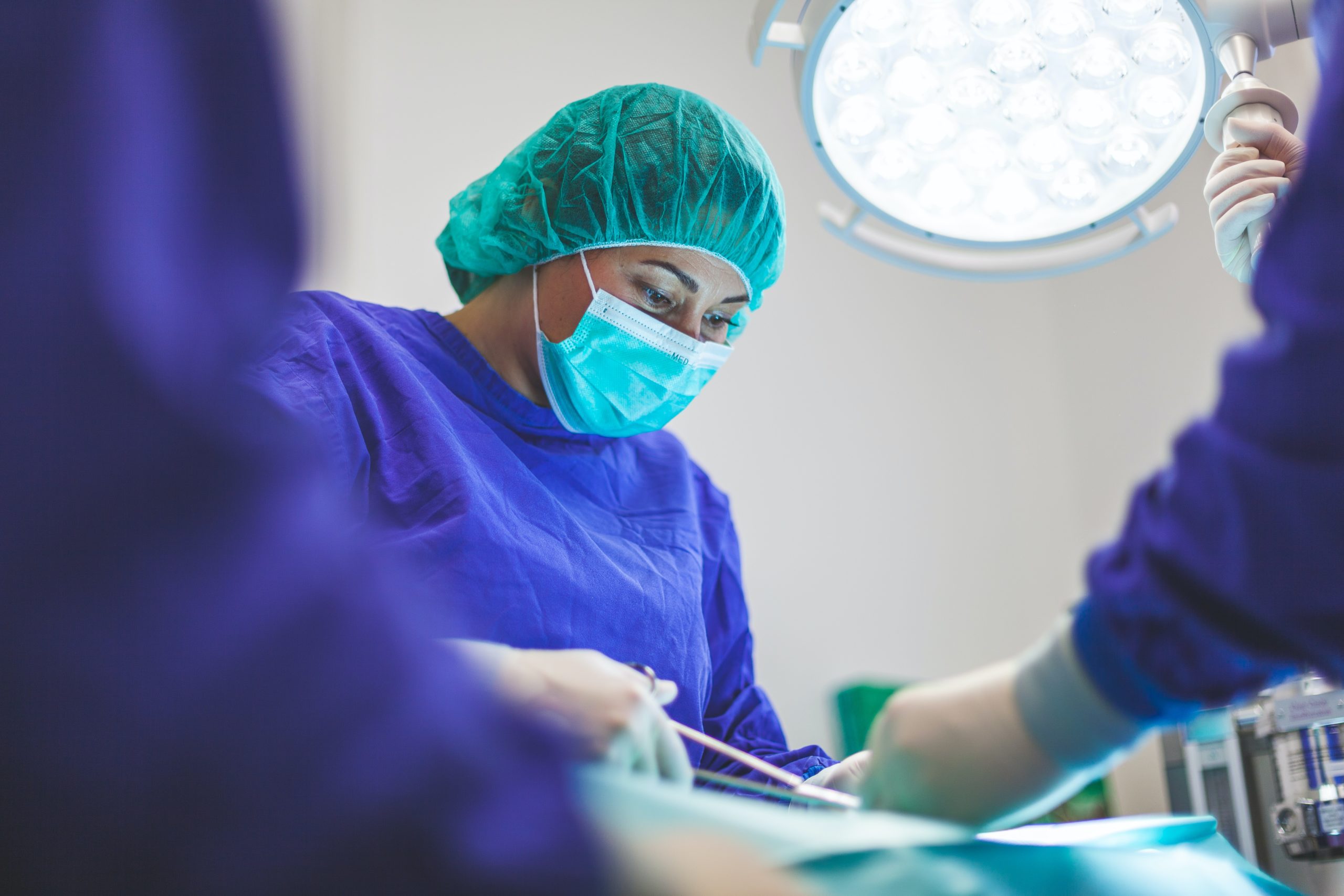
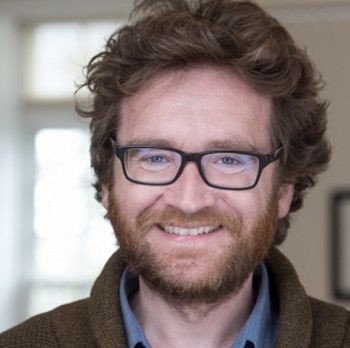
🔬 Research summary by Dr. Andrea Pedeferri, instructional designer and leader in higher ed (Faculty at Union College), and founder at Logica, helping learners become more efficient thinkers.
