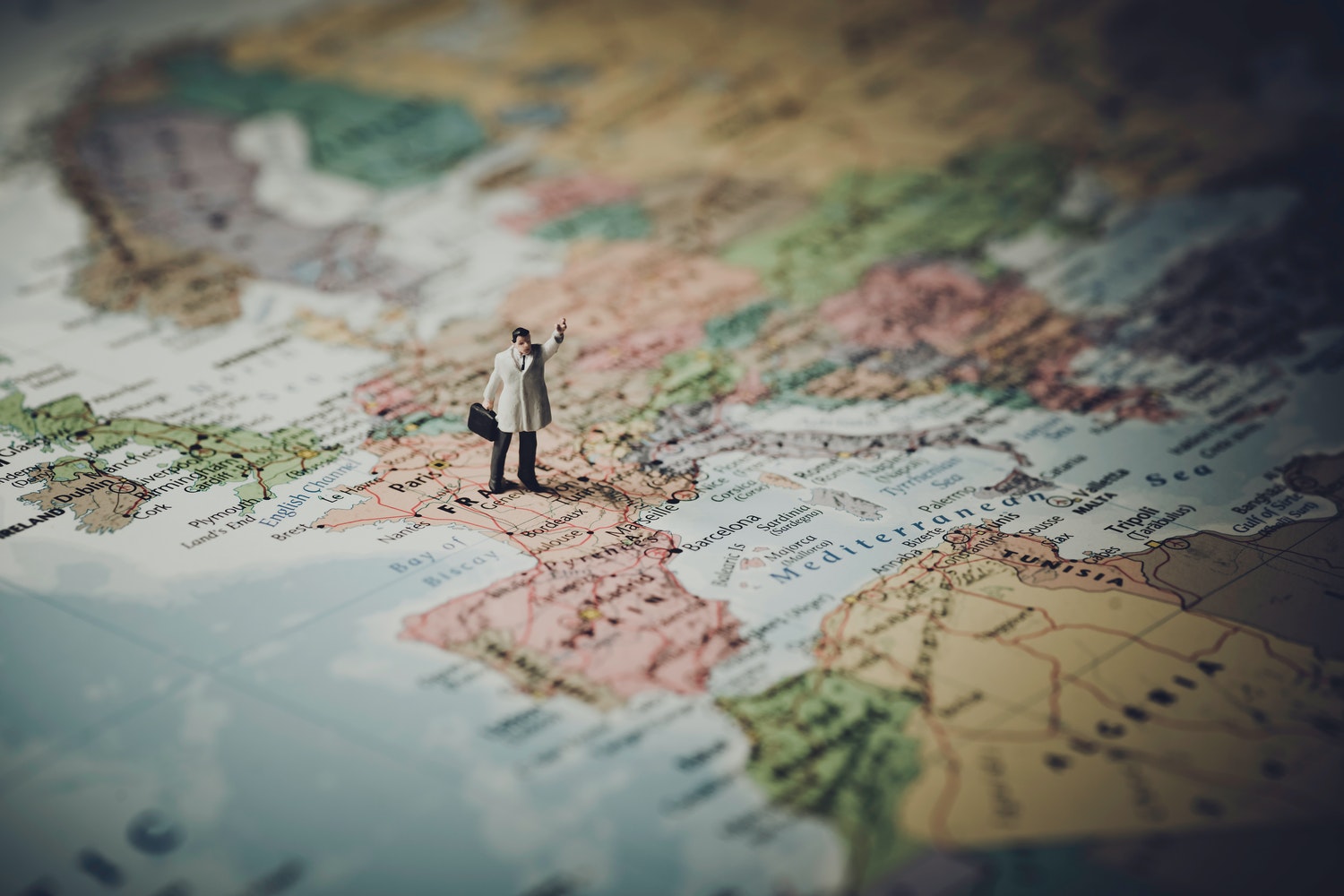
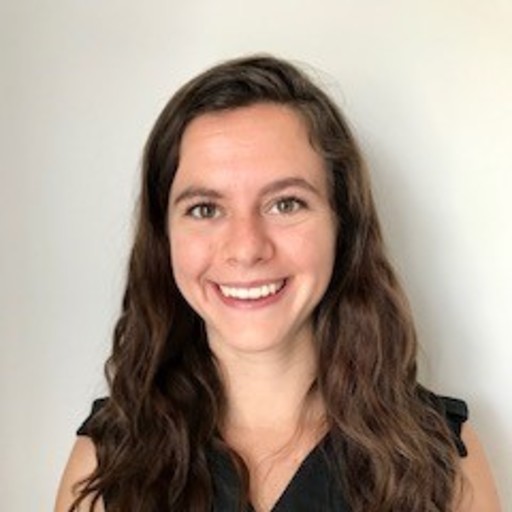
š¬ Research summary contributed by Alexandrine Royer, our Educational Program Manager.
[Link to original paper + authors at the bottom]
Overview: Western AI institutions have started to involve more diverse groups in the development and application of AI systems as a response to calls to level out the current global imbalances in the field. In this paper, the authors argue that increased representation can only go so far in redressing the global inequities in AI development and outline how to achieve broader inclusion and active participation in the field.
When it comes to AI development, the scales are generally tipped in favour of countries in the Global North. It is evident that “those best-positioned to profit from the proliferation of artificial intelligence (AI) systems are those with the most economic power.” To counterbalance these global inequities, Western institutions have called for more diverse groups in the development and application of AI.
