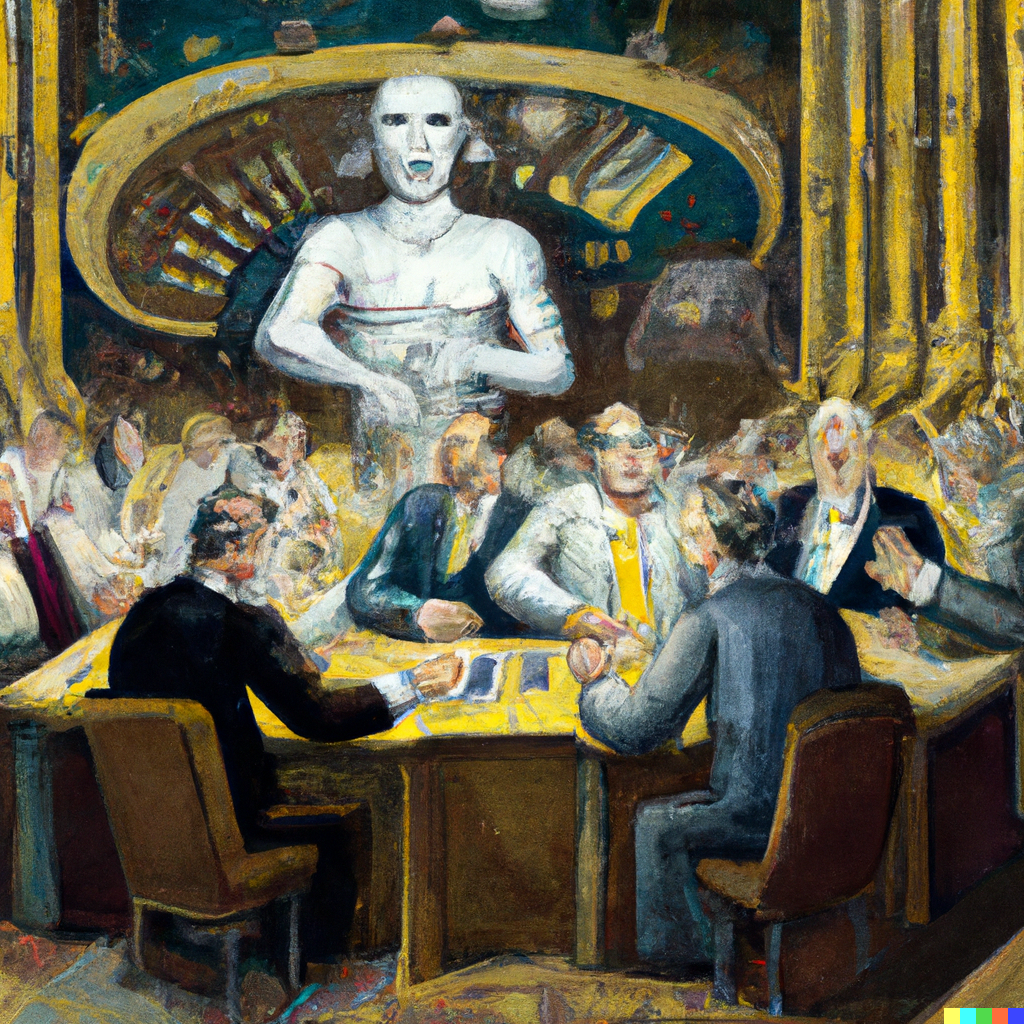
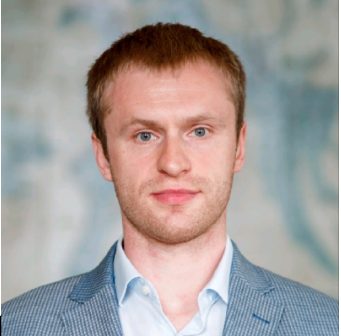
🔬 Research Summary by Risto Uuk, the EU Research Lead at the Future of Life Institute; Expert in the Working Group on Future of Work at Global Partnership on AI; International Strategy Forum Fellow at Schmidt Futures; and Expert in CEN and CENELEC AI standards working groups.
[Original paper by Risto Uuk, Carlos Ignacio Gutierrez, and Alex Tamkin]
Overview: Through its Artificial Intelligence (AI) Act, the European Union (EU) is seeking to regulate general-purpose AI systems (GPAIS). However, clear criteria to discriminate between fixed and general-purpose systems have yet to be formulated. This paper assesses different perspectives for determining what systems could be classified as GPAIS by examining four approaches: quantity, performance, adaptability, and emergence. Based on this work, we suggest that EU policymakers engage with these approaches as a starting point for determining the inclusion criteria for GPAIS.
Introduction
The EU has defined foundational terms to outline the AI Act’s scope properly. The definition of the term “AI” itself has been widely debated. On the one hand, certain interest groups have advised against a definition that excludes relevant systems. On the other hand, member states advocated for a narrower definition adopted by the Council in December 2022. Moreover, the European Parliament has recently adopted several terms this year, including GPAIS, foundation models, and generative AI.
