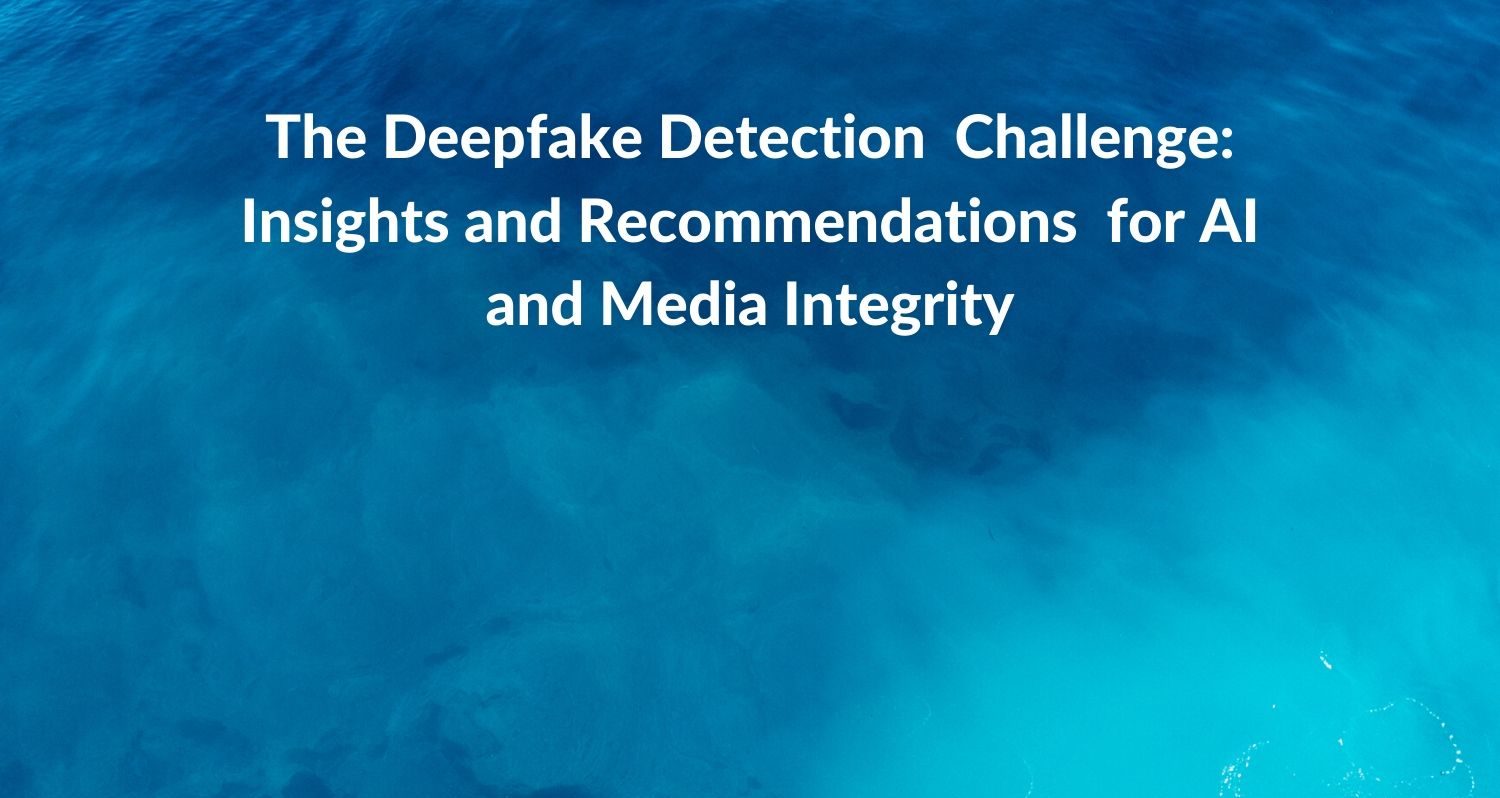
Top-level summary: Accurate and trustworthy information plays a key role in the development of a well-functioning society. When that is compromised by synthetic and non-synthetic actors polluting media streams with disinformation in the interest of confounding public discourse on important matters, we must fight back. This has come in sharp focus with the ongoing pandemic where every person is flush with loads of information but has difficulty ascertaining what is true and what isn’t. In an increasingly automated world, we’ll see further use of Deepfake technology to push malicious content onto people and the Deepfake Detection Challenge is meant to surface effective technical solutions to mitigate this problem. The Partnership on AI via their AI Media Integrity team compiled a helpful list of recommendations to improve the challenge but the lessons also apply widely to any others doing work in this space. At MAIEI, we have our own work ongoing with a team of professionals spanning UX design, machine learning, inclusive design, educational program development, media psychology, and industry that is helping to address challenges in the field. The document from PAI highlights the importance of building multi-stakeholder teams that have worked extensively with each other to improve the efficacy of the mechanisms so developed for media integrity. It also provides insights on how to construct datasets, how to put up scoring rules, responsible publishing practices and more that position efforts in the domain to succeed. The guidelines provided are actionable and encourage a mixed methods approach of combining technology with existing practices by journalists and fact checkers. It also advocates for teams building these solutions to make them available and accessible to the wider ecosystem such that we achieve defense in depth via the deployment of these tools at various points in the content lifecycle.
