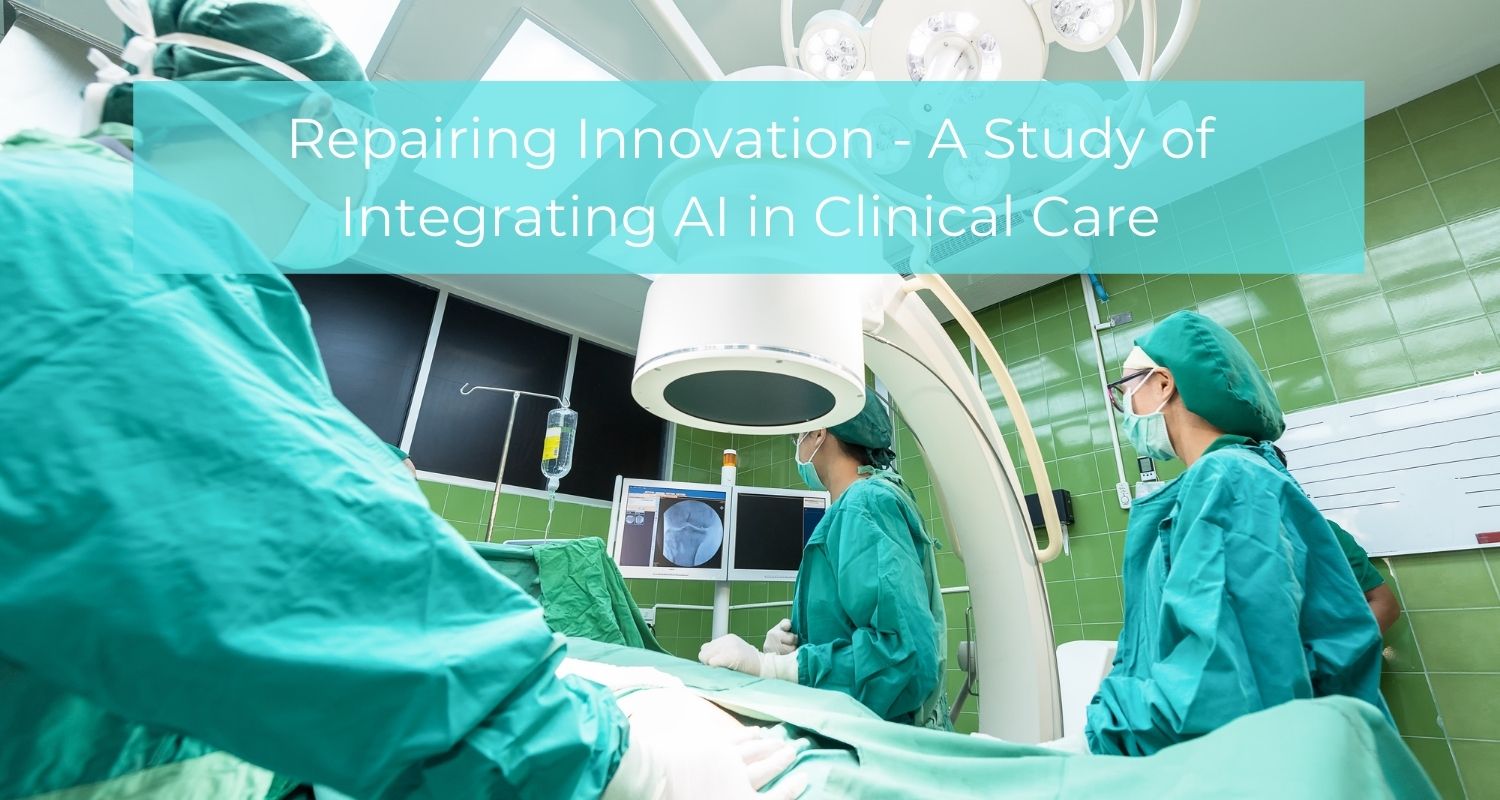
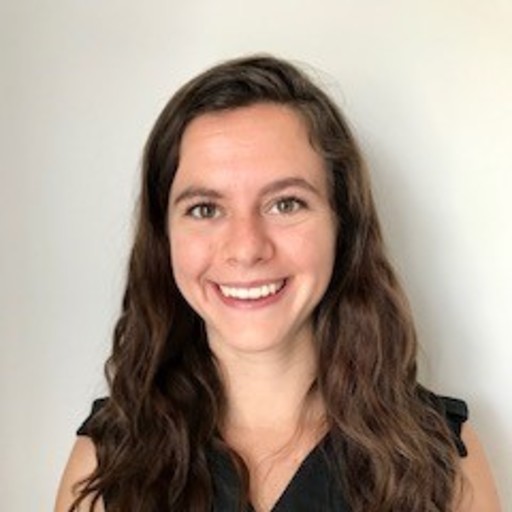
Summary contributed by our researcher Alexandrine Royer, who works at The Foundation for Genocide Education.
*Link to original paper + authors at the bottom.