The term GPAIS has not received as much attention as the definition of AI. Although this concept was rarely employed prior to the EU’s development of the AI Act, it is now a central idea for describing systems without a fixed purpose. As a technology, GPAIS are important because they include an increasingly powerful set of systems that are being widely deployed, such as ChatGPT, Bard, and Bing Chat. The EU’s proposed definition for GPAIS has faced criticism from external parties for being too broad in scope. Many stakeholders engaged in this process have proposed alternative definitions to better identify systems unconstrained by a fixed purpose (see Table 1).
The goal of this paper is to evaluate four approaches for identifying GPAIS. The first section summarises existing approaches to define GPAIS by the EU and external actors. It finds that a crucial common denominator between proposals is the need to clarify and operationalize what can be considered a unique purpose, herein discussed as “distinct tasks.” The second section discusses four approaches (quantity, performance, adaptability, and emergence) that stakeholders can use to make this differentiation. The last section examines the overarching role these approaches should play in the EU’s governance of AI.
Key Insights
Author | Proposed definition |
Draft EU Position | AI system that – irrespective of how it is placed on the market or put into service, including as open source software – is intended by the provider to perform generally applicable functions such as image and speech recognition, audio and video generation, pattern detection, question answering, translation and others; a general purpose AI system may be used in a plurality of contexts and be integrated in a plurality of other AI systems. |
Gutierrez et al., 2022 | An AI system that can accomplish or be adapted to accomplish a range of distinct tasks, including some for which it was not intentionally and specifically trained. |
Gahntz & Pershan, 2022 | AI systems that are provided without a specific intended purpose; instead, they can serve a large number of purposes, including purposes not foreseen or declared by their original providers. |
Engler & Renda, 2022 | AI systems characterized by their training on especially large datasets to perform many tasks, making them particularly well-suited for adaptation to more specific tasks through transfer learning. |
Campos & Laurent, 2023 | AI systems that can accomplish a range of distinct valuable tasks, including some for which it was not specifically trained. |
Moës, 2022 | • Preferred definition: General purpose AI systems are AI systems that score above x% on the EU standardized testing suite for generality administered by the European Benchmarking Institute.• OK/temporary definition: General purpose AI systems are AI systems that can be reasonably foreseen to carry out a broad range of tasks (e.g., ≥ 10) from the EU official list of tasks without substantial modification. |
Table 1: GPAIS definitions relevant to the EU context
The first approach to identifying a GPAIS is based on the number of distinct tasks a system performs. Moës (2022) suggests a two-pronged proposal using this line of thought. It begins by suggesting that the EU create a list of the distinct tasks an AI system can perform. Subsequently, policymakers would establish a threshold number of tasks to separate fixed and general-purpose systems. Because an official EU list does not exist, the EU standardization bodies could play a role in its creation.
A second approach to discriminating systems relies on measuring their effectiveness in completing distinct tasks. It might not be sufficient to ask how many tasks a model could theoretically perform because that casts too broad a net: for example, a rudimentary autocomplete system could, in theory, be used to write the rest of a complex report, but it might do so poorly. Instead, this approach focuses on discriminating between systems based on how well they perform different tasks based on agreed-upon metrics.
The third approach for characterizing GPAIS is to assess its ease of adapting to perform new distinct tasks. While both GPAIS and fixed purpose systems can execute at least one task, as the number grows, we can distinguish technologies by their ability to accomplish additional tasks they are applied to. For example, a facial recognition system, which is a fixed-purpose system, is not useful unless given an image, and its ability to recognize other objects is limited by its training. By contrast, GPAIS could learn to perform new tasks, such as classifying new objects. In practice, this adaptation is often done by conditioning and priming the GPAIS with examples of a task description or by modifying or fine-tuning its parameters.
The final approach for distinguishing a GPAIS is to discern a system’s potential for developing emergent abilities that enable it to perform distinct tasks. Emergence is when “quantitative changes in a system result in qualitative changes in behavior.” This means that certain systems can develop distinct emergent task abilities as their amount of computation, parameters, or training dataset size grows. For example, a 1B parameter model may not be able to accomplish tasks that the same architecture of a model with a 100B parameter count can. The same may be true for one trained with 10ˆ20 versus 10ˆ24 FLOPs.
Between the lines
We do not expect the Council or the Parliament to take a specific position during trialogue negotiations of the AI Act. When developing the final version of the AI Act, other EU stakeholders, especially the Commission and European standards bodies, should consider these four approaches (quantity, performance, adaptability, and emergence) when operationalising the inclusion and exclusion criteria for a GPAIS. Each has advantages and weaknesses that need to be addressed based on the EU’s ability to maximize the practicality, flexibility, and future-proofness of any selected approach. If an AI system surpasses a previously established and consented EU threshold for any of them, then the technology should be considered a GPAIS under the AI Act. However, while each approach alone could give reason to consider an AI system a GPAIS, they are best used holistically.