Synthetic media is any media (text, image, video, audio) that is generated by an AI system or that is synthesized. On the other hand, non-synthetic media is one that is crafted by humans using a panoply of techniques, including tools like Photoshop.
Detecting synthetic media alone doesn’t solve the media integrity challenges, especially as the techniques get more sophisticated and trigger an arms race between detection and evasion methods. These methods need to be paired with other existing techniques that fact checkers and journalists already use in determining whether something is authentic or synthesized. There are also pieces of content that are made through low tech manipulations like the Nancy Pelosi video from 2019 which showed her drunk but in reality it was just a slowed down video. Other such manipulations include simpler things like putting fake and misleading captions below the true video and people without watching the whole thing are misled into believing what is summarized in the caption. In other cases, the videos might be value neutral or informative even when they are generated so merely detecting something as being generated doesn’t suffice. A meaningful way to utilize automated tools is a triaging utility that flags content to be reviewed by humans in a situation where it is not possible to manually review everything on the platform.
While tech platforms can build and utilize tools that help them with these tasks, the adjacent possible needs of the larger ecosystem need to be kept in mind such that they can be served at the same time, especially for those actors that are resource-constrained and don’t have the technical capabilities to build it themselves. The tools need to be easy to use and shouldn’t have high friction such that they become hard to integrate into existing workflows. Through open sourcing and licensing, the tools can be made available to the wider ecosystem but it can create the opportunity for adversaries to strengthen their methods as well. This can be countered by responsible disclosure as we’ll cover below.
For any datasets created as a part of this challenge and otherwise to aid in detection, one must ensure that it captures sufficient diversity in terms of environment and other factors and reflects the type of content that might be encountered in the world. The scoring rules need to be such that they minimize gaming and overfitting and capture the richness of variation that a system might encounter. For example most datasets today in this domain aim to mitigate the spread of pornographic material. They also need to account for the vastly different frequencies of occurrence of authentic and generated content.
Solutions in this domain involve an inherent tradeoff between pro-social use and potential malicious use for furthering the quality of inauthentic content. The release of tools should be done in a manner that enhances pro-social use while creating deterrents for malicious use. The systems should be stress-tested by doing red team-blue team exercises to enhance robustness because this is inherently an adversarial exercise. Such challenges should be held often to encourage updating of techniques because it is a fast evolving domain where progress happens in the span of a few months.
Results from such detection need to be accessible to the public and stakeholders and explanations for the research findings should be made available alongside the challenge to encourage better understanding by those that are trying to make sense of the digital content. Responsible disclosure practices will be crucial in enabling the fight against disinformation to have the right tools while deterring adversaries from utilizing the same tools to gain an advantage. A delayed release mechanism where the code is instantly made available to parties in a non-open source manner while the research and papers are made public with the eventual release of the code as well after a 6-12 months delay which would help with the detectors having a headstart over the adversaries.
Such detection challenges can benefit from extensive multi-stakeholder consultations which require significant time and effort so budget for that while crafting and building such challenges. Some of the allocation of prize money should be towards better design from a UX and UI perspective. It should also include explainability criteria so that non-technical users are able to make sense of the interventions and highlights of fake content such as bounding boxes around regions of manipulations. The process of multi-stakeholder input should happen at an early stage allowing for meaningful considerations to be incorporated and dataset design that can be done appropriately to counter bias and fairness problems.
Finally, strong, trusting relationships are essential to the success of the process and require working together over extended periods to have the hard conversations with each other. It is important to have clear readings ahead of meetings that everyone has to complete so that discussions come from an informed place. Spending time scoping and coming to clearer agreement about projects goals and deliverables at the beginning of the process is also vital to success.
Original paper by Partnership on AI: https://www.partnershiponai.org/wp-content/uploads/2020/03/671004_Format-Report-for-PDF_031120-1.pdf