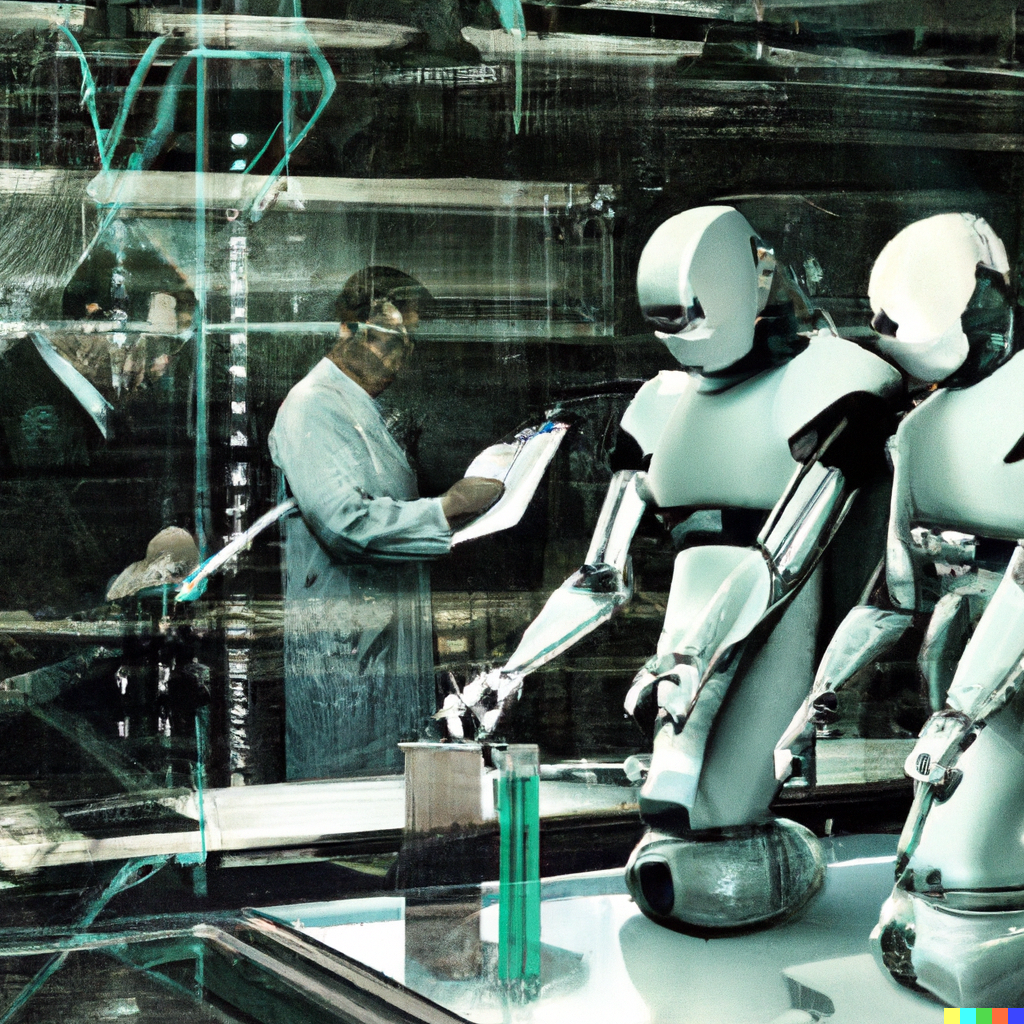
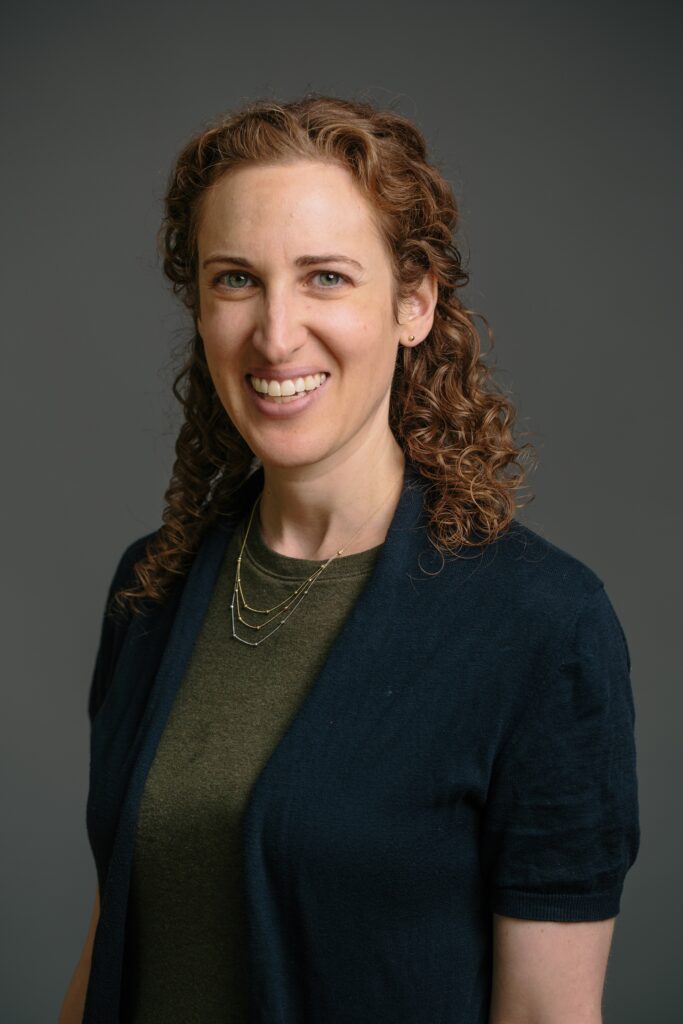
🔬 Research Summary by Meredith Ringel Morris, Director of Human-AI Interaction Research at Google DeepMind; she is also an Affiliate Professor at the University of Washington, and is an ACM Fellow and member of the ACM SIGCHI Academy.
