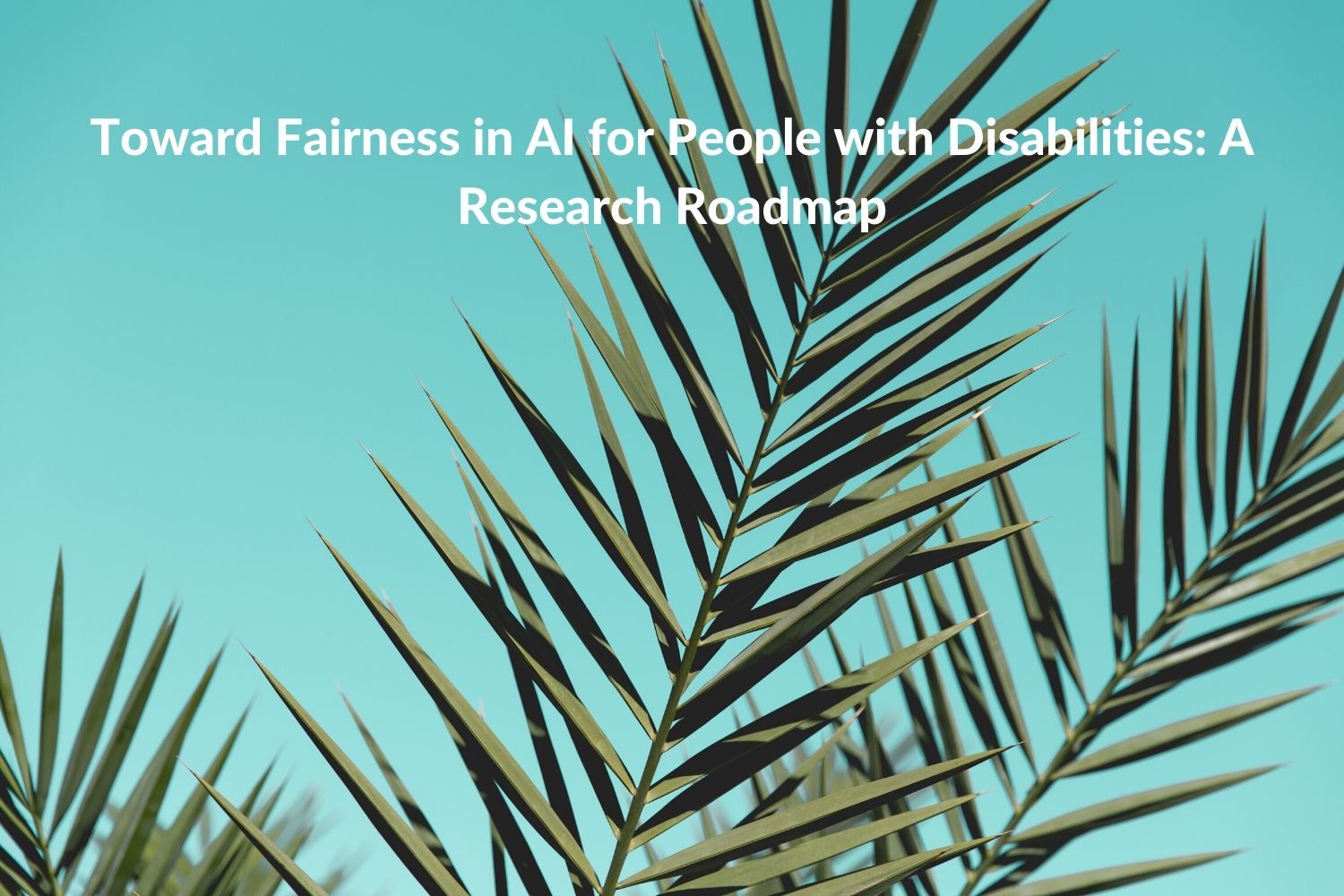
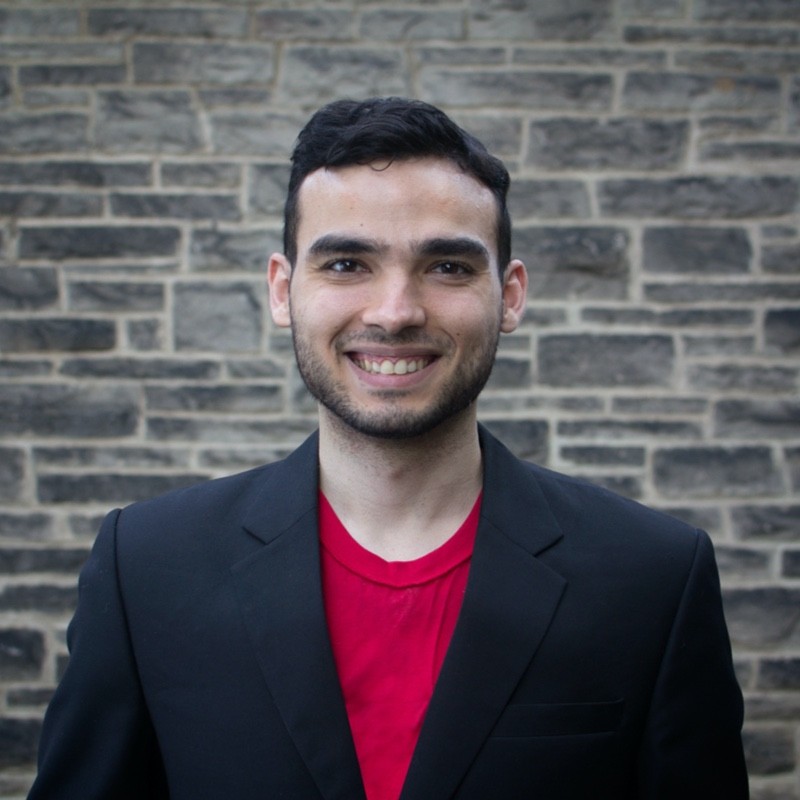
Summary contributed by Pablo Nazé, Sr Business Manager of Responsible AI at Fairly AI. MBA grad from Rotman.
