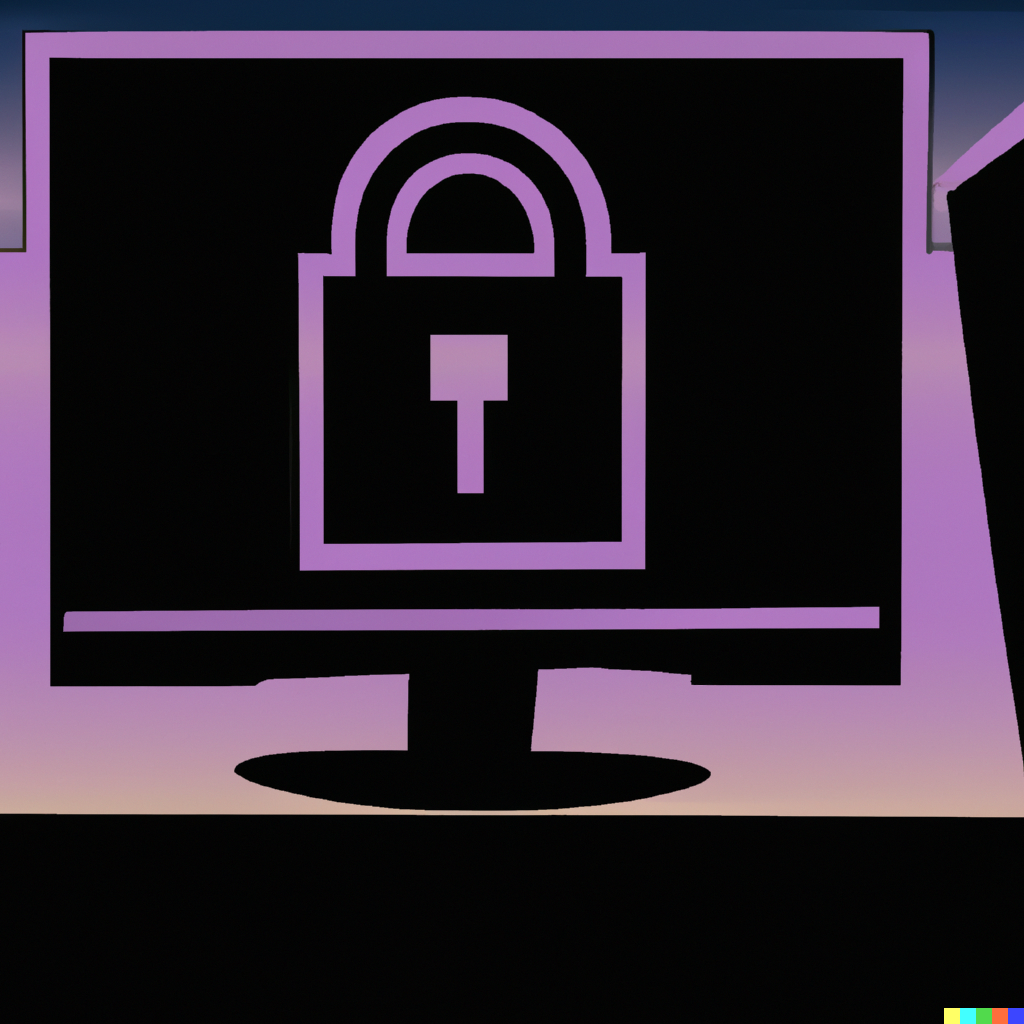
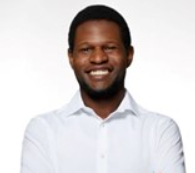
🔬 Research Summary by Lionel Tidjon, PhD is the Chief scientist & Founder at CertKOR AI and Lecturer at Polytechnique Montreal.
[Original paper by Lionel Tidjon and Foutse Khomh]
Overview: Bringing AI systems into practice poses several trust issues, such as transparency, bias, security, privacy, safety, and sustainability. This paper examines trust in the context of AI-based systems, with the aim to understand how it is perceived around the world, and then suggest an end-to-end AI trust (resp. zero-trust) model to be applied throughout the AI project life-cycle.
Introduction
AI can diagnose various types of cancer, such as brain, mammary, and pancreatic cancers, providing early detection and prognosis. However, an important question arises: Can we trust AI? This paper dives into the topic of ensuring the reliability of AI systems. It examines the concept of trust in AI-based systems and identifies gaps in our understanding of what makes AI truly trustworthy. By reviewing existing approaches, the researchers propose a trust model (and even a zero-trust model) for AI. They also outline a set of essential qualities that AI systems should possess to ensure their trustworthiness.
