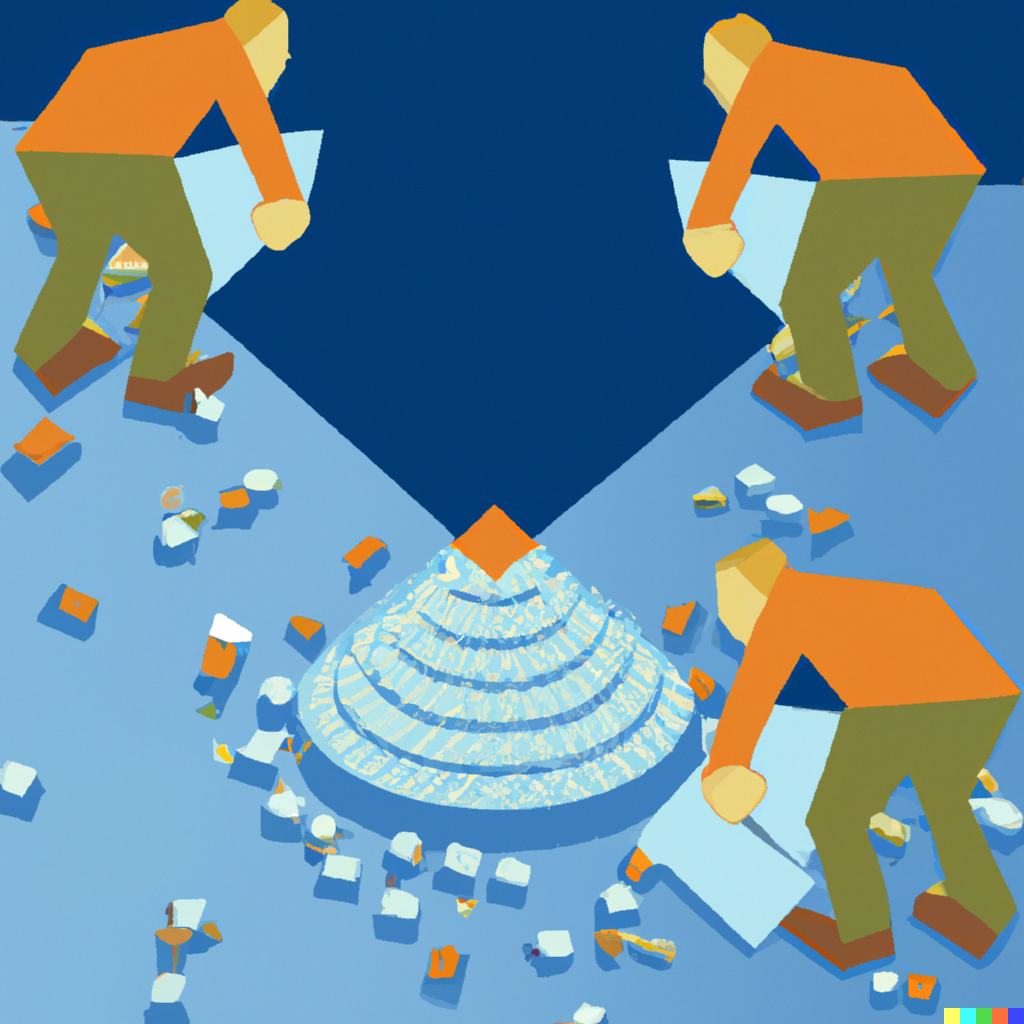
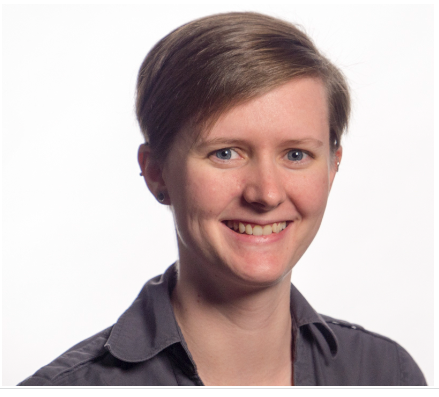
🔬 Research Summary by Maggie Delano, an Assistant Professor of Engineering at Swarthmore College whose research focuses on developing medical devices for chronic diseases and inclusive engineering design.
