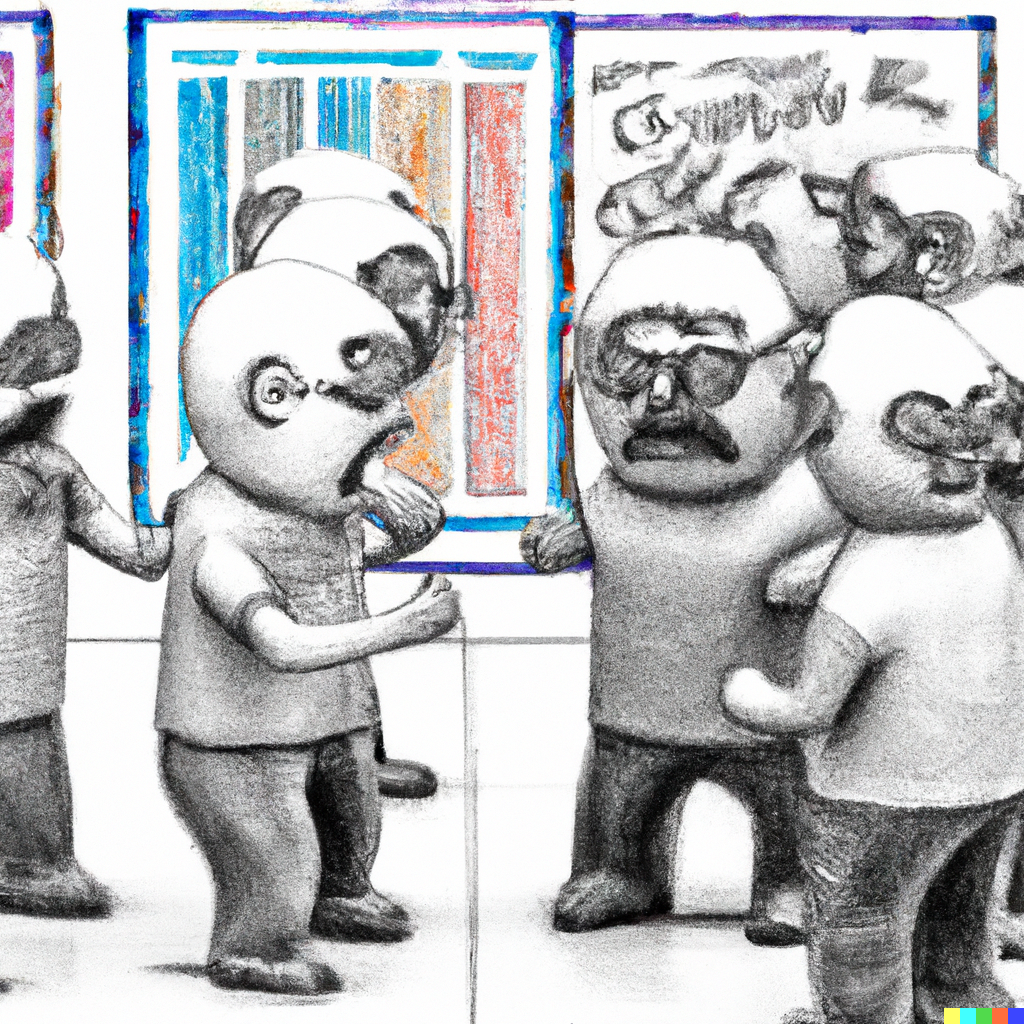
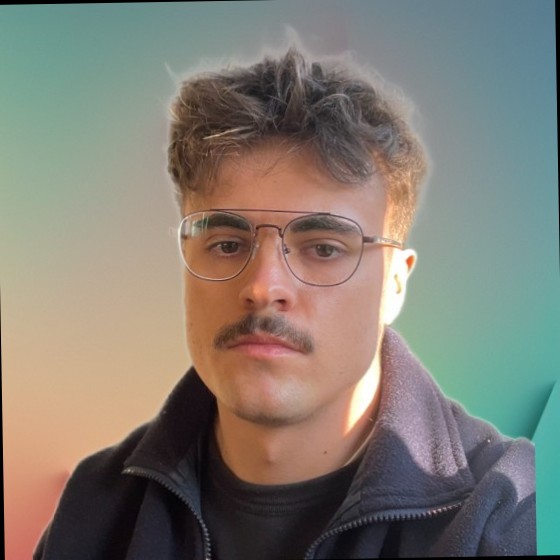
✍️ Column by Harry Law, a researcher at the Leverhulme Centre for the Future of Intelligence, University of Cambridge
Overview: Cultural battle lines are being drawn in the AI industry. Recently, California Democrat Representative Anna Eshoo sent a letter to the White House National Security Advisor and Office of Science and Technology Policy that criticized a highly popular AI model developed by Stablity.AI, one of a handful of new labs focused on the development of text-to-image systems. Unlike other popular text-to-image systems, however, Stability.AI is both freely available and provides minimal controls over the types of outputs a user chooses to generate––including violent and sexual imagery.