Overview: This report follows the implementation of Sepsis Watch, an AI-powered medical diagnosis tool at Duke Hospital, and uncovers the interplay between technical infrastructure, human actors and expert knowledge that allow for the successful integration of new medical technologies.
Health care is one of the areas in which AI promises to bring celebrated advances to patients’ and medical practitioners’ lives. Outspoken medical professionals such as Eric Topol have stated that, paradoxically, machine-led and digital medicine can bring back the central elements of human interactions in care by giving hospital workers the “gift of time.” AI technologies can alleviate the time-pressed schedules of medical professionals through assisting in the early detection and treatment of disease, effectively acting as a safety net against fatigue-induced human oversight, error in judgement and mistaken gut instinct. Sepsis, a highly prevalent and deadly infection in US hospitals, requires early detection for treatment to be efficient. According to the Centers for Disease Control and Prevention, one in three patients who dies in hospitals has sepsis. However, as underscored by Elish and Watkins, sepsis is notoriously challenging to diagnose consistently in its early stages when patients are most receptive to treatment, making it a prime target for AI-based medical interventions.
Elish and Watkins have produced an in-depth study of Sepsis Watch, an AI-powered monitoring tool launched by Duke University and Duke Health system that uses deep learning and hospital protocols to improve sepsis treatment quality. Rather than a simple technological intervention or a more reductionist view, a machine learning model, Watkins and Elish, underline how Sepsis Watch is a complex sociotechnical system. To elaborate, social context, relationships, and power dynamics are central to creating, designing, and implementing this technology. In their introductory statement, the authors demonstrate how AI technologies are often uncritically conceived as the solution to medical challenges yet are rarely tested “in the wild,” and their disruptive effects within the environment they are launched frequently escape analysis. In their words, “technology-driven innovation is disruptive; it will always require corresponding repair work to complete the process of effective innovation.” Part of this repair work entails creating new sets of practices within existing workflows that allow for the successful integration and application of medical technologies.
Sepsis Watch was piloted at Duke Emergency Department as part of routine clinical care for two and a half years. The Sepsis Watch Model was built on a dataset curated by Duke Health clinicians, who identified the necessary variables, and contained over 32 million data points. It can only identify correlations in patient characteristics and the likelihood of sepsis -it works to signal if a patient is at risk, and not why. To describe how Sepsis Watch works, when a patient is admitted to the emergency department, their personal health record data is processed by the Sepsis Watch system, used on an IPad application, which signals whether or not they are high risk. This information is received by a rapid response nurse who transmits the information to the patient’s attending ED physician, with the latter being responsible for making the final call whether to begin sepsis treatment. If so, the treatment by ER clinicians is tracked on the application until it is completed.
Sepsis Watch’s success in patient treatment relies on the fluid interplay between human actors, technical infrastructure, and expert medical knowledge. However, none of these interactions are as straightforward as one would expect. As the application’s monitoring was left in the primary hands of a specialized team of rapid response nurses (RRTs), they needed to develop new communication procedures with ED physicians. Part of this involved reversing normative working hierarchies, as nurses were now responsible for making diagnosis calls to attending physicians, causing some initial tensions in the workplaces. The exchange of information was made especially difficult as RRT nurses would not see the patients and only responded to Sepsis Watch alerts. Working patterns among ED physicians had to be modified to ensure that sepsis risk was well-communicated. The app also revealed the hospital managerial staff’s different perceptions, who saw ED as the focal point for sepsis diagnosis, and ED physicians who did not consider the disease a priority in the department and complained it hindered their professional discretion. RRTs had to find new strategies and techniques to ensure that doctors would follow up with their sepsis alerts and minimize their annoyance.
Although the pilot project results will only be released next year, the authors report that hospital managers found that Sepsis Watch had “dramatically improved the care of patients who are diagnosed with sepsis.” However, the application’s success did not rest solely on its technological capabilities, but rather the skills of RRT nurses who were tasked with setting the system in motion and attenuating its disruptive effects through repair work. The authors wonder whether improvement in patient care was due to the RRTs reminding calls over sepsis, keeping it fresh in physicians’ minds, rather than alerts from the app itself.
Ellis and Watkin’s report reveals the importance of studying medical technologies’ implementation within hospital settings and closely attending to those bumps along the road. Such studies reveal how AI-power medical technologies must not be evaluated solely on their capacity to produce medical results but also their impact on existing workplace flows and professional norms. Seeing medical technologies as part of broader social networks means considering the power, often gendered, dynamics of hospital workplaces and recognizing the emotional labour that goes into ensuring the adoption of a new system. This also entails a rethinking of the division of labour within hospital settings and proper compensation for the tactical knowledge and new kinds of expertise required of healthcare practitioners. While Sepsis Watch may have given physicians more time to attend to their patient’s risk of sepsis, it entailed putting additional responsibilities on RRTs. Indeed, referring back to Eric Topol, before celebrating the gift of time of AI medical technologies, there needs to be closer studies of the time-consuming and labour-intensive process of implementing these very technologies.
Original paper by Madeleine Clare Elish, Elizabeth Anne Watkins: https://datasociety.net/wp-content/uploads/2020/09/Repairing-Innovation-DataSociety-20200930-1.pdf