[Original paper by Kendra Albert and Maggie Delano]
Overview: Considering sex and gender in medical machine learning research may help improve health outcomes, especially for underrepresented groups such as transgender people. However, medical machine learning research tends to make incorrect assumptions about sex and gender that limit model performance. In this paper, we provide an overview of the use of sex and gender in machine learning models, discuss the limitations of current research through a case study on predicting HIV risk, and provide recommendations for how future research can do better by incorporating richer representations of sex and gender.
Introduction
False assumptions about sex, gender, and their intersections are deeply embedded in society, and medical machine learning is no exception. We reviewed over two dozen medical machine learning papers in our recent paper. We found that sex and gender are almost universally considered binary variables, with frequent substitution of sex for gender and vice versa. Not only are sex and gender not binary, but their use in machine learning is indirect: they serve as proxies for variables that may have more direct clinical relevance, such as hormone status, diet, or genetics. However, without a better understanding of what sex and gender are proxies for, models trained using these data will perform worse for anyone who deviates from the average. We encourage any researchers developing or using models incorporating sex and/or gender to educate themselves about sex/gender, work in teams with a range of competencies, and focus on untangling the variables most predictive for their work.
Key Insights
Sex Is Not A Binary Variable
While the binary sex variable in a machine learning model might seem straightforward, it is more complex. Sex acts as a proxy for a variety of biological and sociocultural variables. This includes not only biological attributes commonly associated with a given sex, like hormone status or chromosomes, but also gendered practices, such as diet or exercise patterns that imprint on the body. With sex as a “stand-in” for so many different variables, the predictive power of a machine learning model is reduced, especially for individuals that deviate from the average individual in the training set. This exacerbates existing issues with model transferability and means performance will be especially worse for historically marginalized populations such as transgender people.
As transgender people have become more accepted in society, there’s been increasing recognition that sex and gender are distinct, though entwined, concepts. However, this acceptance has not yet translated to improvements in how sex and gender are used in medical machine learning models. We reviewed over two dozen medical machine learning papers published since 2016 and found that these papers overwhelmingly continue to use binary sex variables. In some cases, authors use the word “gender” to refer to this variable, even though the labels are “M” and “F.” This is a phenomenon we call sex/gender slippage, where the presumed concordance of sex and gender leads to the frequent substitution for one variable with the other.
Sex Confusion
The trouble with relying on a binary sex variable is apparent when one considers the confusion that can result when using it in a medical context. Many transgender people choose to update their identifying documents to match their gender. This is not only affirming but may also be necessary for safety reasons. When a transgender person receives medical care, it may not be clear from their medical records whether the listed sex is the sex they were assigned at birth or a sex that reflects their current gender identity. This is what we call “sex confusion.” EHRs introduced a gender identity field to try to address this issue. However, our research suggests that in many cases, the gender identity field is used more for avoiding misgendering patients, not necessarily to consider it for delivering medical care. Many clinicians consider sex assigned at birth more important than gender identity, even when it may not be relevant for care in a given context. We call this “sex obsession.”
Doing Better
When machine learning models use sex and/or gender variables, they must reconcile not only with sex confusion (knowing whether something is sex assigned at birth or not) but also with sex obsession (the idea that what really “matters” is sex assigned at birth). We advocate for researchers to approach model development with a mindset of designing from the margins by focusing on increasing data richness.
A design from the margins approach centers on people historically decentered. One way to do this in a machine learning context is data richness. Rather than only using a binary variable for sex or gender, consider using a variety of variables that might be relevant to the research question and include additional information such as disease onset, phenotype, etc. By focusing on data richness, researchers can build more predictive models and have a deeper understanding of which variables improve model performance, thereby potentially improving performance for groups not served by a binary approach. This improves outcomes for underrepresented groups and everyone, as there is a more foundational understanding of the predictive variables.
Between the lines
Funding institutions and journals have called for increased consideration of sex and gender in clinical research. However, without avoiding the pitfalls we describe in our paper, this research may have a limited impact, especially for the marginalized groups this consideration is intended to help. Designing from the margins and focusing on data richness take time but can positively impact everyone.
Moving beyond binary sex variables requires a mindset shift and new clinical research and research methods. Researchers must be willing to take things slow and consider which variables to incorporate into their models. Unfortunately, which variables to include might not be clear as medical research has historically held many of the same false assumptions that medical machine learning research has. More support for basic research will be needed to help researchers investigate further rather than falling back on existing approaches. Funding institutions and journals will need to weigh the costs of continuing to support work that relies on binary sex variables and how to support the development of new models and datasets while recognizing that all research has limitations.