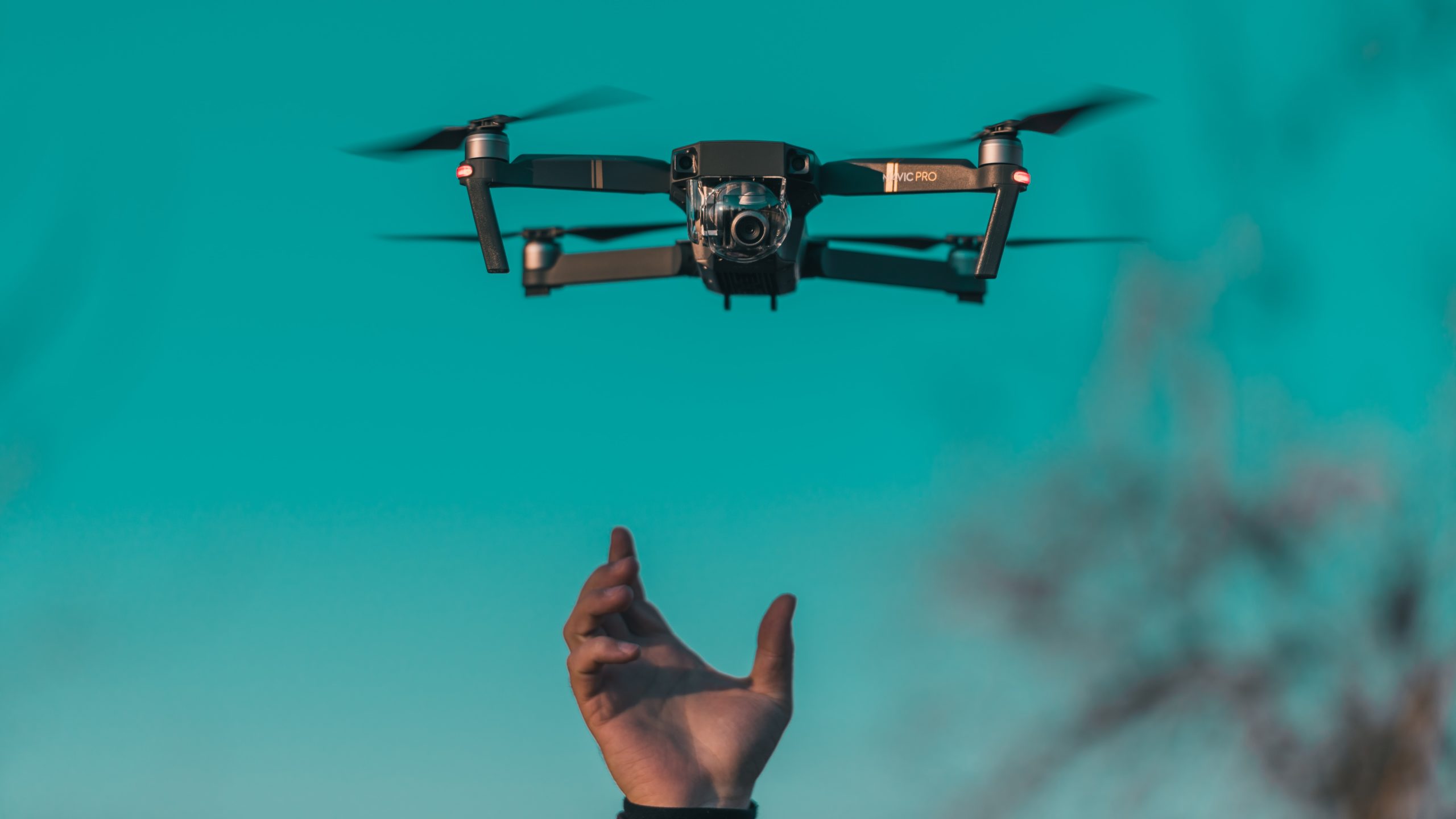
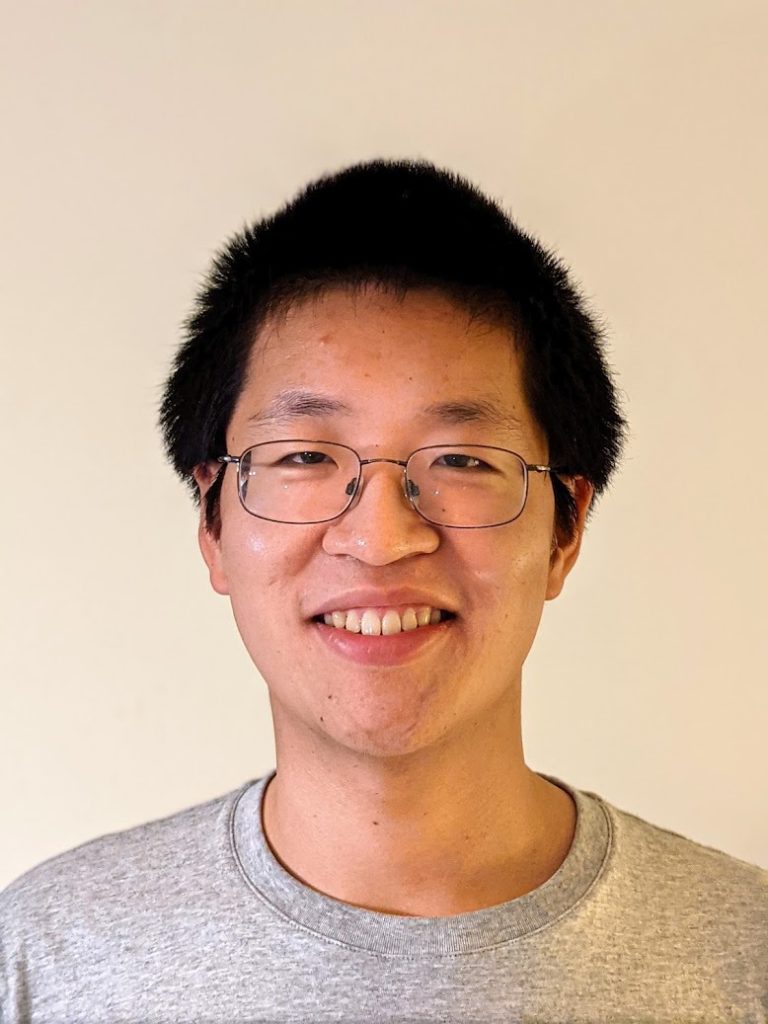
🔬 Research Summary by Geoffrey Ding, a graduate student at the Massachusetts Institute of Technology conducting research on advanced air mobility.
[Original paper by Geoffrey Ding, Alex Berke, Karthik Gopalakrishnan, Kwassi H. Degue, Hamsa Balakrishnan, Max Z. Li]
Overview: Drone package delivery systems may lead to loss of consumer privacy, given current safety regulations. We formalize and analyze these privacy concerns and propose strategies to mitigate these issues. We also examine possible trade-offs between privacy and delivery time.
Introduction
Many companies are promising rapid drone deliveries soon for goods ranging from take-out food to prescription medications to everyday home goods. To ensure safety, drones may be required to share their locations while in operation. For example, the US Federal Aviation Administration has codified such requirements in remote identification legislation; similar rules can be found in other regions. By tracking the location of a drone, an observer can deduce where a drone goes, connecting a vendor to its customer. Would you want everyone else to be able to find out how often you ordered take-out food—or perhaps something less innocuous?
Of course not. Nobody needs to know what you’re buying, especially not for profit-driven or privacy-invading purposes such as targeted advertisements, discrimination by insurers, or customer profiling. Fortunately, ideas from other domains can obfuscate what you ordered. We’ll look at some strategies that delivery companies might use to protect your privacy and see how that might—but doesn’t necessarily—require you to wait longer for your items.
