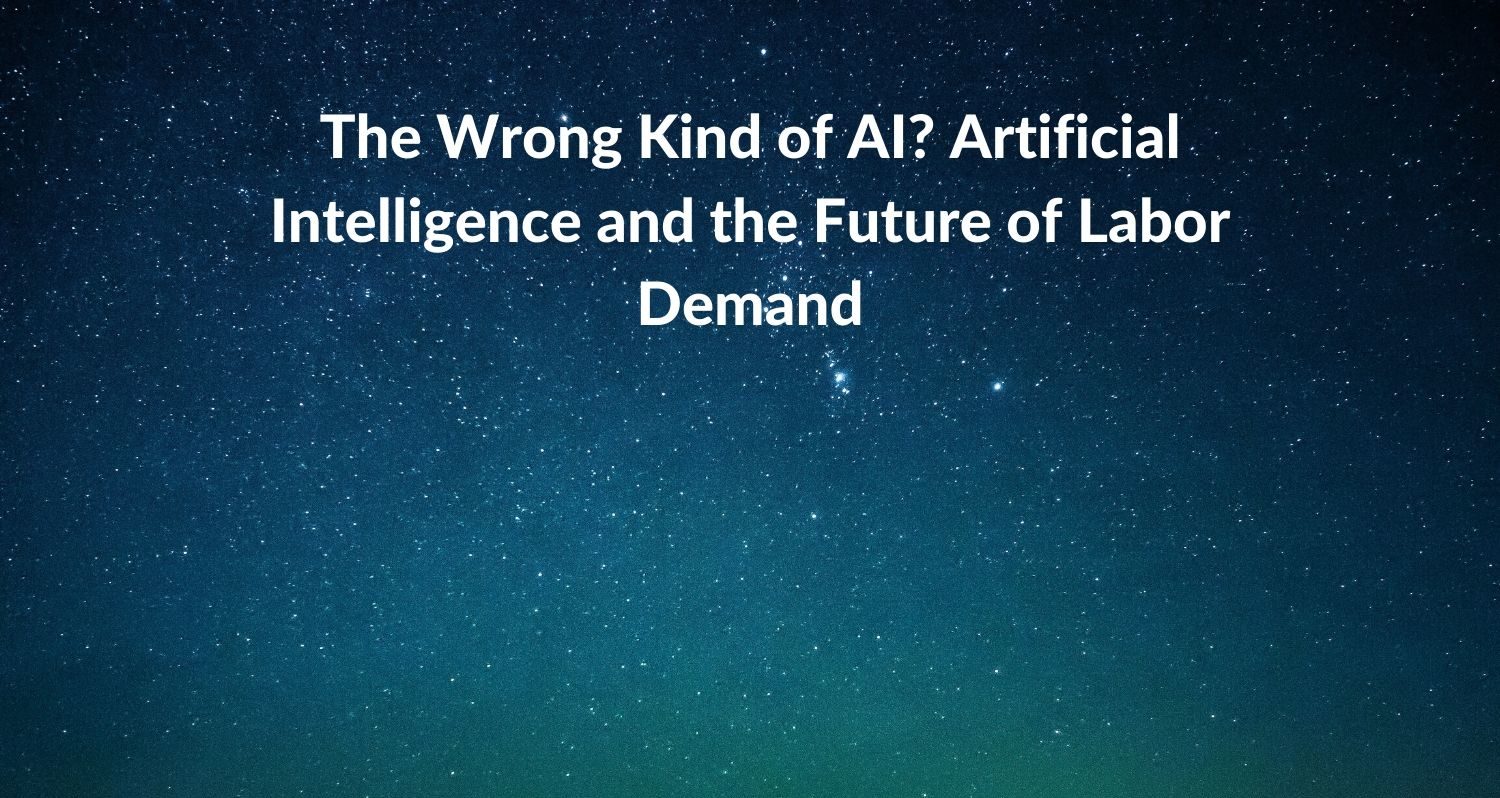
This paper by two economists (Daron Acemoglu and Pascual Restrepo) provides some grounding for these discussions in terms of how to approach this dilemma in trying to develop the right kind of AI systems that can create social and economic benefits.
