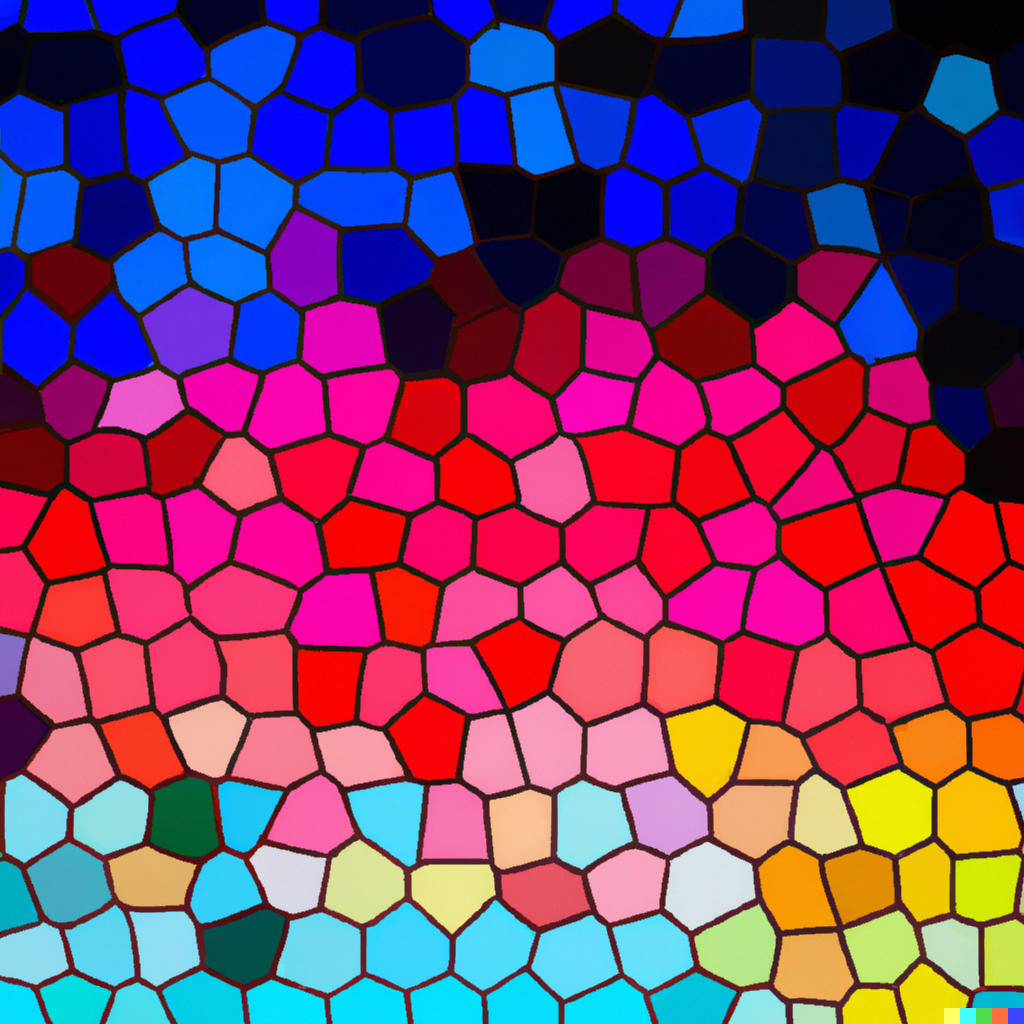
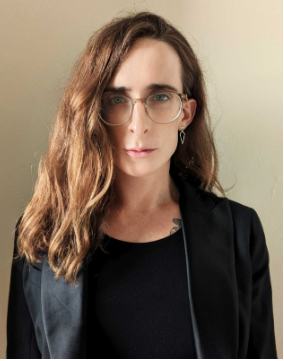
🔬 Research Summary by Blair Attard-Frost, a PhD Candidate and SSHRC Joseph-Armand Bombardier Canada Graduate Scholar at the University of Toronto’s Faculty of Information.
[Original paper by Blair Attard-Frost & David Gray Widder]
Overview: AI ethics practices and AI policies need more comprehensive approaches for intervening in the design, development, use, and governance of AI systems across multiple actors, contexts, and scales of activity. This paper introduces AI value chains as an integrative concept that satisfies those needs. We review the literature on AI value chains and the ethical concerns related to AI value chains, and we suggest five directions for future research, practice, and policy on AI value chains.
Introduction
AI ethics principles and practices often fail to prevent many societal and environmental harms. In response, many researchers have called for AI ethics to be re-centered around new principles or conceptual focal points such as design practices, organizational practices, or relational structures. In this paper, we respond to those calls by presenting an ethics of AI value chains that overcomes the limitations of many current approaches to AI ethics. By re-centering our ethical reasoning around the value chains involved in providing inputs to and receiving outputs from AI systems, we can more broadly account for and intervene in a wider range of ethical concerns across many actors, resources, contexts, and scales of activity.
Key Insights
Value Chains
To position AI value chains as an integrative concept for AI ethics, we first theorize the concept of a value chain with reference to the strategic management, service science, and economic geography literature. Building upon those perspectives, we define value chains as networking structures with three structural properties:
(1) Situatedness: Value chains are situated in specific contexts.
(2) Pattern: The resourcing activities involved in value chains are spatially, temporally, and organizationally patterned and thus capable of recurring with some degree of regularity.
(3) Value relationality: The resources and resourcing activities involved in value chains are perceived and evaluated differently by multiple value chain actors with aligned or misaligned values.