Key Insights
Privacy risk
We use “privacy risk” to refer to the chance, on a scale from 0 (impossible) to 1 (certain), that some outside observer can guess what you ordered. For example, if a drone flies from a liquor store to your residence, it’s pretty clear that you ordered alcohol, so your privacy risk is 1. On the other hand, if a drone flies from a liquor store to a pharmacy before dropping off your item, there is uncertainty as to whether you got something from the liquor store or the pharmacy. Thus, your privacy risk has been lowered.
Mitigation strategies
There are a few ways a drone delivery company can reduce privacy risks for its customers. In our description of privacy risk, we introduced one—decoy vendors. By stopping at additional stores before or after the actual store of interest, there is ambiguity in where the order came from. Moreover, decoy vendors may be able to provide ancillary services such as drone recharging (and be compensated accordingly).
Now, imagine a drone needs to fulfill multiple orders. If the drone goes back and forth between vendor and customer, it is again easy to match up who got what. If the drone visits all vendors first and then all customers, however, anyone could have ordered anything, and privacy risk is decreased. We call this privacy via aggregation—specifically, aggregation of orders.
When considering aggregation, we must also keep in mind the capacity limit of the drone. If the drone can carry as many items as there are orders, then orders can be aggregated arbitrarily and in any sequence. At the other extreme, if the drone can only carry one item at a time, then it would seem like we are once again stuck with a privacy risk of 1. That is, any observer can link a customer with the corresponding vendor. But we have seen this problem before! The solution is to use decoy vendors. In between, when the drone can carry more than one—but not all—items, we find that there are two ways to improve privacy without decoy vendors: a drone with a larger capacity may be used, or more orders may be delivered. A drone with a larger capacity enables increased aggregation, while more orders permit more delivery route options that can lower privacy risk.
Geographical considerations
We must also consider the costs of using these tactics to preserve privacy. Putting aside financial considerations for now—it’s unclear whether there even is a market for this form of privacy—we examine how the desire for privacy may require a trade-off in delivery time. As it turns out, this trade-off is highly dependent on geography.
First, we provide a situation where we must choose between privacy and fast deliveries. Consider a setting where vendors and customers are randomly located. Then, if we wanted to maximize privacy, we would need to first visit all vendors before going to all customers. Even if a customer’s order is aboard the drone as the drone passes near the customer, the delivery cannot happen if the emphasis is on privacy above all else. In the case of minimizing delivery wait times for all customers, any consideration of privacy risk goes out the window, and no level of privacy can be guaranteed. Of course, there is some midpoint between the two extremes, but a trade-off must be made.
Conversely, it is possible to have a setting where we can have both privacy and a low (average) delivery time. Imagine several orders where all the vendors are clustered together, and all the customers are clustered together, but a significant distance separates the two clusters. Then, the way to maximize privacy, by collecting all orders and then delivering all orders, is identical to how to minimize average delivery time. This is because aggregation of all items enables the drone to make the trip across the aforementioned “significant distance” only once, eliminating the need for time-consuming trips back and forth.
Between the lines
Existing work on privacy considerations in drone operations tends to focus on privacy from the perspective of surveillance of uninvolved parties, for example, via onboard cameras. We look at privacy concerns for the customer instead. Because this is a novel view of privacy risk in the context of drone operations, there are many open directions for further work. For example, one could ask about longitudinal privacy risk: could someone figure out what you ordered based on tracking a drone’s path over many delivery orders? Or, one might wonder how delivery time requirements affect how easily a vendor-customer matching can be determined. Another interesting question would be the implications of a vendor delivering to multiple customers, a customer receiving orders from multiple vendors or both. All in all, our work with customer privacy risks in drone package delivery systems highlights the consequences—intended or not, foreseen or not—of new technologies and their associated regulations, particularly for those that impact a wide range of consumers.