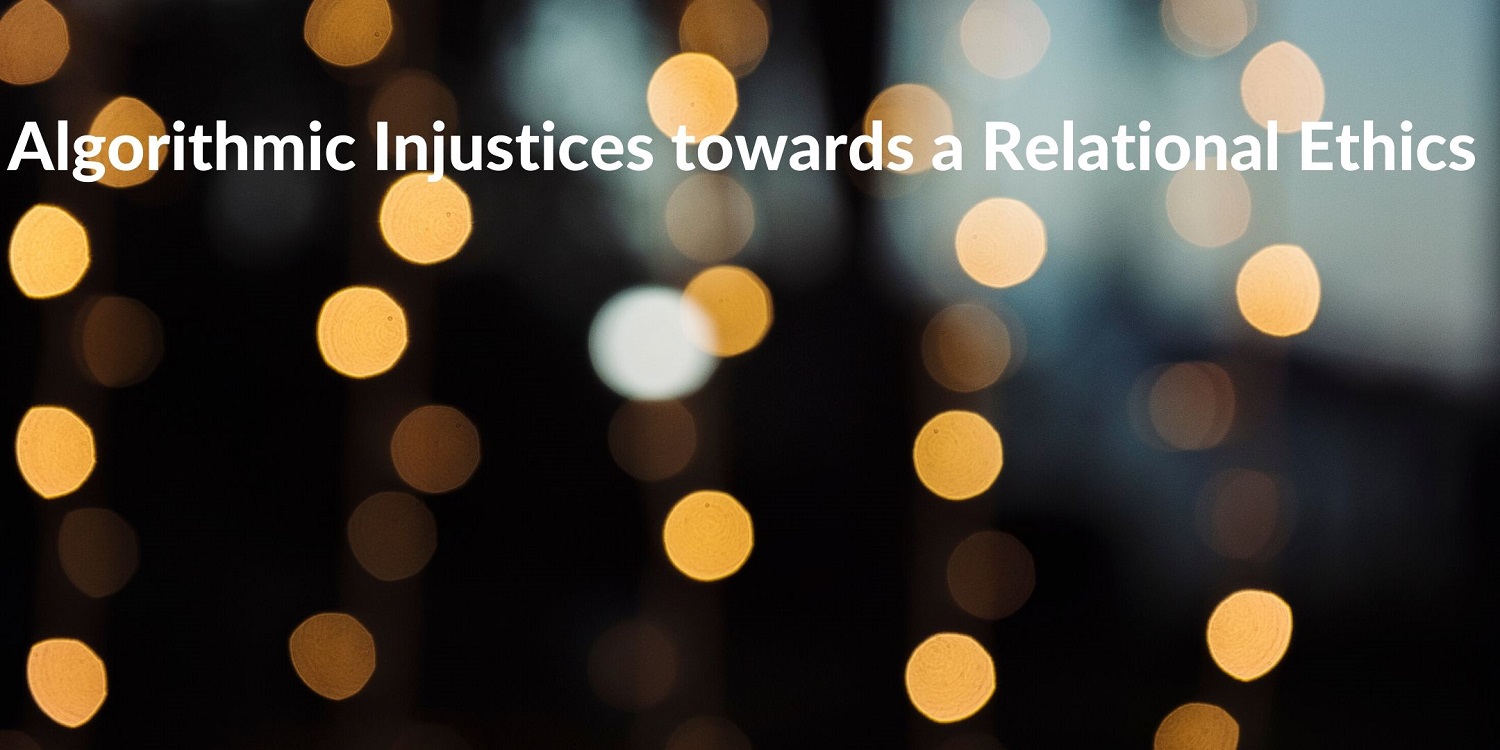
This paper presented by Abebe Birhane and Fred Cummins at the Black in AI workshop at NeurIPS 2019 elucidates how the current paradigm in research on building fair, inclusive AI systems falls short in addressing the real problems because of taking a narrow, technically focused approach. The paper utilizes a relational ethics approach to highlight areas of improvement. The key arguments emerging from such a characterization are centering the population that is going to be disproportionately impacted, focusing on understanding of underlying context rather than pure predictive power of the systems, viewing the algorithmic systems as a tool that can shape and sustain social and moral order and recognizing the temporal nature of the definitions of bias, fairness, etc and keeping the design and development of the systems as an iterative process.Â
The paper starts by setting the stage for the well understood problem of building truly ethical, safe and inclusive AI systems that are increasingly leveraging ubiquitous sensors to make predictions on who we are and how we might behave. But, when these systems are deployed in socially contested domains, for example, “normal” behaviour where loosely we can think of normal as that defined by the majority and treating everything else as anomalous, then they don’t make value-free judgements and are not amoral in their operations. By viewing the systems as purely technical, the solutions to address these problems are purely technical which is where most of the fairness research has focused and it ignores the context of the people and communities where these systems are used. The paper serves to question the foundations of these systems and to take a deeper look at unstated assumptions in the design and development of the systems. It urges the readers, and the research community at large, to consider this from the perspective of relational ethics. It makes 4 key suggestions:Â
- Center the focus of development on those within the community that will face a disproportionate burden or negative consequences from the use of the system
- Instead of optimizing for prediction, it is more important to think about how we gain a fundamental understanding of why we’re getting certain results which might be arising because of historical stereotypes that were captured as a part of the development and design of the system
- The systems end up creating a social and political order and then reinforcing it, meaning we should involve a larger systems based approach to designing the systems
- Given that the terms of bias, fairness, etc evolve over time and what’s acceptable at some time becomes unacceptable later, the process asks for constant monitoring, evaluation and iteration of the design to most accurately represent the community in context.
At MAIEI, we’ve advocated for an interdisciplinary approach leveraging the citizen community spanning a wide cross section to best capture the essence of different issues as closely as possible from those who experience them first hand. Placing the development of an ML system in context of the larger social and political order is important and we advocate for taking a systems design approach (see A Primer in Systems Thinking by Donna Meadows) which creates two benefits : one is that several ignored externalities can be considered and second to involve a wider set of inputs from people who might be affected by the system and who understand how the system will sit in the larger social and political order in which it will be deployed. Also, we particularly enjoyed the point on requiring a constant iterative process to the development and deployment of AI systems borrowing from cybersecurity research on how security of the system is not done and over with, requiring constant monitoring and attention to ensure the safety of the system.