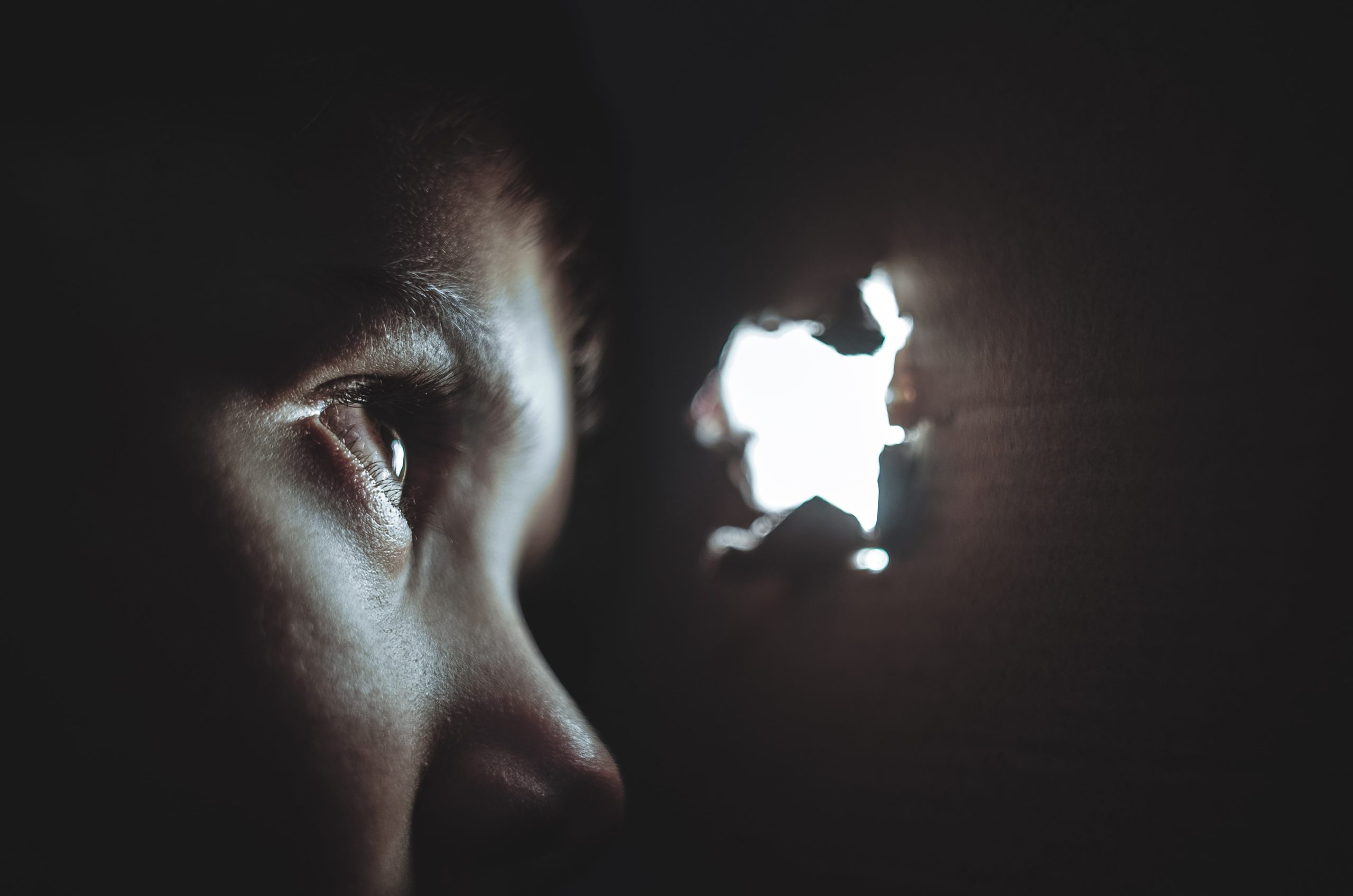
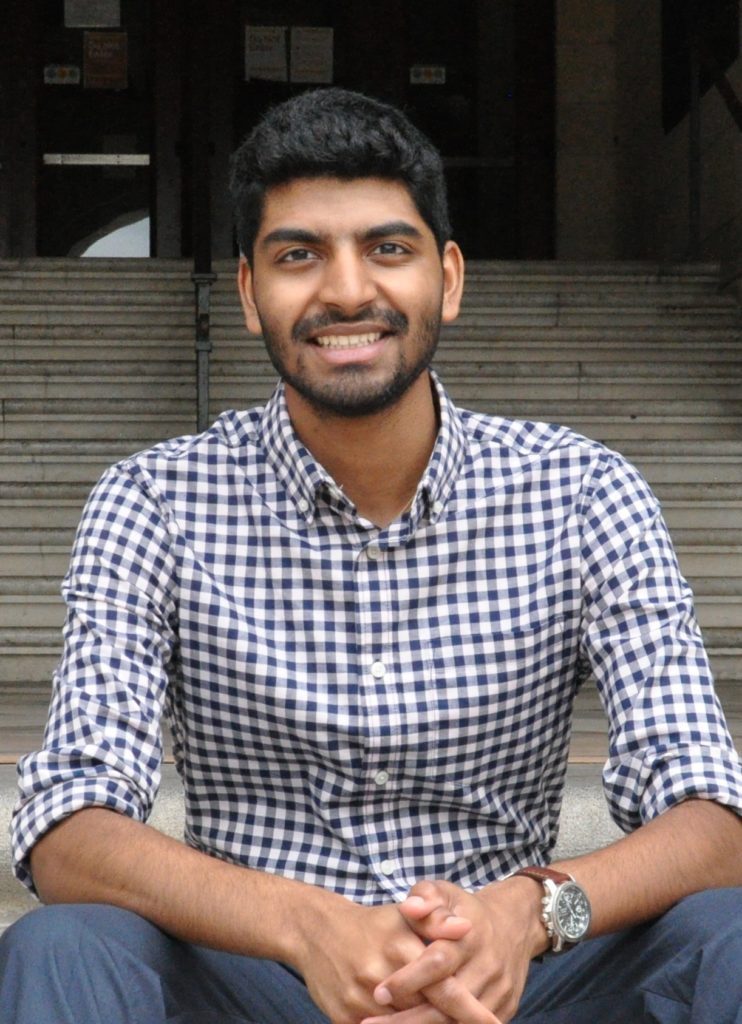
✍️ Original article by Abhinav Raghunathan, the creator of EAIDB who publishes content related to ethical ML / AI from both theoretical and practical perspectives.
This article is a part of our Ethical AI Startups series that focuses on the landscape of companies attempting to solve various aspects of building ethical, safe, and inclusive AI systems.
In our last article, we talked about the “ModelOps, Monitoring, and Explainability” category. We highlighted the idea that many of these companies are attempting to expand horizontally to compensate for a minimal demand space. For example, we mentioned that 2021.AI, which started in the MLOps space, has added dimensions of AI governance, risk, and compliance (GRC) to its offerings.
Another class of companies (EAIDB’s “Targeted Solutions and Technologies” category) solves the demand problem another way. Specifically, these companies hyperfocus on a single vertical and build through entire use cases to cover every angle that a customer might need. We call these companies specialists. Examples of these include FairPlay AI (fair lending), Pave (fair wage benchmarking), and Zelros (fair insurance recommendation engine).
This category also contains some startups that have created general frameworks or technologies that are meant to be built upon or integrated into various ways for different use cases. Examples include ethical facial recognition technology like AlgoFace or different kinds of learning or artificial intelligence technology (e.g., CausaLens for causal AI, Integrate AI for federated AI, etc.).
Due to the sheer number of verticals in industries like fintech, healthtech, hiretech, etc., this category tends to be the most populous in EAIDB.
Growth of the number of companies in the Targeted AI Solutions and Technologies category.
Trends
In the responsible AI segment, funding is skewed towards solutions targeting specific verticals.
In the other categories of EAIDB, we see a more significant number of companies focusing on horizontal game plans as a method of diversifying customer acquisition. We call these companies generalists. For example, many companies begin in MLOps but expand to include aspects of GRC once it is understood that demand for responsible MLOps is somewhat limited in Europe and the United States. The idea is to attract potential customers from MLOps and GRC use cases.