To enhance the trustworthiness of AI models, the researchers introduce helpful tools like VeriDeep, DeepZ, RefineZono, and RefinePoly. These tools enable us to verify the reliability of AI models and ensure they meet the necessary standards. As AI continues to revolutionize industries and impact our lives, it’s crucial to address the question of trust.
Key Insights
Can we trust AI systems?
This paper explores the question and discusses essential steps to ensure the reliability of AI-based technologies. In today’s world, where AI plays an increasingly prominent role, it’s crucial to examine how trustworthy these systems are. This paper investigates the concept of trust in AI and identifies gaps that challenge our understanding of its reliability.
The paper reviews different approaches proposed to ensure the trustworthiness of AI systems. It also introduces a new trust and zero-trust model for AI based on ideal qualities discussed in previous studies. By building upon existing research, this model aims to redefine our perception of trustworthy AI. The context of this study is a set of 100 documents containing 100 trustworthy properties in the six continents (e.g., fairness, transparency) gathered online from reports of national/international organizations. The data sources are selected based on their reliability, recency, and diversity. The paper uses a literature review approach to examine existing approaches proposed for ensuring the trustworthiness of AI systems and identify potential conceptual gaps in understanding what trustworthy AI is.
However, there are contentious issues that need attention. Defining what trustworthy AI means poses challenges due to the lack of a unified definition. This gap necessitates further exploration. Additionally, we must consider the ethical, legal, and societal impacts of AI, which remain uncertain. To guarantee responsible AI implementation, more research in these areas is crucial. Furthermore, the paper highlights the difficulties in auditing and certifying AI systems. Their opaque nature and vulnerability to threats make it challenging to ensure their reliability. Addressing these challenges calls for continued research and innovation in the development, auditing, and certification processes for AI systems.
Are you curious to uncover the truth about trustworthy AI?
The findings of this paper will transform the understanding of AI’s trustworthiness while shedding light on the gaps that need urgent attention.
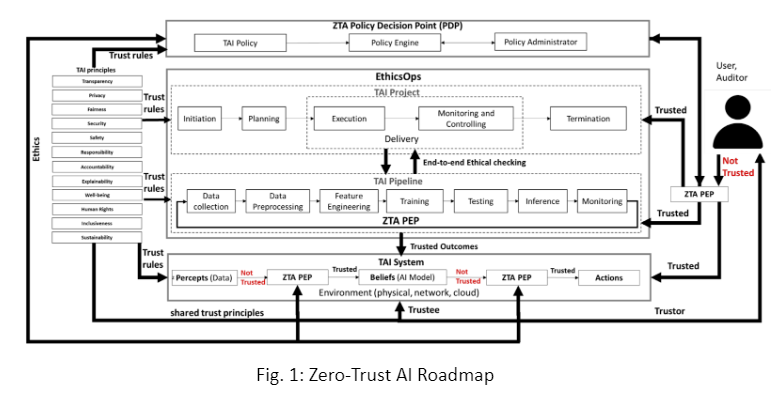
The paper introduces a cutting-edge trust and zero-trust model for AI (see Fig. 1). Building upon the ideal properties discussed in previous studies, this model lays a roadmap to foster trust in AI systems. By incorporating these essential properties, organizations can develop and implement AI systems that are transparent, accountable, and dependable. The zero-trust AI (ZTA) model consists of six components: human (trustor), AI system (trustee), Trustworthy AI (TAI) principles, Policy Enforcement Point (PEP), Policy Decision Point (PDP), and EthicsOps. ZTA PEP enables, monitors, and closes trust relationships between the trustor and the trustee. It interacts with a policy administrator responsible for establishing and/or stopping trust relationships between parties based on the feedback from the policy engine. ZTA PDP takes an immediate decision based on instructions received from the policy administrator in the ZTA PEP component. EthicsOps ensures the continuous monitoring of these actions to identify any deviation in trust so that failing or untrusted components can be updated to move the system into a trust state.
Between the lines
In conclusion, this research summary highlights the complexities of trust in AI systems and presents a roadmap for building trustworthiness. It challenges us to question and verify AI’s reliability rather than relying on blind trust. The findings have far-reaching implications for governments, organizations, and individuals. By following this roadmap, we can navigate the evolving landscape of AI and shape a future where trustworthy AI systems inspire confidence and ethical decision-making.