[Original paper by Meredith Ringel Morris]
Overview: There is potential for Generative AI to have a substantive impact on the methods and pace of discovery for a range of scientific disciplines. We interviewed twenty scientists from a range of fields (including the physical, life, and social sciences) to gain insight into whether or how Generative AI technologies might add value to the practice of their respective disciplines, including not only ways in which AI might accelerate scientific discovery (i.e., research) but also other aspects of their profession, including the education of future scholars and the communication of scientific findings.
Introduction
How will Generative AI transform the practice of scientific discovery and education? We interviewed 20 life, physical, and social science researchers to gain insight into this topic.
Our participants foresaw the potential for both positive and negative impacts of Generative AI. Potential benefits include novel educational paradigms, AI-supported literature review including cross-disciplinary connections and insights, tools to accelerate dataset creation and cleaning, AI-assisted coding to accelerate various aspects of experimentation and analysis, novel AI-assisted methodologies for data collection and experimental design, and AI-enhanced writing and peer-review systems.
Concerns included AI-aided cheating and lost opportunities for critical reflection, the introduction of bias through the use of AI, factuality, and trustworthiness of AI tools, the proliferation of publication spam, fake data, and scientific misinformation. This work contributes findings that can support more human-centered and responsible development of AI tools for the sciences by representing the perspectives of experts with deep knowledge of the practice of various disciplines within the physical, life, and social sciences.
Key Insights
We interviewed twenty scientists (seven female, thirteen male), all with Ph.D. degrees in a field relevant to their discipline. We included participants with backgrounds in the physical sciences (e.g., physics, geology, chemistry), the life sciences (e.g., immunology, bioinformatics, ecology), and the social sciences (e.g., sociology, anthropology, behavioral economics).
Our interviewees agreed that advances in Generative AI were likely to change many aspects of both undergraduate- and graduate-level education in the sciences. Many of these changes are potentially positive, such as innovation in instructional methods and the lowering of barriers for English language learners. On the other hand, many participants also expressed concerns about the impacts of AI-assisted cheating on evaluation methods and critical thinking.
Data are core to many aspects of the scientific method. Data may represent observations; analyzing these observations can support the generation of hypotheses. Experiments designed to test hypotheses can, in turn, generate data, analysis of which can support drawing conclusions and iterating on predictions, experiments, etc. Our participants saw many opportunities for Generative AI technologies to support data acquisition, preparation, and analysis. However, they had concerns about introducing errors into data sets, removing human reflection from data analysis, and applying modern ML methods to “small data” scientific fields.
Keeping abreast of the scientific literature in one’s field is a key part of scientists’ jobs. This is important for myriad reasons, including maintaining fresh, professional skills through an ongoing awareness of key trends and discoveries, finding inspiration for future research ideas, identifying potential collaborators, and conducting literature reviews before embarking on new research projects to validate the novelty of a contribution, build appropriately on prior knowledge (such as by using comparable methods and data to past experiments for replicability and comparability), and appropriately citing related work as part of the scholarly publication process. Generative AI, particularly language models, is poised to alter many aspects of the literature review process substantially.
Generative AI systems such as OpenAI’s Codex and GitHub’s Copilot, which debuted in 2021, are specifically designed to complement (or potentially replace) human programmers by producing software snippets that can function as-is or that can be edited by a human developer to accelerate the coding process. In addition to coding-specific AI tools, many general-purpose LLMs can produce code as an emergent behavior since code samples are present in many internet forums used for training models. Computation is increasingly a part of many fields of science; programming is necessary at various stages of scientific work, including collecting or cleaning data, running simulations or other experimental procedures, and running statistical analyses on experimental results. Most scientists are not formally trained in computer science or software development, and producing correct code (much less high-quality code that is efficient and reusable) is extremely time-consuming for this constituency. AI tools that can accelerate or automate coding via a natural language interface have a huge potential to accelerate scientific discovery.
Generative AI has the potential to directly accelerate the discovery of new scientific knowledge through improved experimental design, novel methods and models, and support for the interpretation of experimental results. Our participants reflected on how new AI models can support various aspects of scientific discovery and cautions to be wary of.
One of the biggest near-term opportunities for generative models is to support scientific communication, including writing scholarly articles, grant applications, and preparation of presentation materials such as slide decks. There is also an opportunity for AI tools to play the reviewer role, offering preliminary feedback that helps scholars fine-tune their arguments ahead of true peer review. LLM-assisted scientific writing also carries many risks, particularly the risk of generating publication spam and scientific misinformation.
The issue of trusting AI was core to the interviews; participants frequently shared concerns relating to trust (e.g., concerns about hallucinations and factuality, a need for citation back to sources) and were also asked at the close of the interview to specifically reflect on what would be necessary to trust the use of Generative AI in the sciences. Addressing these concerns (some technical, some social) are vital for developing AI that aligns with scientists’ and society’s values so as not to risk diminishing trust in science itself.
Between the lines
Our interviews revealed possible applications of new AI tools across a large swath of scientific practice, including education, data, literature reviews, coding, discovery, and communication. While there is great potential for new AI technologies to augment scientists’ capabilities and accelerate knowledge discovery and dissemination, there is also a need for caution and reflection to mitigate potential negative side effects of scientific AI tools and prevent intentional misappropriations of new technologies. Notably, our participants envisioned AI as a tool that would complement, enhance, or accelerate scientists’ abilities rather than something that would automate the practice of science. We are on the cusp of an AI revolution that will touch all aspects of society, including the methods and pacing of scientific discovery.