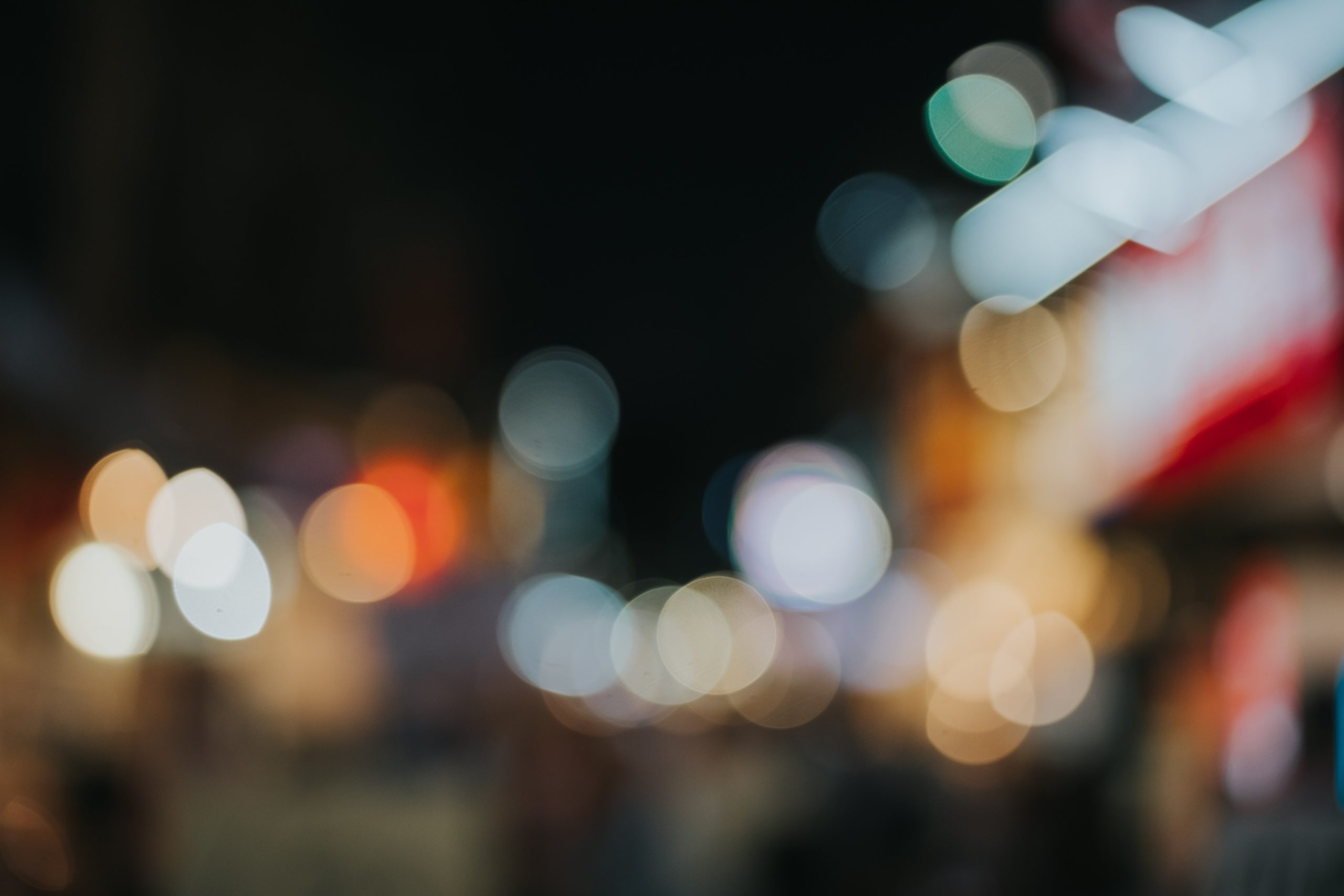
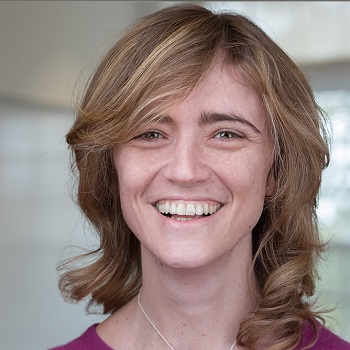
š¬ Research summary by Dr. Marianna Ganapini (@MariannaBergama), our Faculty Director.
[Original paper by Steven Umbrello, Ibo van de Poel]
Overview: Value sensitive design (VSD) is a method for shaping technology in accordance with our values. In this paper, the authors argue that, when applied to AI, VSD faces some specific challenges (connected to machine learning, in particular). To address these challenges, they propose modifying VSD, integrating it with a set of AI-specific principles, and ensuring that the unintended uses and consequences of AI technologies are monitored and addressed.