For Chan, Okolo, Terner and Wang, greater representation can only go so far while structural inequalities remain unchallenged. To enact far-reaching change requires a redistribution of power. If we fail to provide a level playing field, Chan et al. caution that “the future may hold only AI systems which are unsuited to their conditions of application, and exacerbate inequality.” By taking a critical look at the limitations of inclusion in datasets and research labs, Chan et al. offer a list of potential barriers and steps to alleviating the power imbalances in AI development.
Invisible Players
The invisibility of countries in the Global South in conference publications is generally reflective of the broader inequality in AI development; it signals where innovation, hence funding, is happening. Both the NeurIPS and ICML, two of the world’s largest machine learning conference, did not feature countries from Latin America, Africa, nor Southeast Asia in their top ten publication index. In terms of the top ten institutions, the US dominated the list with 8, including familiar names such as Google, Facebook, and Microsoft. Left with a skewed perspective, the lack of contextual knowledge of institutions in the Global North towards the Global South’s realities can cause social and ethical harms in designing and implementing AI systems.
Calls for inclusion may lead to what Sloan et al. have termed “participant washing,” where “the mere fact that someone has participated in a project lends it moral legitimacy.” The term “participant washing” perfectly encapsulates the self-congratulatory tendency to treat representation as a series of quotas to be filled and boxes to be ticked, without ever engaging with the root causes.
Diversifying the Data-Gathering Pipeline
With its abundance of capital, well-funded research institutes and technical infrastructure, the Global North is well-positioned to lead AI innovation. However, its advantageous position is in large part due to riches accumulated through colonial exploitation. While calls to diversify the data-gathering pipeline are steps in the right direction, the authors delineate how the process is much more complicated ā it involves dismantling long-standing global inequities. When it comes to data collection, large image datasets, such as ImageNet and OpenImage, remain heavily US and Euro-centric. For Chan et al., current data collection practice “neglect consent and poorly represent areas of the Global South.” The focus on the accumulation of large ā and frequently uncompensated- data by foreign institutes to diversify datasets obscure whether such data should be collected in the first place.
As for data labelling, there are limited possibilities for individuals in the Global South to participate or achieve any form of upward mobility. Given the tedious and repetitive nature of the task, data labelling companies often seek out a low-wage workforce from the Global South, contracted via crowdsourcing platforms such as MTurk and Samasource. These third-party providers accentuate the disparity between data labelling companies’ profits and workers’ earnings, leading Chan et al. to assert that “in parallel with colonial projects of resource extraction, data labelling as the extraction of meaning from data is no way out of a cycle of colonial dependence.”
By being far-removed from the decision-making centers, “workers are contributing to AI systems that are likely to be biased against underrepresented populations in the locales they are deployed in and may not be directly benefitting their local communities.” Calls for participation in AI development often presuppose that members of the Global South have computing devices and internet connection readily available. Global power dynamics fuel the uneven growth of tech, leading Chan et al. to affirm that “It is instructive to view inclusion in the data pipeline as a continuation of this exploitative history.” As the chasm between data labellers and the downstream product deepens, these workers will continue to be severely exploited and alienated from the fruits of labour.
Rethinking Research Labs
With AI becoming increasingly present in global consumers’ daily lives, major tech companies, from Microsoft, IBM, and Google, have expanded their development centers and research labs outside of the Global North. Research labs in the Global South tend to be concentrated in specific countries, such as India, Brazil, South Africa, and Kenya. Fears over political and economic instability and a misguided view of local talent have led to limited investments in other areas. Chan et al. also underscore how many lab directors and staff within the Global South are frequently recruited from elsewhere; hence local representation is sorely lacking. As the authors argue, “to advance the equity within AI and improve inclusion efforts, it is imperative that companies not only establish locations in underrepresented regions but hire employees and include voices from those regions in a proportionate manner.”
True inclusion requires underrepresented voices to be present at all levels of a company’s hierarchy, including upper management. It follows that opportunities must be provided for local residents to acquire the skills and training needed for management roles and guide critical decisions. Some notable examples of grassroots AI education and training initiatives include Deep Learning Indaba, Data Science Africa, and Khipu AI in Latin America. The authors refer to the sentiment expressed by Makashane, a nonprofit organization committed to improving the representation of African language in natural language processing, that “we [Makashane] do not support shallow engagement of Africans as only data generators or consumers.” Actual representation will ensure that “the benefits of AI apply not only to technical problems that arise in the Global South, but to socioeconomic inequalities that persist around the world.”
Committing to Global Inclusion
When it comes to fast-paced economic development, South Korea’s import substitution industrialization policy (ISI), where the state endeavours to replace imports with domestic production to stimulate home-grown competitive industries, has offered an exemplar model. The authors suggest AI development could benefit from the lessons learned from ISI policies. Instead of relying upon “foreign construction of AI systems for domestic application, where any returns from these systems are not invested domestically, we encourage the formation of domestic AI development activity.” For ISI-like policies to succeed, Chan et al. affirm that ādomestic expertise must be developed in tandem to shape the future of AI development and reap its large profitsā. As American trailblazer and poet Audrey Lourde famously states, the “master’s tools will never dismantle the master’s house. They may allow us temporarily to beat him at his own game, but they will never enable us to bring about genuine change.” Global AI development, as it currently stands, is likely to leave the Global South to bear the burnt of algorithmic inequity. True global inclusion in AI, and the potential to bring genuine change, cannot be done without a redistribution of power.
Original paper by Alan Chan, Chinasa T. Okolo, Zachary Terner, Angelina Wang: https://arxiv.org/abs/2102.01265