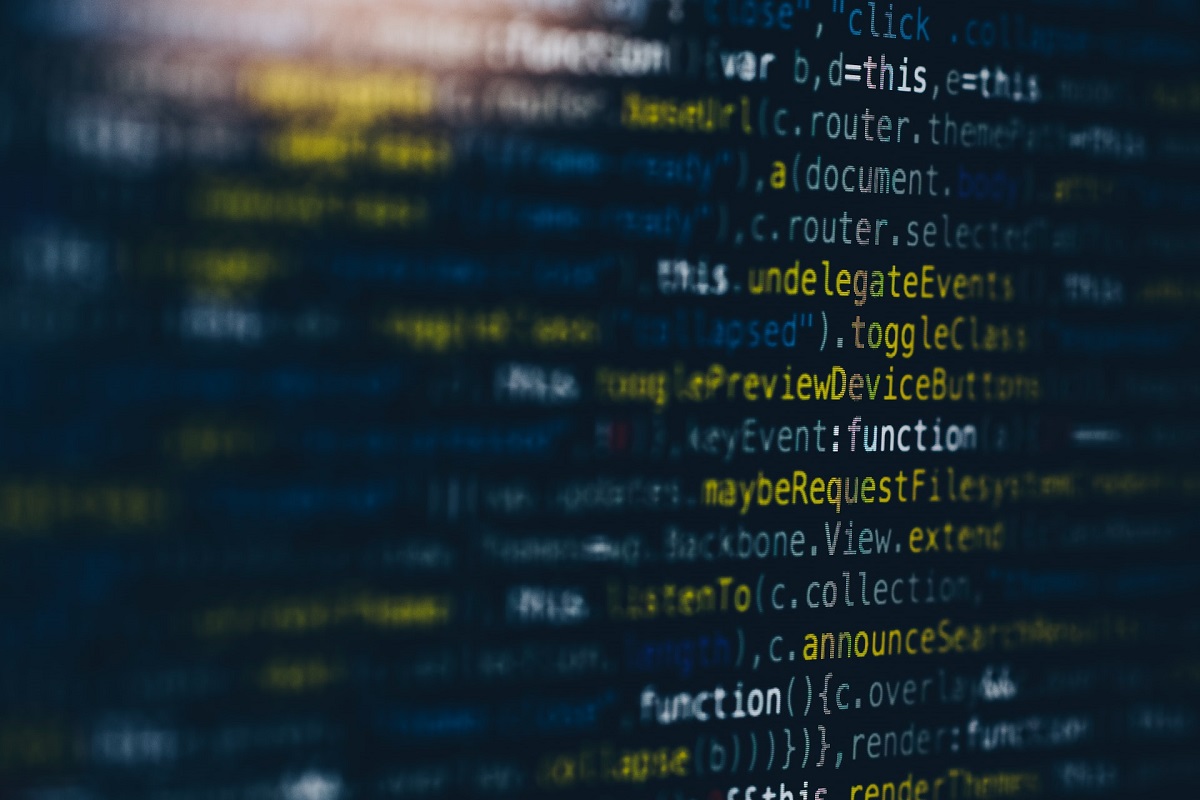
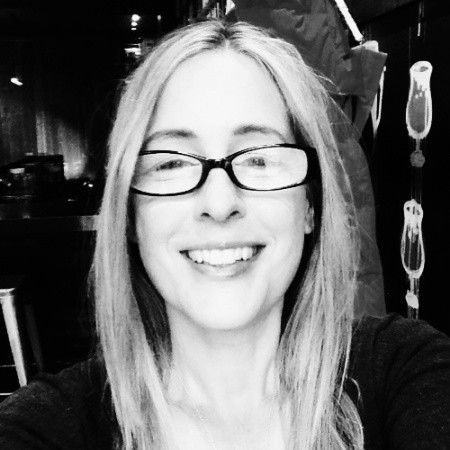
✍️ Column by Natalie Klym, who has been leading digital technology innovation programs in academic and private institutions for 25 years including at MIT, the Vector Institute, and University of Toronto. Her insights have guided the strategic decisions of business leaders and policy makers around the world. She strives for innovation that is open, creative, and responsible.