Introduction
How do we bridge theory and practice when it comes to following ethical principles in AI? This paper aims at answering that very question by adopting Value sensitive design: a set of steps to implement values in technological innovation. Value sensitive design potentially applies to a vast range of technologies, but when used in AI and machine learning, it inevitably faces some specific challenges. The authors propose a way to fix these problems by integrating Value sensitive design with other actionable frameworks.
Key Insights
- Value sensitive design (VSD)
Value sensitive design (VSD) is a method originally developed by researchers at the University of Washington and it lays out actional steps for designing technology in accordance with our values. These steps are grouped in three main categories: conceptual, empirical and technical investigations. Conceptual analysis determines the appropriate set of values (coming from the philosophical literature and/or from the stakeholdersā expectations), whereas empirical investigations may survey direct and indirect stakeholders to understand their values and needs. The third set of steps looks into potential technical limitations and resources to design a technology following the appropriate set of values.
Unfortunately, the self-learning capabilities of AI pose some specific challenges for VSD. Notoriously, models developed through machine learning can have features that were not initially designed or foreseen, and some of these features may be opaque and thus not easily detectable. This could mean that AI systems, originally designed following VSD, āmay have unintended value consequences, […] or unintentionally ādisembodyā values embedded in their original design.ā As the authors explain, this means that we need design principles specific for this kind of technology and expand VSD to address those challenges. The question is how to do that.
- Solutions
The authors propose to modify VSD in the following three ways: (1) VSD should include a set of AI-specific principles (AI4SG); (2) for VSD, the goal should be not only to promote outcomes that avoid harming but also to contribute to social good overall; (3) VSD should look at the downstream consequences of adopting a certain AI system to make sure the designed values are in fact respected.
2.1 VSD & AI4SG
Letsā start witht the first point. The authors propose to adopt AI-specific principles in VSD. In particular, they look at AI4SG (AI for social good) principles, which are actionable guidelines, inspired by the more high level values of ārespect for human autonomy, prevention of harm, fairness, and explicabilityā. These are the principles:
ā(i) falsifiability and incremental deployment; (ii) safeguards against the manipulation of predictors; (iii) receiver-contextualised intervention; (iv) receiver-contextualised explanation and transparent purposes; (v) privacy protection and data subject consent; (vi) situational fairness; and (vii) human-friendly semanticisation.ā
The authors of the paper point out that applying these specific principles in the design of AI systems would address some of the concerns mentioned above. This is because these steps are not only more practical than the high-level values but they are also specific to AI and so are the right tools to avoid the challenges raised by this kind of technology. These principles are, in other words, a more concrete application of the key values (e.g. beneficence) we want to see as part of the design of AI going forward.
2.2 VSD & the social good
Hereās the second issue: VSD should be not only to promote outcomes that avoid doing harm but also to contribute to social good and so āthere must be an explicit orientation toward socially desirable ends.ā To promote this, the authors recommend that VSD adopts āthe Sustainable Development Goals (SDGs), proposed by the United Nations, as the best approximation of what we collectively believe to be valuable societal endsā. Again, this is a matter of complementing and enriching VSD with a set of principles that actively try to promote social good, and as such, they should be part of the design of AI systems.
2.3 VSD and downstream consequences
Finally, ongoing monitoring is needed to address possible unintended consequences of adopting AI systems. Indeed, when employed, AI systems may not respect the original design values (see here for more). This is why there is the need to apply VSD to the entire ālife cycle of an AI technologyā, monitoring systems, and adopt the necessary design changes when needed. The authors point out that prototyping and small scale testing could really help address unforeseen consequences.
By combining these principles and ideas, the authors embrace a framework that encompasses the following recursive loop:
Context Analysis (e.g. societal challenges, values for stakeholders) ā Value Identification (e.g. beneficence, autonomy, SDGs, case specific values) ā Design Requirements (e.g. AI4SG), ā Prototyping (e.g. small-scale testing)
This proposed framework is meant to be taking into account the various aspects of VSD while also addressing some of its shortcomings.
Between the lines
It is important to find a way to bridge theory and practice when it comes to building ethical AI systems. This paper is charting a way forward to address this need. It brings together different methods and approaches by explaining how to integrate action steps within the VSD framework while also making sure social good is taken into account. Now that we have a fairly comprehensive set of high-level values, future research will need to establish more precise, actionable and concrete steps to embody those values within AI systems, and it will need to find new ways to determine the ethically relevant, downstream consequences of the use of those systems.