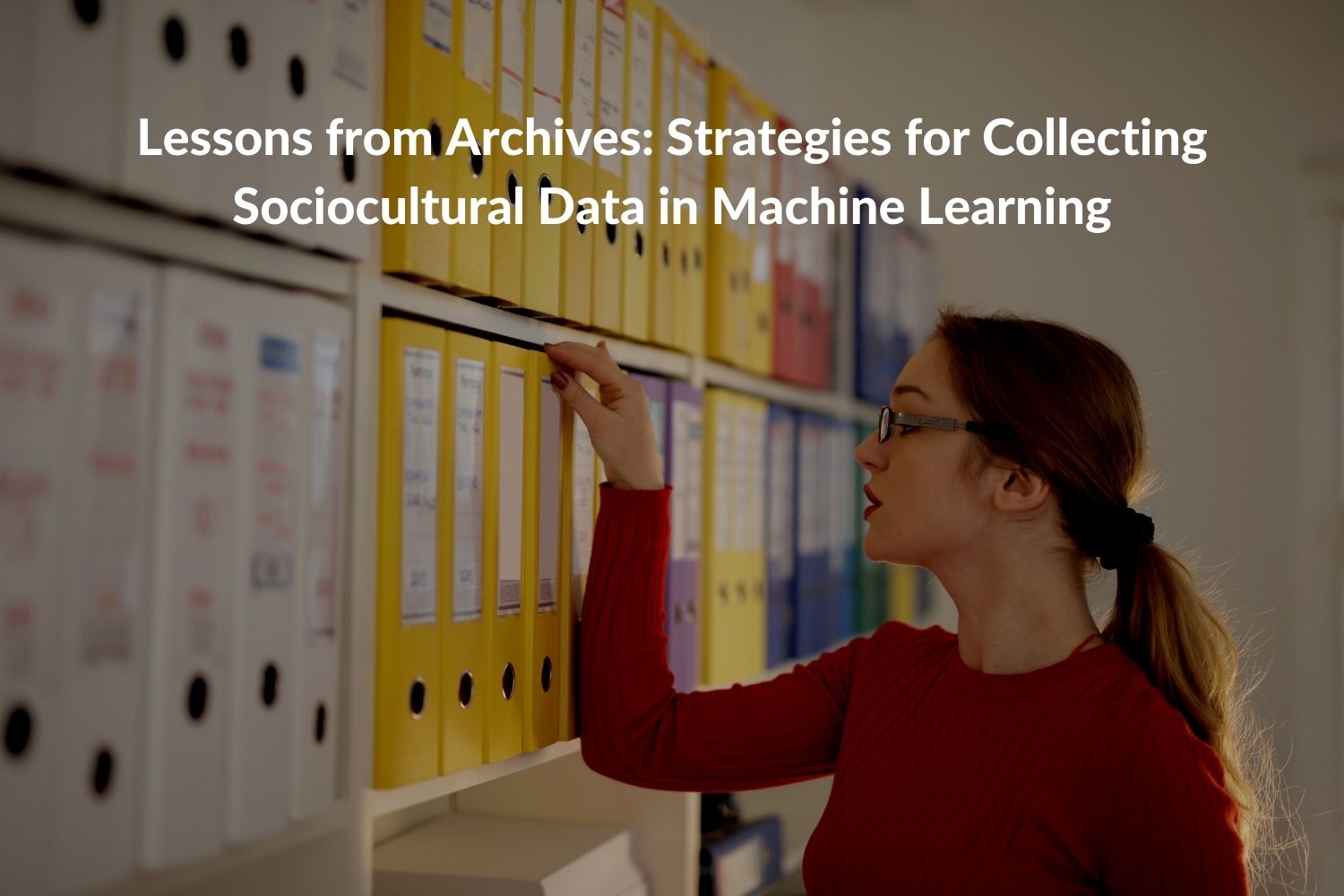
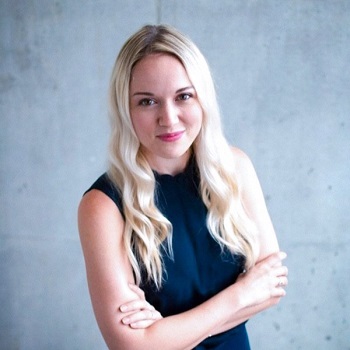
Summary contributed by our researcher Victoria Heath (@victoria_heath7), Communications Manager at Creative Commons
*Authors of full paper & link at the bottom
Mini-summary: It’s no secret that there are significant issues related to the collection and annotation of data in machine learning (ML). Many of the ethical issues that are discussed today in ML systems result from the lack of best practices and guidelines for the collection and use of data to train these systems. For example, Professor Eun Seo Jo (Stanford University) and Timnit Gebru (Google) write, “Haphazardly categorizing people in the data used to train ML models can harm vulnerable groups and propagate societal biases.”
In this article, Seo Jo and Gebru set out to examine how ML can apply the methodologies for data collection and annotation utilized for decades by archives: the “oldest human attempt to gather sociocultural data.” They argue that ML should create an “interdisciplinary subfield” focused on “data gathering, sharing, annotation, ethics monitoring, and record-keeping processes.” In particular, they explore how archives have worked to resolve issues in data collection related to consent, power, inclusivity, transparency, and ethics & privacy—and how these lessons can be applied to ML, specifically to subfields that use large, unstructured datasets (e.g. Natural Language Processing and Computer Vision).