This is part 1 of Natalie’s Permission to Be Uncertain series.
The interviews in this series explore how today’s AI practitioners, entrepreneurs, policy makers, and industry leaders are thinking about the ethical implications of their work, as individuals and as professionals. My goal is to reveal the paradoxes, contradictions, ironies, and uncertainties in the ethics and responsibility debates in the growing field of AI.
I believe that validating the lack of clarity and coherence may, at this stage, be more valuable than prescribing solutions rife with contradictions and blind spots. This initiative instead grants permission to be uncertain if not confused, and provides a forum for open and honest discussion that can help inform tech policy, research agendas, academic curricula, business strategy, and citizen action.
Interview with Michael Conlin, inaugural Chief Data Officer and Chief Business Analytics Officer (2018-2020), US Department of Defense, May 2021
Perhaps the most fundamental paradox when discussing AI ethics emerges when exploring AI within a domain that is itself regarded by many as unethical. Warfare is arguably the most extreme case. Such domains represent harsh realities that are nonetheless better confronted than avoided. For this interview, I was largely inspired by Abhishek Gupta’s writings on the use of AI in war and his summary of the paper, “Cool Projects” or “Expanding the Efficiency of the Murderous American War Machine?” that investigated AI practitioners’ views on working with the U.S. Department of Defense. (See Gupta’s summary here, full paper here.)
Michael, you were the DoD’s first Chief Data Officer (CDO), hired in July 2018. What did the creation of this position signify?
The DoD, like the rest of the public sector, was about 35 years behind in terms of a data strategy. There was actually an initial wave of CDOs hired in government about 10 years earlier. But the goal back then was to protect or safeguard data. Ten years later, the goals were the complete opposite. There was a shift from secrecy to sharing–making data available to the right people and ensuring quality of the data, for purposes of better decision making.
What motivated you to join the US Department of Defense, and why were they interested in you specifically?
They were interested in me because I brought commercial sector know-how to public service. That is a generalized trend in the public sector — to learn from private-sector best practices.
In terms of my personal motivation to join the DoD, I knew they had a big problem with data fragmentation and I wanted to solve it. I enjoy leading digital transformation. They are the largest organization in the world. There are 13,000 IT systems within the department. So there was no department-wide view or decision-making possible. Furthermore, the authoritative structure of the organization added to the balkanization of data. Every system had its own authorized access, i.e., no single person had authority over all the data. The opportunity to offset both data fragmentation and an authoritative organizational culture was interesting to me. And I was going to be senior enough to accomplish something.
The fact that it was an inaugural role was exciting. I never thought I was good enough at anything to be the first one to do it, but it intrigued me as a distinction. They had initially talked about a “data czar,” a title I found entertaining, and then they changed it to Chief Data Officer.
There was also an element of patriotism. I wanted to serve my country and contribute to the safeguarding of our nation.
Within my capacity as CDO, I saw a more specific opportunity to make a positive difference in the way AI is being adopted and implemented in this country. I was particularly concerned with some of the irresponsible practices I had seen coming out of Silicon Valley with regards to AI. The general attitude is captured in Facebook’s motto, “Move fast and break things,” but in some cases, these people were breaking people’s lives.
I studied psychology as an undergraduate so I understand the basics of statistics, testing, and measurement. I understand that data has to be a valid representation of the real world throughout its life cycle. But many of the people I had encountered in Silicon Valley were not careful with regards to these basics, and this offended me as a professional and as a human being. I wanted to make a difference.
So ethics and responsibility were not exactly part of the private sector “best practices.”
Correct. There’s a lot of talk about principles, but not a lot about how to actually apply these in practice. As part of my professional development, I participate in a series of study tours that take place twice a year. During my time at the DoD, these tours took me to Silicon Valley, New York, and London. I got direct exposure to how people were integrating principles into their techniques and methods, or not, as the case may be.
