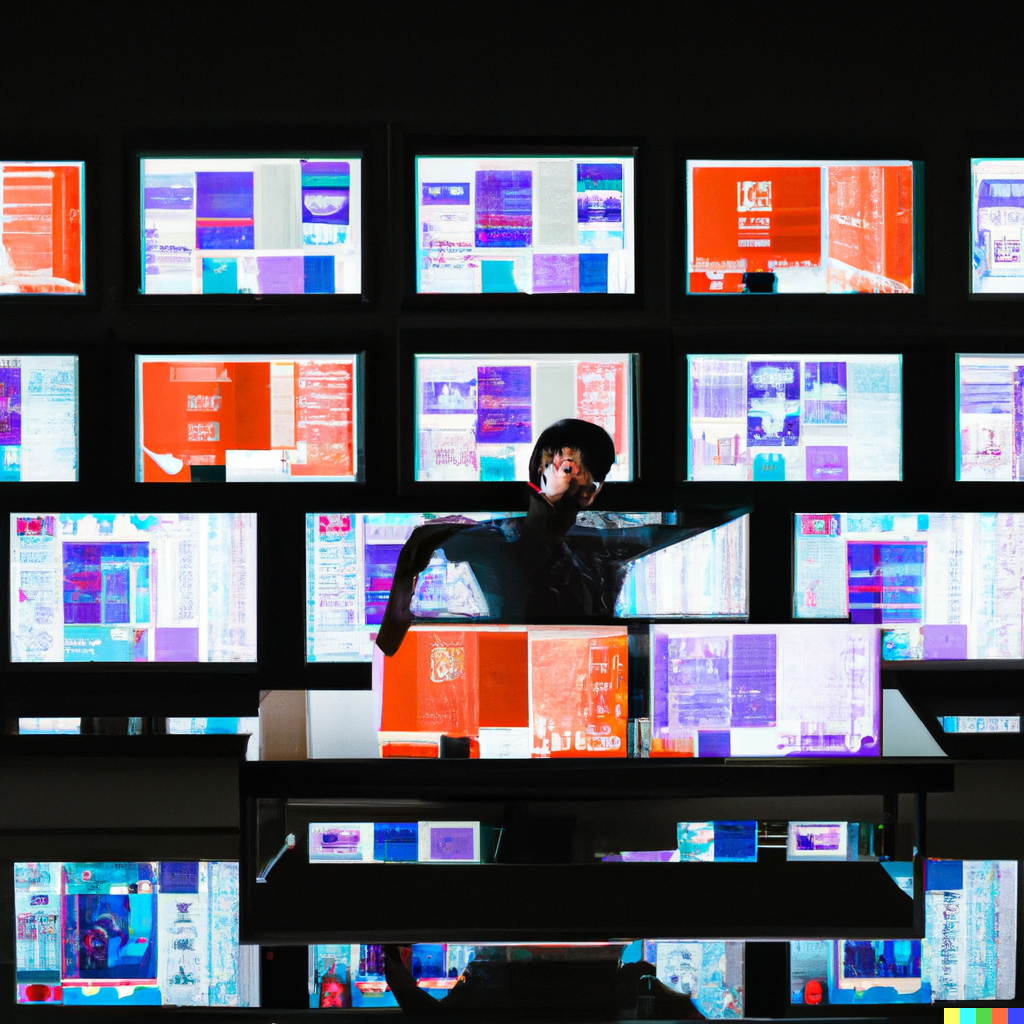
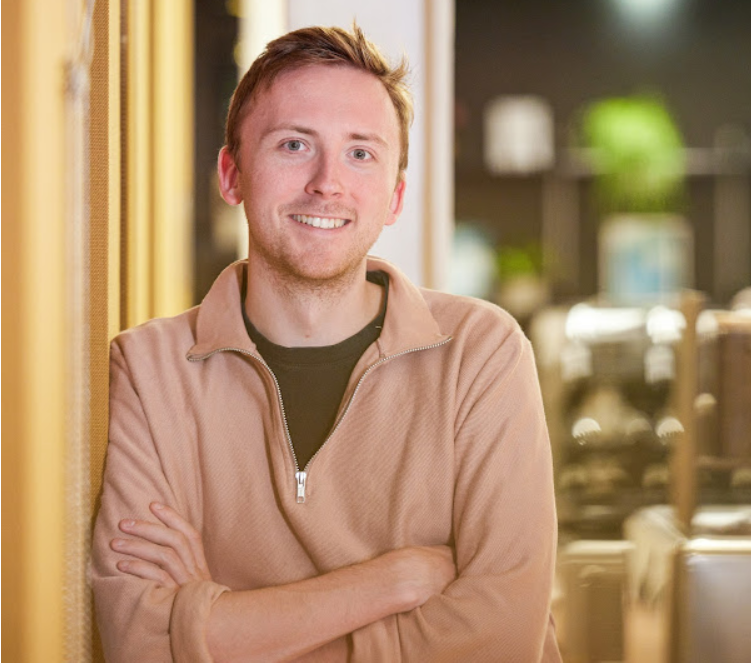
🔬 Research Summary by Will Hawkins, Operational Ethics & Safety Lead at Google DeepMind.