The authors argue that ML should adapt what archives have implemented in their data collection work, including an institutional mission statement, full-time curators, codes of conduct/ethics, standardized forms of documentation, community-based activism, and data consortia for sharing data.Â
Full summary:
It’s no secret that there are significant issues related to the collection and annotation of data in machine learning (ML). Many of the ethical issues that are discussed today in ML systems result from the lack of best practices and guidelines for the collection and use of data to train these systems. For example, Professor Eun Seo Jo (Stanford University) and Timnit Gebru (Google) write, “Haphazardly categorizing people in the data used to train ML models can harm vulnerable groups and propagate societal biases.”
In this article, Seo Jo and Gebru set out to examine how ML can apply the methodologies for data collection and annotation utilized for decades by archives: the “oldest human attempt to gather sociocultural data.” They argue that ML should create an “interdisciplinary subfield” focused on “data gathering, sharing, annotation, ethics monitoring, and record-keeping processes.” In particular, they explore how archives have worked to resolve issues in data collection related to consent, power, inclusivity, transparency, and ethics & privacy—and how these lessons can be applied to ML, specifically to subfields that use large, unstructured datasets (e.g. Natural Language Processing and Computer Vision).
The authors argue that ML should adapt what archives have implemented in their data collection work, including an institutional mission statement, full-time curators, codes of conduct/ethics, standardized forms of documentation, community-based activism, and data consortia for sharing data. These implementations follow decades of research and work done by archives to address “issues of concern in sociocultural material collection.”
There are important differences to note between archival and ML datasets, including the level of intervention and supervision. In general, data collection in ML is done without “following a rigorous procedure or set of guidelines,” and is often done without critiquing the origins of data, as well as the motivations behind the collection, and the potential impacts on society. Archives, on the other hand, are heavily supervised and have several layers of intervention that help archivists determine whether certain documents or sources should be added to a collection. Seo Jo and Gebru point out another important difference between ML and archival datasets: their motivations and objectives. For the most part, ML datasets are built to further train a system to make it more accurate, while archival datasets are built to preserve cultural heritage and educate society, with particular attention to “authenticity, privacy, inclusivity, and rarity of sources.”
The authors argue that there should be a more interventionist approach to data collection in ML, similar to what is done by archives. This is due to the fact that from the very beginning historical bias and representational bias infect data. Historical bias refers to the “structural, empirical inequities inherent to society that is reflected in the data,” and representational bias comes from the “divergence between the true distribution and digitized input space.” The best way to mitigate these biases is to implement what archives have put into place in their data collection practices, which includes:
- Drafting an institutional mission statement that prioritizes “fair representation or diversity” rather than “tasks or convenience.” This will prevent collection methods or even research questions from being driven solely by the accessibility and availability of datasets, which can replicate bias. It also encourages researchers to publicly explain their collection processes and allows for feedback from the public.
- Ensuring consent through community and participatory approaches. This is especially crucial for ML researchers who are building datasets based on demographic factors. “ML researchers without sufficient domain knowledge of minority groups,” write Seo Jo and Gebru, “frequently miscategorize data, imposing undesirable or even detrimental labels onto groups.” Archives have attempted to solve similar issues by creating community archives where collections are built and essentially “owned” by the community being represented. These archives are open to public input and contributions, often enabling minority groups to “consent to and define their own categorization.”
- Creating data consortia to increase “parity in data ownership.” Archives, alongside libraries, have created a consortia model through institutional frameworks that allow them to “gain economies of scale” by sharing resources and preventing redundant collections. This model has been adopted by the Open Data Institute, for example, to share data among researchers in ML. However, issues around the links between profit and data may prevent widespread adoption by ML companies and organizations.
- Encourage transparency by creating appraisal records and committee-based data collection practices. Archives follow rigorous record-keeping standards, including 1) data content standards, 2) data structure standards, and 3) data value standards that pass through several layers of supervision. They also record the process of their data collection to ensure even more transparency. ML should build and maintain similar standards in its data collection practices to address issues emanating from the public (and other researchers) about ML systems.
- Building overlapping “layers of codes on professional conduct” that guide and enforce decisions regarding ethical concerns. For archives, these codes are maintained and enforced by international groups (e.g. International Council on Archives), and because many archivists are employed as professional data collectors they are held to specific standards that are enforced by ethics panels or committees. ML could benefit immensely from creating similar mechanisms that ensure accountability, transparency, and ethical responsibility.
Of course, there are limitations to the ML field’s ability to adopt the measures outlined above. In particular, the authors argue, the sheer amount of data in ML datasets is much larger than many archives and the resources needed to implement these measures may be beyond what many ML-focused companies, researchers, etc. are willing to commit to. This is especially due to the fact that their motivations are primarily profit-focused. However, the ML community must contend with and end its current, problematic data collection practices—and a “multi-layered” and “multi-person” intervention system informed by systems put into place by archives would be a good place to start.
Original paper by Eun Seo Jo (Stanford University) and Timnit Gebru (Google): https://arxiv.org/abs/1912.10389