I would add that it’s not just Silicon Valley that needs to be more careful. The Covid crisis exposed just how complicated things can get even in the most well-intentioned, i.e., “AI for good,” contexts. In the early days, data-driven solutions for containing the spread of the virus proposed by AI researchers were typically framed as a choice between death versus privacy. That kind of framing certainly encouraged privileged members of society to consider giving up their privacy, but for many individuals and communities, this dichotomy doesn’t apply, especially when taken in historical context. In other words, the risk associated with collecting and sharing their demographic data has, historically, been systemic death or violence, including by state actors. The Indian residential school system in Canada during the late 1800s, and the ongoing reservation system, is a case in point and explains much of the resistance by some members of the indigenous community here and elsewhere to collecting and sharing data.
The Ada Lovelace Institute in the UK describes the emergence of a third stage of AI ethics that focuses on the actual application of the principles developed in earlier stages. Does that ring true for you?
I actually spent time at the Ada Lovelace Institute as part of my study. They had a very practical, clear-eyed, and down-to-earth way of looking at things. I loved their focus on actual outcomes. They represented the antithesis of Silicon Valley’s “move fast and break things” attitude. They encouraged a more thoughtful and responsible approach of considering possible positive and negative outcomes, as opposed to just going ahead and seeing what happens. It was about carefully considering the consequences of your actions.
In terms of your earlier comment regarding the goal of making data available to the right people so they can make better decisions, can you elaborate on what kinds of decisions were being made at the DoD?
The DoD is involved in several types of activities including military and humanitarian missions, and a lot of business administration. Back office operations represent about 75% of the department’s budget. My function as Chief Data Officer and subsequently Chief Business Analytics Officer was primarily focused on the business mission, e.g., things like financial management, logistics and supply chain, human resources, medical, real estate acquisition. We were trying to answer critical business questions from an enterprise-wide perspective. What’s the total number of truck tires of dimension X in all of our warehouses? Where are we renting office space that is within 20 miles of other Federal government-owned office space that is less than 50% occupied? What’s the total amount of money we spend with a given electrical utility? Is it better to continue running our own medical school or should we pay for aspiring doctors in uniform to attend the medical school of their choice?
Very little of my work was related to battlefield applications. In fact, only a very small percentage of what the DoD does is about targeted, precision fire, i.e., killing people and destroying things, and assessing the damage after the fact. Ideally, the DoD “holds the adversary at risk” realistically enough that shooting never starts. That said, a lot of the data supports the back office, humanitarian, and military missions simultaneously. You can’t always easily separate them.
What are the military equivalents of the “business” questions you outline above?
Battlefield/warfare activities were outside my purview, and there’s not much I can say here without violating the terms of my security clearance. But the most obvious example I can give you would be Battle Damage Assessment (BDA). For example, let’s say pilots execute a sortie, which means a combat mission, against a designated target. The “After Action” task is for analysts to review imagery to assess the performance of the sortie based on a BDA. The fundamental question is, did we take out the target we intended to take out? This is a complex data problem that requires increasingly sophisticated levels of detail and accuracy that match the increased accuracy of weapon systems.
So how did your learnings about ethics come into play?
I focused on building ethical principles into protocols for curating and analyzing data, across the life cycle. My main concern was whether the data provided a valid representation of the world. People like to talk about Artificial Intelligence because there’s a certain sizzle to the term. As a data practitioner, I know that AI, ML (Machine Learning), BA (Business Analytics), and BI (Business Intelligence) are all variations on a theme. You take some code and feed data into it. The code identifies patterns, relationships and probabilities. Then the code uses those to make decisions, predictions, or both. So the validity and accuracy (I’m using statistical terms here) of the data is critical.
