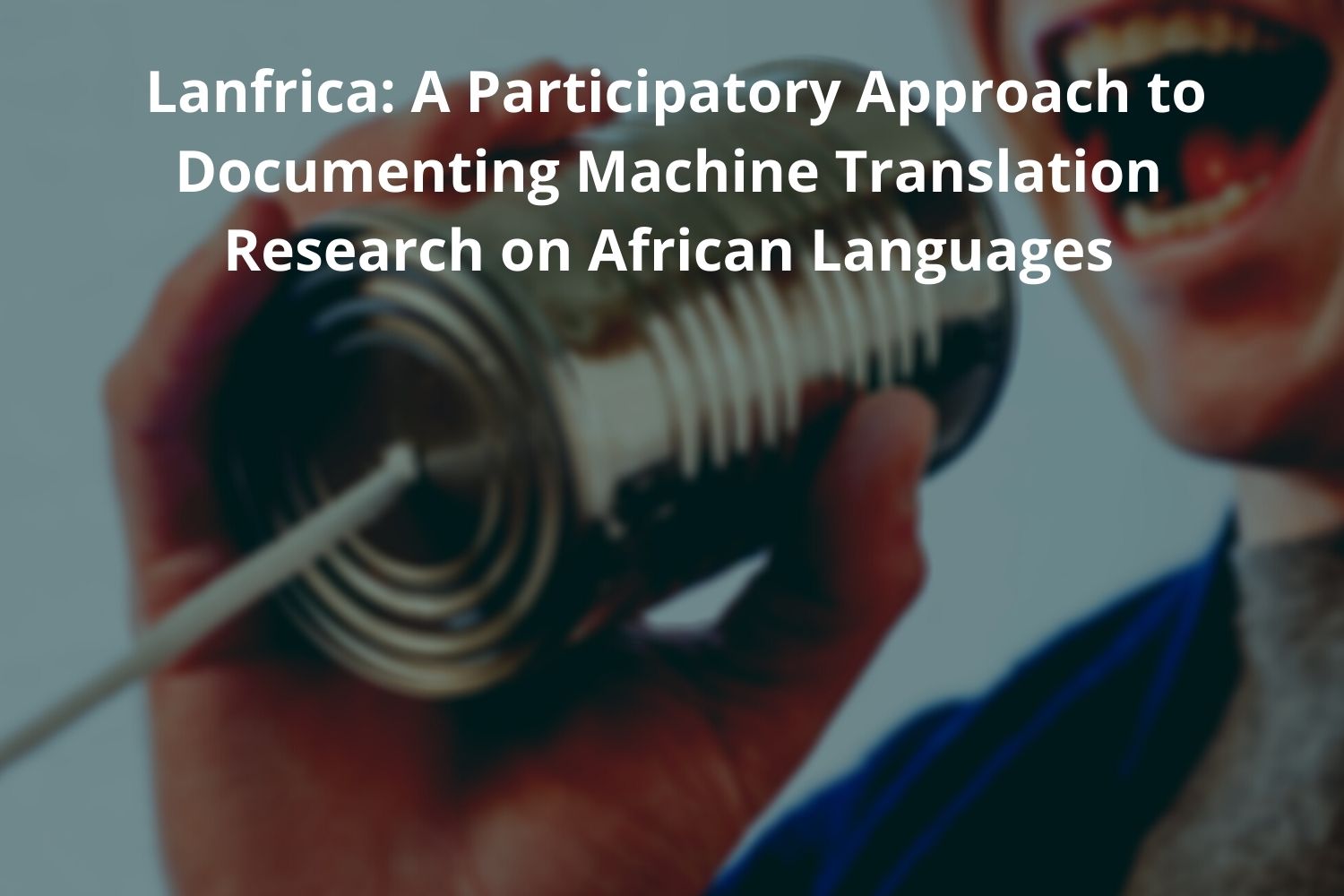
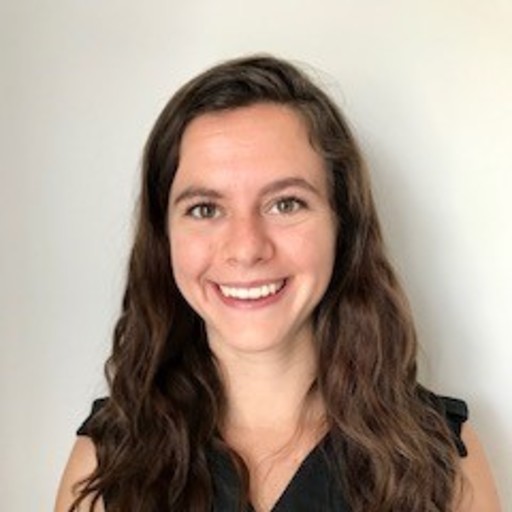
Summary contributed by our researcher Alexandrine Royer, who works at The Foundation for Genocide Education.
