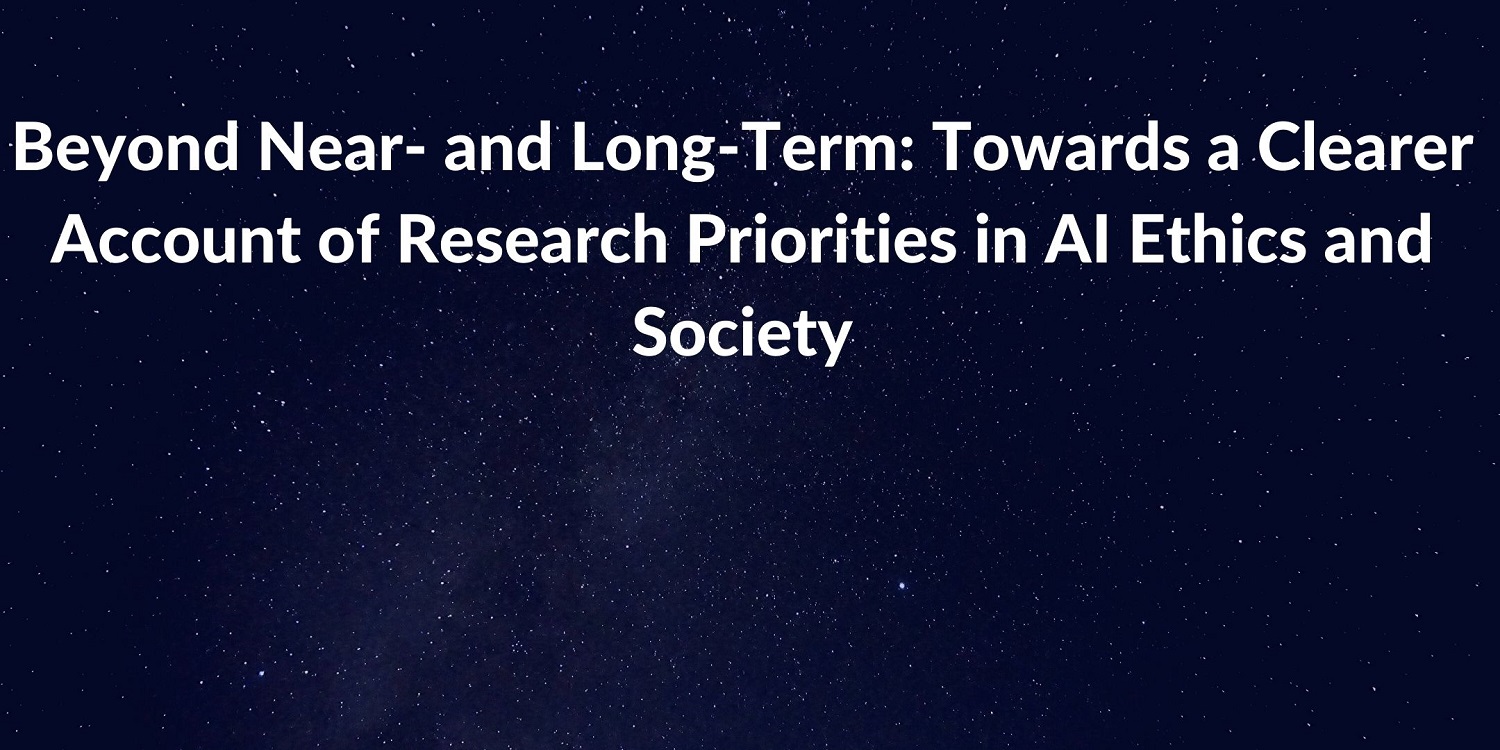
A much needed paper by Carina Prunkl and Jess Whittlestone shedding light in a polarized research and practice community that can clearly benefit from more collaboration and greater understanding of each other’s work. The paper proposes a multi dimensional spectrum based approach to delineate near and long term AI research along the lines of extremity, capability, certainty and impact. Additionally, it asks for more rigour from the community when communicating their research agendas and motives to allow for greater understanding between this artificial divide. While elucidating differences along these different axes and visualizing them reveals how misunderstanding arises, it also highlights ignored yet important research areas, ones that the authors are focused on.
This paper dives into how researchers can clearly communicate about their research agendas given ambiguities in the split of the AI Ethics community into near and long term research. Often a sore and contentious point of discussion, there is an artificial divide between the two groups that seem to take a reductionist approach to the work being done by the other. A major problem emerging from such a divide is a hindrance in being able to spot relevant work being done by the different communities and thus affecting effective collaboration. The paper highlights the differences arising primarily along the lines of timescale, AI capabilities, deeper normative and empirical disagreements.
The paper provides for a helpful distinction between near- and long-term by describing them as follows:
- Near term issues are those that are fairly well understood and have concrete examples and relate to rêvent progress in the field of machine learning
- Long term issues are those that might arise far into the future and due to much more advanced AI systems with broad capabilities, it also includes long term impacts like international security, race relations, and power dynamics
What they currently see is that:
- Issues considered ‘near-term’ tend to be those arising in the present/near future as a result of current/foreseeable AI systems and capabilities, on varying levels of scale/severity, which mostly have immediate consequences for people and society.
- Issues considered ‘long-term’ tend to be those arising far into the future as a result of large advances in AI capabilities (with a particular focus on notions of transformative AI or AGI), and those that are likely to pose risks that are severe/large in scale with very long-term consequences.
- The binary clusters are not sufficient as a way to split the field and not looking at underlying beliefs leads to unfounded assumptions about each other’s work
- In addition there might be areas between the near and long term that might be neglected as a result of this artificial fractions
Unpacking these distinctions can be done along the lines of capabilities, extremity, certainty and impact, definitions for which are provided in the paper. A key contribution aside from identifying these factors is that they lie along a spectrum and define a possibility space using them as dimensions which helps to identify where research is currently concentrated and what areas are being ignored. It also helps to well position the work being done by these authors.
Something that we really appreciated from this work was the fact that it gives us concrete language and tools to more effectively communicate about each other’s work. As part of our efforts in building communities that leverage diverse experiences and backgrounds to tackle an inherently complex and muti-dimensional problem, we deeply appreciate how challenging yet rewarding such an effort can be. Some of the most meaningful public consultation work done by MAIEI leveraged our internalized framework in a similar vein to provide value to the process that led to outcomes like the Montreal Declaration for Responsible AI.