For a long time, one of the fundamental guidelines of IT has been GIGO – garbage in, garbage out. It’s still true. And it’s more important than ever because of two things. First, there’s system bias and the tendency to believe something is correct because the system says so. Second is the sheer number of decisions we’re permitting code to make on behalf of organizations in both the commercial sector and public sector. Only we try to dress it up by referring to the code as algorithms. And when we’ve fed the data into the code we call the result trained algorithms. But what that obscures is that all code is flawed. I say that as someone who made a living by writing code. Even when the code is relatively clean, you have to select the right code for the purpose. Then you have to feed it the right amount of good-enough data (ProTip – all data are dirty and incomplete). Finally, you have to know how to interpret the results. So there are many ways the entire value chain can go wrong and deliver unintended consequences.
Now step away from the role of the data practitioner. As a responsible adult and citizen I have a responsibility to do the decent thing… to behave responsibly. We all do. The DoD role as CDO gave me a platform from which to have a direct positive impact on the way the DoD approached this responsibility.
Look, the DoD, and Federal government in general, is filled with dedicated, smart, highly professional people committed to public service. I understood that superficially before I joined them. Once I was on the inside I got a much greater appreciation of how committed they are to ethical behavior, by which I mean they were well aware of the potential for both good results and bad results in every decision. “Friendly fire” and “collateral damage” are not new concepts to the military.
That is a very important point. We tend to attribute pre-existing issues that intersect with AI as being specific to AI, as if AI itself is the issue.
Can you talk about the DoD AI ethics principles released in February 2020?
The principles had been in the works for over a year before release. They had been developed by a group of political appointees, which in my opinion was full of people attempting to create cover for a corrupt agenda. They slapped labels onto projects that made them sound good and interesting, but they had no clue how the project deliverables could be misused. And they promoted projects that would have compromised personal data by exposing it to commercial firms. The principles themselves are useful, as long as they are put into practice through meaningful disciplines.
There were 5 principles: Responsible AI, Equitable AI, Traceable AI, Reliable AI, and Governable AI. For me, the details regarding principles themselves were less interesting than the question of how to put them into practice. There are minor differences between one principle and another, what’s important is the outcome.
AI ethics is not a binary, either/or, thing. It’s a matter of degree and probability. There’s a ladder of creepiness when it comes to things like surveillance. The goal is to figure out where everyone is on that ladder, and stop the upward movement.
On a more general level, I believe that AI is an ideology. I reject the idea of an AI technology race, i.e., that we in the USA should mimic the Chinese approach. Number 1, we don’t share their values with respect to the balance of power between the individual and the collective. Number 2, we don’t share their enthusiasm for central authority. Number 3, “copy cat” is a losing strategy in business. We have to remain true to our ideals.
Some of the early criticisms regarding the establishment of AI principles across all sectors include the usual “lack of teeth” and mere “ethics whitewashing.” But, in the case of the DoD there’s an obvious contradiction or paradox in the notion of applying ethical principles to activities related to, as you put it, “killing people and breaking things,” regardless of what percentage of overall activities they represent. Did this come up for you during your time at the DoD and how so? Do you personally see a contradiction?
Many people view the DoD as a war machine, and they therefore label me as a warmonger. When I speak in public, I know those people are going to reject my messages, but I expect that and see it as the sign of a diverse audience–and that’s a good thing. I have a habit of starting speeches by saying, you’re not going to agree with a lot of things I say. I embrace that spirit of disagreement.
I was in high school when the Vietnam war was going on, and by my sophomore year, I was within 2 years of being eligible for the draft. I was taught that if you’re drafted and you didn’t go to war, you were a coward and a traitor. And if you weren’t drafted but you volunteered, you were an idiot.
The way I saw serving the DoD as CDO, it wasn’t about enabling people to kill, rather, it was to help defend the country against enemies. That said, we, meaning the US, have created most of those enemies in central Asia and the Middle East. And by ‘we’ I specifically mean the US government, not the US DoD. The DoD is an instrument of the Federal government. Our elected officials determine where and how the DoD operates.
In my role as a citizen, I work through the political process to shape the decisions our elected leaders make. In my role as CDO & CBAO I worked to make the DoD effective in carrying out the will of the elected leaders. There are elements of both roles that I’d say were problematic, but being an adult and a leader means deciding what problems you want to live with.
Michael Conlin is Chief Technology Officer at Definitive Logic.