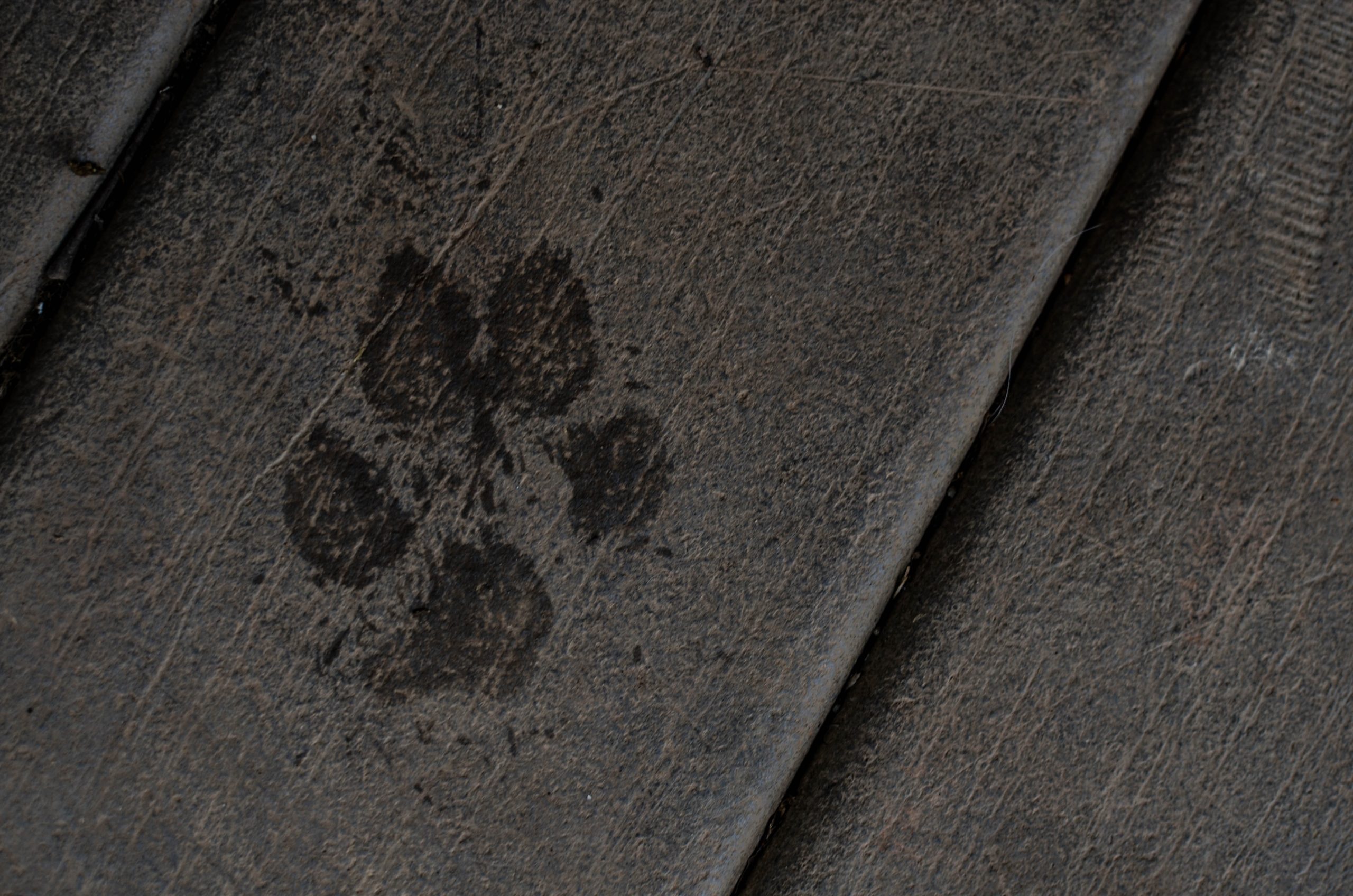
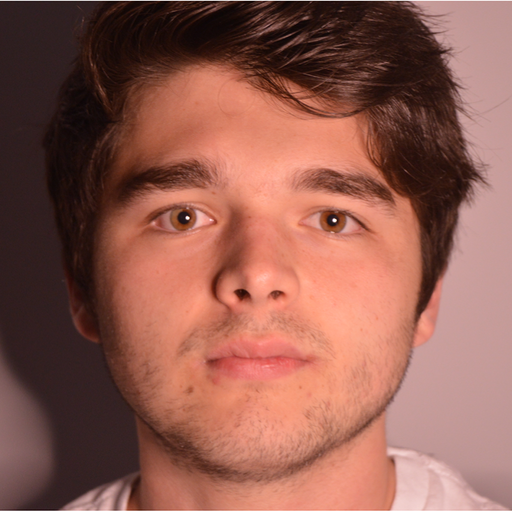
š¬ Research summary by Connor Wright, our Partnerships Manager.