[Original paper by Andrea Ferrario, Michele Loi]
Overview: Can we trust AI? When is AI trustworthy? Is it true that “explainability fosters trust in AI”? These are some of the questions tacked in this paper. The authors provide an account for when it is rational or permissible to trust AI by focusing on the use of AI in healthcare, and they conclude that explainability justifies trust in AI only in a very limited number of “physician-medical AI interactions in clinical practice.”
Introduction
We often hear that “explainability fosters trust in AI” but few have explained what that phrase really means or whether it is true at all. The authors of this article attempt to give an answer to the question, when are doctors justified in trusting a medical AI system? To be sure, they are not tackling the question of whether it is true that doctors trust AI more when it is explainable. They are interested in the normative question: in what cases are doctors justified in trusting an explainable AI? They conclude that though in theory explainability may support doctor’s beliefs that AI is trustworthy, the reality is that this AI is yet not trustworthy and thus justified trust in AI is granted only “in a limited number of physician-medical AI interactions in clinical practice.”
Key Insights
Explainability. As the authors put it, explainability in AI consists of providing users with 1) understandable information on the “inner working” of the model, and 2) explanations of the model outcomes (Lipton, 2018). And we often hear that “explainability fosters trust in AI” in the sense that explainability is a good thing: it should and does produce more trust in AI.
Trust. What does it mean to trust AI? The authors are careful to distinguish various sense of trust and zoom in on what they think is key to the idea of trust: “Trust understood as essentially antithetical to monitoring (“trust as antimonitoring”), […] trusting means not controlling the actions of whom is deemed worthy of trust.” That is, real trust comes with the condition that you don’t feel the need to monitor the agent you trust: you just let them go do their things without the need to control. Given that, there is a form of trust that is key: paradigmatic trust. This form of trust takes place when “X holds a belief on Y’s trustworthiness and relies on Y without monitoring”. Now, justified or warranted paradigmatic trust “ is a monitoring-avoiding relation where the trustor holds a justified belief on the trustworthiness of the trustee” and does not monitor them because of this belief.
Warranted/permissible trust & explainability. So now the question is: if and when does AI explainability allow a doctor to hold a justified belief on the trustworthiness of an AI medical system, a belief that is needed for them to permissibly trust the AI? The authors believe that in theory explainability justifies beliefs on the trustworthiness of AI: a more understandable AI allows us to be better informed about the key components of the system and understand the results it provides. So explainability is instrumental to having a warranted trust in AI, at least in general. However, when we get to the specifics of the physician-medical AI interactions, the authors believe that explainability does not help paradigmatic trust. More specifically, “physicians are not rationally justified in lowering monitoring as a result of being presented with explanations that are successful according to the standard defined by current explainability methods.” The authors are concerned that for how things stand now, paradigmatic, non-monitoring trust is not justified, not even if an AI is explainable. As they put it, “the use of explainability tools in medical AI systems is fraught with challenges that affect both the possibility of a physician to form a justified belief on the trustworthiness of the AI and the possibility to calibrate monitoring because of it.” Medical applications of AI face so many challenges (starting with robustness), that there is no real justification for letting our guards down and letting monitoring go in these settings (except maybe for some very simple uses of AI in the clinical practice).
Between the lines
This paper does a good job at mapping the various ways in which we can trust AI while offering a reasonable account of how explainability interacts with trust. Questions still remain about what conditions provide justification for thinking that an AI system is trustworthy. This issue relates to the two following distinct questions: what features make AI actually trustworthy (e.g. robustness), and what kind of knowledge and beliefs an agent should have to be in a position to justifiably trust AI.