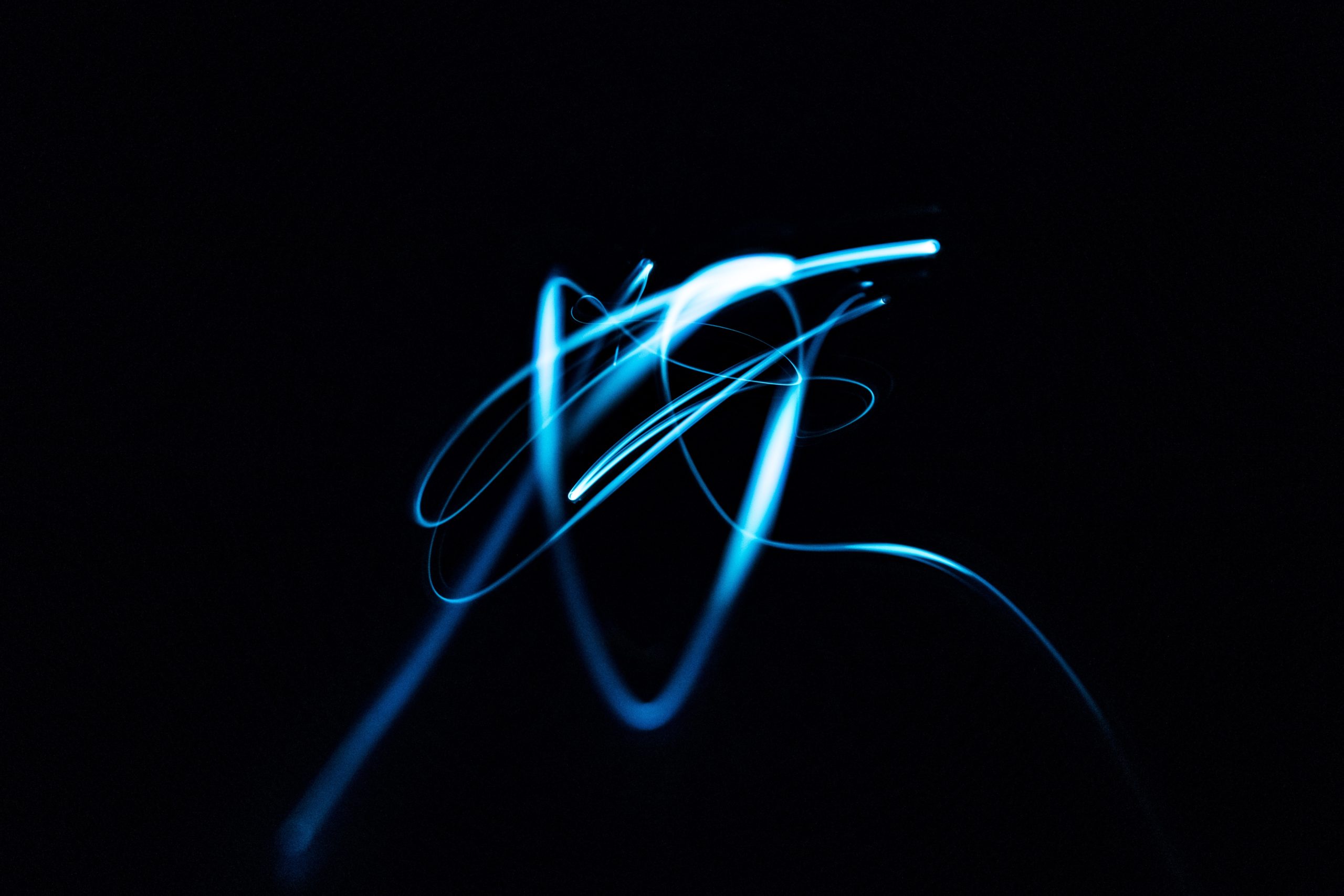
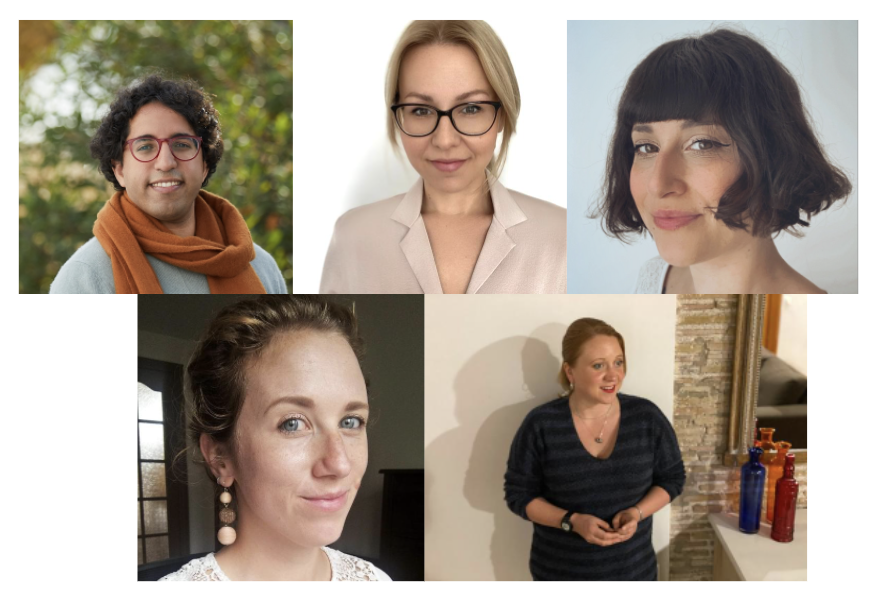
✍️ Original article by Yacine Jernite, Suzana Ilić, Giada Pistilli, Sasha Luccioni, and Margaret Mitchell from HuggingFace.
Yacine Jernite is a researcher at Hugging Face working on exploring the social and legal context of Machine Learning systems, particularly ML and NLP datasets.
Suzana Ilić is a Technical Program Manager at Hugging Face, co-leading the BigScience organization.
Giada Pistilli is an Ethicist at Hugging Face and a Philosophy Ph.D. candidate at Sorbonne University.
Sasha Luccioni is a Research Scientist at Hugging Face, studying the environmental and societal impacts of AI.
Margaret Mitchell is a researcher working on Ethical AI, currently focused on the ins and outs of ethics-informed AI development in tech.
This is part of the Social Context in LLM Research: the BigScience Approach series written by participants of several working groups of the BigScience workshop, a year-long research project focused on developing a multilingual Large Language Model in an open and collaborative fashion. In particular, this series focuses on the work done within the workshop on social, legal, and ethical aspects of LLMs through the lens of the project organization, data curation and governance, and model release strategy. For further information about the workshop and its outcomes, we encourage you to also follow:
- BigScience Blog
- BigScience Organization Twitter
- BigScience Model Training Twitter
- BigScience ACL Workshop
Introduction
A new category of AI models, Large Language Models (LLMs) is rapidly gaining traction in systems ranging from internet search to automatic translation to online discourse moderation. As a result, LLMs are likely to have sharply increasing importance in our societies. However, given the growing cost of training ever-larger models on ever more data, the development of this technology happens primarily in large private labs that seldom share complete details of the specifics. This status quo excludes most of the direct and indirect stakeholders whose lives will be affected by these new systems, putting regulators and society in a position where they always have to respond to harms after they have already been created in real-world situations.
To help forestall those harms and guide the practices of LLM development toward more accountability to these stakeholders, we need this research to also happen in a setting where their input and expertise can come into play much earlier in the design process; so they can help shape the values and priorities of the entire research project, collaboratively decide what data (and what views of the world and varieties of language) it uses, what evaluations should be run to assess the models’ appropriateness for specific uses, and how to govern both the data and trained model to protect the data and algorithm subjects’ rights.