[Original paper by A. Grinbaum and L. Adomaitis]
Overview: With the ability of large language models to reproduce text becoming more prominent (such as Metaās Galactica and GPT-3), it becomes increasingly cumbersome to distinguish between machine-generated and human-generated text. Consequently, the authors propose a watermark technique to separate the two to avoid the grave dangers of manipulation.
Introduction
Large language models are being treated as one of the research areas in AI with the greatest promise (where AI companies in healthcare have increased the portion of their budget dedicated to these models). Consequently, mastering AI-generated texts is becoming increasingly difficult to distinguish the machine-generated and human-generated text. As a result, the authors propose focusing on watermarking methods to preserve this distinction. What results is the problem of āindistinguishability.ā
Key Insights
The problem of āindistinguishabilityā
Since the Turing test, ā[i]ndistinguishabilityā (p. 2) has become the benchmark for positive AI performance. However, the current regulation does not clearly distinguish between human-generated and machine-generated text. The latter does not possess the critical thinking and reflection of the former. In this way, AI can indeed reproduce text, but it will not be able to reflect the meaning of the text it produces. That is to say, it may be able to produce scientific papers like other language models such as Galactica, but it will not possess the ability to acknowledge it is producing fake news. Without a clear distinction between the two, it becomes difficult to impose sanctions on instances of manipulation.
Manipulation
Tying into the debate surrounding truth and deepfakes, AI-generated pieces are not as trustworthy. They cannot be held to the same account as human-generated pieces, according to the authors. Given the opportunity to manipulate at scale, AI can use emotionally charged language to influence the user. Within the text, this is demonstrated in the conversation between Joshua and Jessica, with Jessica (the language model) using specific terminology to persuade Joshua that it is Jessica lives on despite passing away. These types of stories not only taint the possibility of detecting what is true but also a positive view of technology. Hence, distinguishability is crucial not only for preserving truth but also for the beneficial view of technology.
To preserve these two perspectives, the authors propose the watermarking technique.
Watermarking
Watermarking techniques look to provide clear signs of whom the piece of writing in question belongs to. These techniques include a hash function to generate a specific bit sequence (a row of 1s and 0s) alongside steganographic approaches (hiding a secret message within a normal body of text).
The authors propose equidistant language sequencing as a non-intrusive watermarking method. This is where a word/letter is repeated at regular intervals in the text without interrupting the user’s reading experience. For example, the language model may repeat the letter āaā every 70 characters to signal that it is machine-generated. This may not be visible to the human eye immediately, but it will serve interested parties well when required to distinguish the machine-generated and human-generated text.
Between the lines
The watermarking technique, while in its infancy, is important to consider. Even when we know we are talking to a machine, we still tend to project mental states onto the AI and anthropomorphize the technology. Hence, a discernable sign to help prevent emotional manipulation by machines will prove a crucial step in the ethical safeguards proposed for these technologies.
However, the type of watermark proposed may need to be clearer. While we donāt want to muddle the dataset by adjusting the data to contain a visible label, requiring someone else to decipher the watermark may prove an avoidable step. Instead, having the piece labeled as āmachine-generatedā like in the self-disclosure agreement in the California chatbot law could prove a helpful step in the fight against manipulation.