*Link to original paper + authors at the bottom.
Mini-summary: It is no secret that English has dominated the machine learning landscape. Yet, multilingual researchers worldwide are trying to change the narrative and put their language on the digital map. With machine learning research efforts springing up across the continent, which is home to over 1500 languages, it is difficult to coordinate and keep track of current research happening in silos. Emezue et Dossou found that a significant hindrance to the advancement of MT research on African languages is the lack of a central database that gives potential users quick access to benchmarks and resources and enables them to build comparative models. The authors propose an open-source and publicly available database, titled Lanafrica, that will allow users from the scientific and non-scientific community to catalog and track the latest research on machine learning developments in African languages.
Full summary:
English has become the lingua franca of machine learners and data scientists, yet a minority of fewer than 26% of internet users speak it. Against this trend, there have been a growing number of initiatives to include African languages in machine translation research, and in particular, natural learning processes for online platforms. Africa is the continent with the highest language diversity, being home to over 1500 documented languages, and over 40% of its population uses social media platforms. To keep track of these ongoing developments, Emezue et Dossou offers Lanfrica a participatory-led framework in documenting researches, projects, benchmarks, and datasets on African languages.
As Emezue et Dossou points out, there are already several existing online communities dedicated to promoting AI research in Africa, such as Masakhane, Deep Learning Indaba, BlackinAI and Zindi. These organizations reflect not only a desire to put Africa forward in machine learning but also to preserve the continent’s distinct cultures within the digital space. Some limitations currently hinder the advancement of African natural language processes, including:
- A lack of confidence from African societies that their languages can be a prevalent mode of communication in the future
- A lack of resources for African languages
- A lack of publicly available benchmarks
- Minimal sharing of existing research and code
To redress these issues of lack discoverability, publicly available benchmarks, and sharing of resources, Emezue et Dossou created an open-source and user-friendly database system that documents machine learning researches, research-results, benchmarks, and projects on African languages. By surveying the Masakhane community, an open-source group of NLP researchers, the authors found that to build a neural machine translation (NMT) model, researchers had difficulty accessing model comparisons to guide them in data preparation, model configuration, training, and evaluation.
The soon-to-be-launched Lanafrica website will catalog ongoing ML research efforts based on the African language of interest and allow users to submit information on their projects, with contributions coming from both researchers and non-researchers alike. To improve ML reproducibility, links that provide access to open-source test data will be featured on the website.
Despite being a growing pole of ML research, Africa is underrepresented in discussions surrounding AI, often overshadowed by academic and corporate research labs in wealthy bubbles such as Silicon Valley and Zhongguancun. Digital assistants like Siri, Google Talk, and Alexa have yet to be programmed to accommodate widely-spoken languages such as Lingala, Oromo, and Swahili, and Google Translate only offers translations for 13 African languages. Unlike large databases such as Google scholar, Lanafrica is an initiative that is specifically tailored to African language researchers, allowing them to build networks in a digital space that reflects their interests and priorities. As the most linguistically diverse place on Earth, natural language machine learners in North America and Asia can also benefit from learning about the advances in Africa.
Original paper by Chris C. Emezue, Bonaventure F.P. Dossou: https://arxiv.org/pdf/2008.07302.pdf