This blog post is the first in a series outlining the efforts of the participants of a collaborative research project regrouping over 1000 participants from 60 countries toward making these aspects of the LLM lifecycle more inclusive. Specifically, it provides an overview of the ethical, legal, and governance work that happened throughout the workshop on the way to training and releasing a multilingual Large Language Model, and will be followed by further installments focusing on the project’s ethical and legal grounding, its approach to data governance and representativeness, and to its model governance and release strategy.
The BigScience Workshop
As a community-driven open science project supported by public computation infrastructure (the French Jean Zay supercomputer), BigScience proposes a different approach that brings together hundreds of multidisciplinary researchers around the world to collaborate not only on the training of a new model but also on understanding its various social and legal contexts and developing governance mechanisms that are informed by their specific technical workings. Initiated by Hugging Face in collaboration with the Institute for Development and Resources in Intensive Scientific Computing (IDRIS) and Grand Équipement National de Calcul Intensif (GENCI) in January 2021, the BigScience “Summer of Large Language Models 2021” workshop grew into a community-driven effort bringing together over 1000 participants from 60 countries to study the technical and social aspects of Large Language Models across 30 working groups, each with a different focus.
The workshop brings together researchers with a variety of academic backgrounds and experiences to work on questions that explicitly address the technology’s place and role in society. This unique approach to collaborative research allows participants with ethical, legal, and technical expertise to not only learn from each other but also to identify how recent developments and scholarship in each of these areas interact in practice to determine what further research is needed. The present series of blog posts describes how this multidisciplinary work occurred in three domains of particular importance to our goal of enabling broader participation in the development of LLMs, outline the artifacts produced to serve this purpose, and proposes directions for follow-up works
Aspects and Domains of the Broader LLM Research Context
Research projects of a scale with BigScience necessarily consist of a complex network of interactions, research questions, intermediary goals, artifacts and by-products, complementary skills, assumptions, and values. This complexity gains yet another dimension when considering the outputs of the endeavor (including the trained LLM, named BLOOM) not just as isolated artifacts but rather as situated in their broader context within the society that supports the research.
In order to better understand these interactions and shape our efforts to promote more inclusive research, we analyze three main aspects of the BigScience workshop through the lens of three complementary domains that are particularly relevant to understanding their broader context and impact on society: namely, we describe the participants’ work on questions relating to the project organization, the data selection and management, and future uses of the trained model. For each of these aspects, we outline research done on their ethical and legal dimensions, and the governance processes the participants developed.
Ethics, Law, and Governance: Complementary Domains
Law, philosophy, and political and social sciences provide complementary approaches to assigning a place for LLMs within society. Legal frameworks help characterize the normative aspects of good governance of LLMs, while ethics defines what actions are desirable and morally accepted by communities and the people affected. When we decide to operationalize common moral values to inscribe them in an ethical charter, philosophy comes into play. If we consider morality a fundamental exercise to be carried out, which even precedes the formalization of law, a collaboration between the two subjects becomes necessary. The synthesis of these two aspects leads us to formalize defined governance frameworks that manage to identify specific details to make it operable.
In this framework, we define ethics as the philosophical branch that investigates the morality of human agents in moments of deliberation. In our scientific domain, ethics guides human action at sensitive moments such as technical choices. In comparison, we consider law as the legislative framework capable of guiding collective choices at the societal level. Whereas ethics is adopted voluntarily, held only to moral obligations, non-compliance with law implies legal sanctions. Then, governance provides an organizational framework and policy guidance for choices made on moral or legal grounds. Governance is concerned with the management, control, and proper adherence to ex-ante ethical and legal standards. In our scientific domain, this amounts to operationalizing the ethical and legal reflections that may emerge from our discussions.