Eshoo describes models like Stable Diffusion (the name of the system developed by Stability.AI) as “dual-use tools that can lead to real-world harms like the generation of child pornography, misinformation, and disinformation.” She called on the National Security Advisor and the Office of Science and Technology Policy to “address the release of unsafe AI models similar in kind to Stable Diffusion using any authorities and methods within your power, including export controls.”
This intervention comes after companies like Midjourney, Meta AI, and Stability.AI have allowed millions of people to access text-to-image models that can create highly realistic imagery. For better or worse, technology that was previously the reserve of researchers and engineers has made its way into the public sphere. The labs developing these systems have each taken a response to publication that sits on a spectrum of access ranging from the permissive to the restrictive: some gate access and apply controls to ensure safe usage, while others share research without restrictions and provide little oversight. OpenAI’s flagship release, DALL-E 2, boasts over a million users and was made freely available to the public following a beta test designed to improve safety features. The lab reported that lessons learned from deployment and subsequent improvements to its safety processes were both key reasons for wider availability, including more robust filters that reject generations of sexual and violent content as well as new detection and response techniques to prevent misuse.
Although OpenAI provided access via an API, Stability.AI offered access through an API on its DreamStudio platform while sharing the model’s weights, enabling people to build use cases across third-party applications such as Adobe Photoshop. While often positive, commentary from the rapidly growing pool of users of text-to-image applications has criticized the data collection practices of labs which involve scraping large volumes of artwork from the internet to power what critics describe as systems developed explicitly for commercial purposes. This vein of commentary rightly highlights the data that drives AI development by demonstrating that, far from freestanding examples of ‘intelligence,’ modern AI systems exist as a web of ideas, data sources, funders, researchers, organizations, technologies, and more. But while this commentary acknowledges the nature of AI as a sociotechnical assembly, it tends to overlook the role of users––and researchers––in refining systems after deployment.
AI researchers remain split on the most effective and ethical approaches to developing and deploying today’s AI systems. On one side, researchers eschew the benefits of open-source approaches; they want to iteratively deploy new technologies via APIs by using controls that prevent misuse by understanding user behavior. Conversely, some seek to take a more libertarian approach to AI development. Here, controls are light or absent, and model weights are released ostensibly to encourage scrutiny of the research and the speedy development of downstream applications.
Both groups are critical of the approach taken by the other. Regardless of the arguments, this debate continues to swirl while powerful AI has made its way into the hands of the public. Real safety concerns matter right now, but the norms surrounding the safe development and deployment of AI systems in the future remain up for grabs. More good-faith dialogue is needed to find the middle ground between polarized perspectives, especially as the sophistication of the systems––and their subsequent potential for harm––increases.
A third way might be to expand the sociotechnical assembly concept to include the people who use the systems––not just those who help build them. Too often, labs demark their position in sterile terms about governance, terms of service, and user policies that obscure the very human perspectives of the millions of people who now use today’s AI technologies daily. Yet while criticism has so far rightly focused on a failure to acknowledge the role of people (in this instance, artists) who have provided the data from which today’s AI draws its predictive power, there is little said by researchers about the person on the other side of the computer screen.