On the opposite side of the spectrum are the “Targeted AI Solutions and Technology” companies (specialists). Most have focused on a particular set of use cases and emphasize the customer journey through those use cases. While there is no distinct advantage to one approach over the other (both have produced several high-performance startups), specialists have historically enjoyed higher funding rates. About 92% of the startups in this category have raised funding of some kind, as opposed to 77% and 78% for our “MLOps, Monitoring, and Explainability” and “Data for AI” categories, respectively.
While fund rates are not generally indicative of startup success (especially in such a nascent industry), they provide minimal validation of a business model/approach. Put simply, our theory is that investment firms find it easier to understand the value proposition of specialists because they focus on a few “types” of customers and run use cases very deep.
Funding rates of companies in this category.
For example, consider a lending institution looking to train a fair lending algorithm. If the institution wants speed and doesn’t have a more nuanced use case, a generalist like Arthur will allow data scientists to input data and output a fair model. However, suppose the institution wants to identify the shortcomings of an existing model or do different types of analysis that a generalist like Arthur may not be able to fulfill. In that case, they go to a specialist like FairPlay AI.
The hiretech boom is over, but there is now much room for other industries.
Fairness and responsible AI received ample publicity in the mid-2010s as the importance of diversity in hiring began appearing in the headlines. Companies came in droves to solve the problem, with some cementing their place in the market, even to this day (e.g., Pave, Eightfold AI). In recent times, there has been an ongoing shift away from a focus on hiretech and towards other heavy industries like fintech, insuretech, healthtech, etc.