Following this distinction, we thus start by answering the following questions when describing our work on specific aspects of the workshop in the rest of this series:
- People. Who will be the people most directly affected by our efforts in this aspect of the workshop? Who are we thinking of as direct or indirect stakeholders?
- Ethical focus. How do we arrive at a value statement to guide our choices in this aspect of the workshop?
- Legal focus. What regulations currently exist that are relevant to this aspect of the workshop? How can our work in turn inform new regulations?
- Governance. How do we organize our efforts on this aspect of the workshop to account for both the identified moral values and legal context?
Project, Data, and Model Questions: Deeper Dives
We use the above questions to contextualize our efforts in three particular aspects of the workshop, each is the focus of another blog post in this series. The following outline provides an overview of the work done on each of these aspects and a selection of the artifacts that were produced along the way, and the linked posts provide more detail into what the work consisted of and how we approached the interplay of the ethical, legal, and governance questions:
- Project Ethical and Legal Grounding: identifying a set of driving values and engaging with existing and emerging relevant regulations around the world are both critical aspects of ensuring that the project makes progress toward its stated objectives of principled governance of inclusivity and responsibility. The first linked blog post describes our efforts to these ends, and their concrete outcomes including:
- a collaboratively built ethical charter emphasizing value pluralism to help guide a project of this scale,
- a multi-jurisdiction legal playbook to support ML and NLP researchers working with human-centric data around the world.
- Data Governance and Representation: not only are LLMs a data-driven technology, but they also deal with human-centric data; i.e. data created by or about human subjects whose rights and interests have bearing on how it may and should be used. The second linked blog post outlines our efforts to collect and use data in a way that respects these rights and acknowledges these interests, which led among other outcomes to devising:
- a new international data governance structure with supporting tools,
- a data agreement formalizing relationships between data owners and hosts,
- a novel language-expert-driven, bottom-up approach to collecting a catalog of geographically diverse data sources,
- a suite of language-specific curation tools developed with native speakers,
- an interactive dataset card to help navigate the sources represented in the training corpus.
- Model Governance and Responsible Use: the BigScience workshop culminates in the release of a 176B-parameter LLM. The model was designed primarily to support further research and we believe that stakeholders are best positioned to decide what that research should look like and how to use the model. We designed a release strategy that aims to strike a balance between enabling a variety of actors to make the best use of the trained model and acknowledging its limitations and potential harms through:
- a new Responsible AI License (RAIL) for the model release which includes behavioral use-case restrictions to require users to abstain from specific known harmful uses and uses that are likely to cause harm,
- work on model evaluations assessing the model’s performance on traditional NLP tasks and interrogating the biases represented in its trained weights,
- a thorough model card to inform users and help them scope appropriate and inappropriate uses.
Each of these aspects will be the focus of a deeper dive in each of the three next installments in this series, we refer the reader to these for further details and a more extensive list of the produced outcomes and research artifacts.
Conclusion
With its over 1000 participants spread around the world and multidisciplinary approach to addressing questions at the intersection of technology and society, the BigScience Workshop has provided a unique opportunity to help make research into newer language technology more inclusive and grounded in societal considerations.
Rather than attempt to fully solve the complex questions that arose in this context within the limited time scope of the project, we strove instead for making meaningful progress so as to support future efforts grappling with similar issues, for helping surface and frame research directions that will need further work in similar endeavors, and for creating tools and processes that will help an even greater diversity of participants to leverage our work.
While our effort is unique in its approach and in enabling a new scale of collaboration on recent language technology, we also want to take a moment to acknowledge its many inspirations here. Just to name a few, we recall the experiment on CERN’s Large Hadron Collider, which managed to bring together more than 10000 scientists and 100 universities and laboratories, OpenML for fostering open collaboration among machine learning researchers, the grassroots collective EleutherAI’s work including the GPT-J model and The Pile dataset, and grassroots NLP communities such as Masakhane supporting research on African languages for Africa.