AI Value Chains
Building upon our theorization of value chains, we define AI value chains as those involved in developing, using, and governing AI systems. We review recent literature on AI value chains from researchers, industry, and governments. We find that research and industry literature on AI value chains has so far taken a generally narrow perspective on the ethics of AI value chains, centering ethical implications related to the computational resources required to develop and use AI systems (e.g., datasets, models, compute, APIs) rather than the larger social, political, economic, and ecological contexts in which computational resourcing activities are situated.
Some policy perspectives, such as EU and OECD AI policy initiatives and academic perspectives from the economic geography and critical AI studies literature, provide comparatively broad accounts of societal and environmental impacts upstream and downstream in AI value chains. However, a more comprehensive account of the actors, resourcing activities, and ethical implications of AI value chains remains a significant gap in the literature.
Ethical Implications of AI Value Chains
We apply the typology of AI ethics concerns developed by Stahl et al. (2022) to analyze the actors, resourcing activities, and ethical concerns involved in AI value chains across four categories: (1) benefits, (2) issues arising from machine learning, (3) ethics of living in a digital world, and (4) metaphysical issues. A diagram depicting how our analysis process was mapped onto these four categories can be found below.
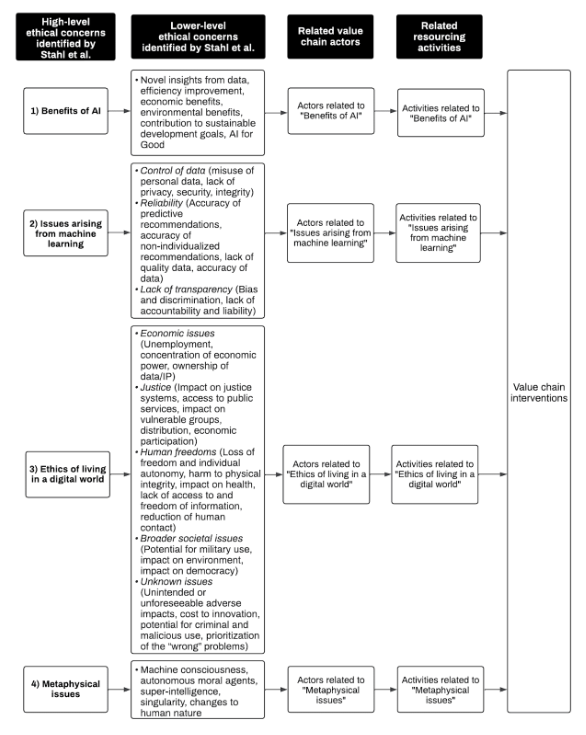
The potential benefits implicated in AI value chains are somewhat limited and involve activities such as the development and use of analytics platforms to obtain novel insights from data, the adoption of industrial AI applications to improve efficiency and service quality, potential economic benefits associated with job creation and hiring, optimization of energy consumption, and policy development processes.
Comparatively, the potential harms implicated in AI value chains are extensive and severe. Ethical concerns arising from activities related to machine learning include (but are not limited to) the provision of informed consent and authorization for data collection and use in training machine learning models; the sale, purchase, brokerage, and ownership of training data; the exchange of machine learning skills and knowledge, and the funding of machine learning research; the outsourcing of data annotation and verification work; the documentation, disclosure, and explanation of automated decision-making processes; the distribution and enforcement of liabilities for harms caused by machine learning technologies amongst value chain actors.
Beyond technical issues arising from machine learning, many broader societal and environmental concerns are implicated in AI value chains. The activities associated with these concerns include (but are not limited to) the use of AI applications in the hiring, contracting, dismissal, and surveillance of workers; distribution of capital, wealth, and other financial resources; distribution, open-sourcing, access to, and licensing of data, code, and other computational resources and intellectual property; public and private funding of AI procurement; inclusion of knowledge from marginalized groups in AI education, design, development, and governance value chains; worker compensation for data work and model work; energy, water, and mineral consumption and environmental footprints of AI systems throughout their software and hardware lifecycles; unforeseen misuses and abuses of personal data and digital identities enabled by AI systems; implementation and enforcement of excessively strict or excessively permissive AI regulations.
Finally, several speculative “metaphysical issues” can also be accounted for within the ethics of AI value chains, such as issues related to the development of artificial moral agents, conscious machines, and power asymmetries between humans and “superintelligence.” We argue that the resourcing activities involved in these issues are speculative extensions of present-day, real-world issues related to the development of AI systems by human moral agents, harms caused to conscious humans in AI value chains, and power asymmetries between humans. These speculative issues, therefore, do not warrant any significant study by empirically-grounded researchers or evidence-based practitioners and policymakers.
Directions for Future Research, Practice, & Policy
Based on our review of the literature and our analysis of the ethical implications of AI value chains, we identify four opportunities for researchers, practitioners, and policymakers to further investigate and enact the ethics of AI value chains:
- Conduct more empirical and action research on the specific ethical concerns, value chain actors, and resourcing activities involved in real-world AI value chains. Researchers can thereby provide a rich evidence base upon which other researchers, practitioners, and policymakers can conduct further research and governance on the basis of.
- Develop, apply, and evaluate the effectiveness of methods for systematically modeling AI value chains, identifying ethical concerns in AI value chains, and planning and enacting interventions in AI value chains.
- Design and implement ethical sourcing practices across all value chains involved in resourcing AI systems. Governance mechanisms such as industry standards, certification programs, procurement policies, and codes of conduct should also be used to support implementing and formalizing ethical sourcing practices.
- Design and implement legislation, regulations, and other policy instruments intended to equitably distribute benefits and responsibilities for preventing harm throughout AI value chains.