Representation of hiretech vs. other industries in this category.
In hiretech, the waves of responsible AI pushes by startups and the media are finally paying dividends in the form of changing legislation (e.g., NYC’s hiring bias labor law), but the problem with the space is that there is a dearth of real verticals within hiretech that startups can operate in. For example, with fair hiring, there are only a few focuses that have emerged: fair interviewing / candidate selection, fair salary benchmarking, and diversity measurement, to name a few. Compare this with fintech, which has verticals like lending fairness (in mortgage and personal markets, which are pretty different!), marketing fairness (as defined recently by the CFPB), redlining, and much more.
Some new technologies in this category will disrupt the way AI is performed.
In addition to innovation in verticals, the “Targeted AI Solutions and Technologies” also includes startups attempting to alter the ML techniques used in practice fundamentally. Some of these techniques are inherently more interpretable and easier to audit/explain than today’s ML gold standard.
While investigating each of these would take an entire article on their own, some examples of companies altering the ML dynamic include CausaLens (causal learning), UMNAI (neurosymbolic learning), and Integrate AI (federated learning). In general, the momentum is somewhat shifting away from traditional ML to some of these alternative methods due to their apparent advantages.
Barriers
Specialist startups have the additional hurdle of proving why a shift away from the incumbent is necessary.
The more ingrained companies get in a specific vertical, the more they start to face pushback from key players. To assuage all parties involved, they often need to prove that their product yields a significant advantage over the incumbent while being more ethical and responsible. The best performers are all able to consistently demonstrate this with concrete results.
For example, consider the fair lending startup FairPlay AI. To prove their value, they not only improve fairness in lending algorithms but also help discover new originations in underrepresented classes that boost the overall profitability of the business. More generally, they have robust technology to enable responsible AI but emphasize that they can provide this at a gain to the overall business. One without the other is not enough to encourage a switch away from an incumbent. This is a non-trivial problem that any specialist startup must solve.
Paradigm shifts are prolonged and require years to gain momentum.
This is somewhat expected from “disruptors” to a methodology of AI/ML development that is so ubiquitous. From universities to startups to enterprises, traditional methods reign supreme. However, the advantages of some of the newer systems of learning are tangible, clear, and impactful. Federated learning (spurred by Integrate AI and others) circumvents the high cost of moving data and adds guarantees to privacy that traditional ML has no hope of replicating. Causal AI (spurred by CausaLens) brings some level of cause-and-effect that today’s gold standard fundamentally lacks. Neurosymbolic AI (spurred by UMNAI) is on an even higher level and provides a strictly better method of performing statistical inference and machine learning.
The problem with startups like these is that it takes years for the AI community to switch from traditional systems. Of the three companies, CausaLens is the oldest (founded in 2017). Today’s AI climate is relatively warm to causal AI, and adopters of the technology span insurers, investment managers, and health care providers. Even so, earlier this year, Gartner published their Hype Cycle analysis and listed Causal AI as reaching its peak adoption in two to five years. Startups trying to bring more responsible alternatives via a paradigm shift will have difficulty staying afloat until the adoption is reached.
Garner’s Hype Cycle for 2022.
Outlook
The gradual shift away from generalists towards specialists will increase in speed.
The existing market for ethical/responsible AI is such that it’s hard for a potential customer to get a specific answer to the question “why do I need this product?” from generalists because their client bases are so heterogeneous. Recently, there has been a dichotomy in the responsible AI market where companies are choosing sides. Some choose to focus solely on one customer segment and adapt their products to fit all use cases the customer may require. Others embrace the generalist approach and build products that handle the most troublesome parts of a more comprehensive array of use cases. However, the number of companies pivoting to specialism is increasing as the market matures – responsible AI is, after all, highly domain-dependent. The leaders in generalism (Fiddler, Arthur, Credo) will remain at the top due to their competitive advantages, but others are likely to pivot.
There are some domains in which being a generalist is the norm and being a generalist with an upside is even better. Facial detection, for example, is one such domain. Many startups create general facial detection software for any application (facial detection for security, AR / VR, etc.). AlgoFace is an exciting example of a company that has focused wholeheartedly on general facial detection with the added upside of a complete and utter focus on ethical sourcing, training, and implementation. In this case, the generalist has significant advantages over any other incumbents, even if the incumbents are specialists.
Alternative methods to traditional AI / ML are poised to disrupt today’s standards.
While it is too early in the lifecycle of these innovations, there is undoubtedly a lot of promise. Traditional AI has many problems ranging from needing data to be centralized to privacy concerns to interpretability/explainability issues. The only solution to most of these is a paradigm shift. In a future article, we’ll talk about each of these in turn and focus on some companies creating entirely new ways of machine learning as solutions to them.