[Original paper by Will Hawkins & Brent Mittelstadt]
Overview: Breakthrough AI research has increasingly relied upon engagement with online crowdsourced workers to collect data to ‘enrich’ models through processes such as “Reinforcement Learning from Human Feedback” (RLHF). This paper assesses how AI research papers involving data enrichment work consider ethics issues, such as consent, payment terms, and worker maltreatment, highlighting inconsistency and ambiguity in existing AI ethics disclosure norms.
Introduction
It is no secret that leading large-scale AI systems such as ChatGPT, Bard, and Claude rely on huge amounts of human-generated data to improve or enrich their models. In recent months, scrutiny over ‘data enrichment’ work, often performed by crowdsourced remote workers, has been highlighted in articles from TIME and Verge, identifying issues relating to payment terms, consent, and worker maltreatment.
This work analyses the research papers driving the development of these systems, exploring how and to what extent ethics requirements and norms have developed for AI data enrichment work. The paper takes a longitudinal approach to assess how researchers engage with ethics issues compared with other fields with established research ethics norms, such as Psychology.
The work finds that existing ethics norms are inconsistently followed by AI researchers engaging with data enrichment workers, and workers are frequently unrecognized and under-considered in papers relying on huge amounts of crowdwork input to develop. These conclusions suggest a need for more meaningful governance for AI research engaging with data enrichment workers.
Key Insights
What is data enrichment work?
The Partnership on AI has defined data enrichment as “data curation for the purposes of machine learning model development that requires human judgment and intelligence.” In a research setting, in practice, this means human workers undertaking tasks such as labeling existing data, evaluating system performance, or producing new data to enrich a model (see Shmueli et al. for more).
The need for huge amounts of curated data for large models has led to the rise of platforms offering these types of services, ranging from open-call crowdwork platforms such as Amazon’s Mechanical Turk (MTurk) and Prolific to bespoke data enrichment providers like Scale and Surge. The reliance on ‘gig economy’ crowdworkers is essential to the success of data enrichment platforms.
Why does data enrichment work raise ethics issues?
Crowdsourcing platforms are often utilized due to their low costs, and consequently, many critiques of crowdwork relate to payment. MTurk allows requesters to place tasks online for as little as $0.01 per task, with mean payment rates estimated at around $3 per hour. Considering around 75% of MTurk workers are based in the US (with 16% based in India), rates can be far below federal minimum wage levels.
Beyond payment, data enrichment workers have been hired to identify harmful content, meaning an individual might be asked to label or evaluate data that could be pornographic, violent, or otherwise offensive. Studies might not always adequately explain the nature of the content involved in a task, or workers can be subjected to task rejections without explanation. The lack of security for crowdworkers has meant they can be treated as interchangeable, with minimal recourse or feedback mechanisms. Consequently, their contributions have been dubbed ‘Ghost Work.’
In a research setting, ethics issues are typically governed by Institutional Review Boards (IRBs) or research ethics committees. However, US law only requires this oversight in federally funded projects and where the human participant is the subject of the study. The law was not designed for engaging with gig economy workers or for research driven by private entities, and therefore many studies may fail to adequately consider ethics issues.
How does AI research engage with research ethics issues?
As a result of these issues, this study analyzed the contents of academic papers from leading AI research venues, NeurIPS and ICLR, to understand if and how AI researchers engage with ethics issues related to data enrichment work. It found that at these venues, ethics oversight from a body such as an IRB was rare, with just 5% of relevant ICLR papers between 2018 and 2022 involving crowdsourced workers disclosing any form of research ethics oversight.
It also found that payment rates and consent information was infrequently disclosed in papers across major conferences. This is despite NeurIPS introducing a requirement to disclose payment rates in papers, with only 54% of crowdsourcing papers adhering to this in 2021.
How does this compare to other fields?
The work concluded by comparing these findings to other venues and fields with a deeper history of engagement with crowdworkers and participants, such as Psychology. The results showed that Psychology papers engaged with research ethics questions far more frequently, and papers from venues focused on human-computer interaction, such as CHI, also engaged with research ethics issues at a far higher rate.
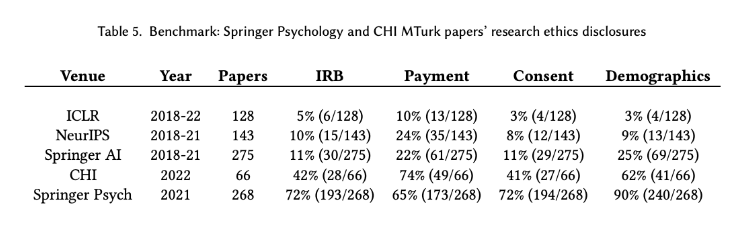
Why does this matter?
Data enrichment work is becoming increasingly essential to the AI supply chain, but a lack of consideration for the people involved in these tasks can lead to widespread maltreatment. Addressing this issue at the level of AI research is essential because of the field’s reliance on academic literature to develop state-of-the-art AI systems. This paper concludes with three overarching findings:
- Papers at leading AI research venues do not align with traditional research ethics disclosure standards, partly due to the differing nature of tasks falling beyond the initial intentions of research ethics oversight laws, leading to inconsistency and ambiguity over appropriate norms.
- Research journals and conferences have the power to influence engagement with research ethics by creating and enforcing policies addressing ethics issues.
- Leading AI research breaks with scientific tradition by scarcely considering demographic impacts, which can lead to hidden compounding biases in systems developed using this data.
Through further engagement with data enrichment workers and consideration of ethics issues, this paper seeks to improve workers’ conditions and encourage a more sustainable supply chain for AI models in the future.