*Author & link to original paper at the bottom.
In this position paper, the authors identify potential areas where Artificial Intelligence (AI) may impact people with disabilities (PWD). Although AI can be extremely beneficial to these populations (the paper provides several examples of such benefits), there is a risk of these systems not working properly for PWD or even discriminating against them. This paper is an effort towards identifying how inclusion issues for PWD may impact AI, which is only a part of the authors’ broader research agenda.
The authors note that this systematic analysis of interactions between PWD and AI is not an endorsement of any system, and that there may exist an ethical debate of whether some categories of AI should be built. It’s important to note that this analysis is a starting point towards this theme, and may not be exhaustive.
The paper is then divided into considerations across many AI functionalities and possible risks when used by PWD. The authors covered computer vision (identification of patterns in still or video camera inputs), speech systems (systems that recognize the content or properties of speech or generate it from diverse inputs), text processing (understanding text data and its context), integrative AI (complex systems based on multiple models), and other AI techniques.
Computer Vision – Face Recognition: The authors hypothesize that such systems may not work well for people “with differences in facial features and expressions if they were not considered when gathering training data and evaluating models”. For example, people with Down syndrom, achondroplasia, or cleft/lip palate. Systems may also malfunction for blind people, who may not show their faces at an expected angle or who may use dark glasses. Finally, emotion and expression processing algorithms may malfunction for someone with autism, Williams syndrom, who suffered a stroke, Parkinson’s disease or “or other conditions that restrict facial movements”.
Computer Vision – Body Recognition: “Body recognition systems may not work well for PWD char- acterized by body shape, posture, or mobility differences”. Among some examples, the authors point to people who have amputated limbs or someone who experiences tremors or spastic motion. Regarding people with differences in movement, systems may malfunction for “people with posture differences such as due to cerebral palsy, Parkinson’s disease, advanced age, or who use wheelchairs”. The paper cites an Uber self-driving car accident, in which the car hit someone walking a bicycle.
Computer Vision – Object, Scene, and Text Recognition: Many of these systems are trained in high quality pictures, usually taken by sighted people. It’s to expect that these systems may malfunction while trying to detect objects, scenes, and texts from images taken by a blind user, or someone who has tremors or motor disabilities.
Speech Systems – Speech Recognition: Automatic Speech Recognition (ASR) may not work well for “people with atypical speech”. It’s known that such systems works better for men than women, while malfunctioning for people of very advanced ages or with stronger accents. The authors point to speech disabilities, such as dysarthria, that need to be taken into consideration for a fair construction of those systems. Further, ASR locks out people who cannot speak at all.
Speech Systems – Speech Generation: Systems may include Text To Speech (TTS) technologies. These systems may be challenging for people with cognitive or intellectual disabilities, who may require slower speech rates.
Speech Systems – Speaker Analysis: These systems can identify speakers or make inferences about the speaker’s demographic characteristics, potentially being used for biometric authentication. These systems may malfunction for people with disabilities that impact the sound of their speech. Further, Analysis trying to infer sentiments may fall short for austitic people.
Text Processing – Text Analysis: Some systems, such as spelling correction and query rewriting tools, may not handle dislexyc spelling. Moreover, since autistic people express emotion differently, systems that infern sentiments from text may also fall short for this population.
Integrative AI – Information Retrieval (IR): These are complex systems, such as the ones that power web search engines. It is possible that IR amplifies existing bias against PWD. For example, search results can return stereotypical content for PWD, while targeted-advertising may eventually exclude PWD from products or even employment opportunities.
Integrative AI – Conversational Agents: These agents are present in various services, such as healthcare and customer service. These systems may amplify existing bias in their results, if not trained properly. Further, people with cognitive disabilities may encounter poor experience while utilizing these services. It is important that these systems can adapt to the users’ needs, such as reduced vocabulary or expression in multiple media.
Other AI Techniques: For example, outlier detection. These systems usually flag outlier behaviour as negative, tied to punitive action. For example, input legitimacy (use of CAPCTHAs or other mechanisms to separate humans from bots), may not work well for people with atypical performance timing, such as someone with motor disabilities or visual impairments.
The authors exposed in this opinion paper ways in which AI can negatively affect PWD, which usually reflects in a worse quality of service, underrepresentation, or stereotyping for these populations. Some of the cases mentioned in the paper are hypothesis, while some are backed up by evidence. The authors also propose a broader research roadmap for AI fairness regarding PWD, including testing the hypotheses presented, building representative datasets, and innovative new AI techniques “to address any shortcomings of status quo methods with respect to PWD”.
Original paper by Anhong Guo, Ece Kamar, Jennifer Wortman Vaughan, Hanna Wallach, Meredith Ringel Morris: https://arxiv.org/abs/1907.02227