Do increasing efficiency and social benefits stand in exclusion to each other when it comes to automation technology? With the development of the “right” kind of AI, this doesn’t have to be the case. AI is a general purpose technology that has wide applications and being offered as a platform, it allows others to build advanced capabilities on top of existing systems creating an increasingly powerful abstraction layer with every layer.
According to the standard approach in economics, a rise in productivity is often accompanied with an increase in the demand for labor and hence a rise in wages along with standards of living. But, when there is a decoupling between the deployment of technology and the associated productivity accrual, it can lead to situations where we see more output but not a corresponding increase in the standards of living as the benefits accrue to capital owners rather than wage-earning labor which is distanced from the production lifecycle. This unevenness in the distribution of gains causes losses of jobs in one sector while increasing productivity in others, often masking effects at an aggregate level through the use of purely economic focused indicators like GDP growth rates.
The authors expound on how the current wave of automation is highly focused on labor replacement driven by a number of factors. When this comes in the form of automation that is just as good as labor but not significantly better, we get the negative effects as mentioned before, that is a replacement of labor without substantial increases in the standards of living. Most of these effects are felt by those in the lower rungs of the socio-economic ladder where they don’t have alternate avenues for employment and ascent. A common message is that we just have to wait as we did in the case of the industrial revolution and new jobs will emerge that we couldn’t have envisioned which will continue to fuel economic prosperity for all. This is an egregious comparison that overlooks that the current wave of automation is not creating simultaneous advances in technology that allow the emergence of a new class of tasks within jobs for which humans are well-suited. Instead, it’s increasingly moving into domains that were strongholds of human skills that are not easily repeatable or reproducible. What we saw in the past was an avenue made available to workers to move out of low skill tasks in agriculture to higher skill tasks in manufacturing and services.
Some examples of how AI development can be done the “right” way to create social benefits :
- In education, we haven’t seen a significant shift in the way things are done for close to 200 years. It has been shown that different students have different learning styles and can benefit from personalized attention. While it is infeasible to do so in a traditional classroom model, AI offers the potential to track metrics on how the student interacts with different material, where they make mistakes, etc., offering insights to educators on how to deliver a better educational experience. This is accompanied by an increase in the demand for teachers who can deliver different teaching styles to match the learning styles of students and create better outcomes.
- A similar argument can be made in the field of healthcare where AI systems can allow medical staff to spend more time with patients offering them personalized attention for longer while removing the need for onerous and drudgery in the form of menial tasks like data entry.
- Industrial robots are being used to automate the manufacturing line often cordoning off humans for safety reasons. Humans are also decoupled from the process because of a difference in the level of precision that machines can achieve compared to humans. But we can get the best of both worlds by combining human flexibility and critical thinking to address problems in an uncertain environment with the high degree of preciseness of machines by creating novel interfaces, for example, by using Augmented Reality.
An important distinction that the authors point out in the above examples is that they are not merely the job of enablers, humans that are used to train machines in a transitory fashion, but those that genuinely complement machine skills.
There are market failures when it comes to innovation and in the past, governments have helped mitigate those failures via public-private partnerships that led to the creation of fundamental technologies like the internet. But, this has decreased over the past two decades because of smaller amount of resources being invested by the government in basic research and the technology revolution becoming centered in Silicon Valley which has a core focus on automation that replaces labor, and with that bias and their funding of university and academic studies, they are causing the best minds of the next generation to have the same mindset.
Markets are also known to struggle when there are competing paradigms and once one pulls ahead, it is hard to switch to another paradigm even if it might be more productive thus leading to an entrenchment of the dominant paradigm. The social opportunity cost of replacing labor is lower than the cost of labor, pushing the ecosystem towards labor replacing automation. Without accounting for these externalities, the ecosystem has little incentive to move towards the right kind of AI. This is exacerbated by tax incentives imposing costs on labor while providing a break on the use of capital. Additionally, areas where the right kind of AI can be developed don’t necessarily fall into the cool domain of research and thus aren’t prioritized by the research and development community. Let’s suppose large advances were made in AI for health care. This would require accompanying retraining of support staff aside from doctors, and the high level bodies regulating the field would impose resistance, thus slowing down the adoption of this kind of innovation.
Ultimately, we need to lean on a holistic understanding of the way automation is going to impact the labor market and it will require human ingenuity to shape the social and economic ecosystems such that they create net positive benefits that are as widely distributed as possible. Relying on the market to figure this out on its own is a recipe for failure.