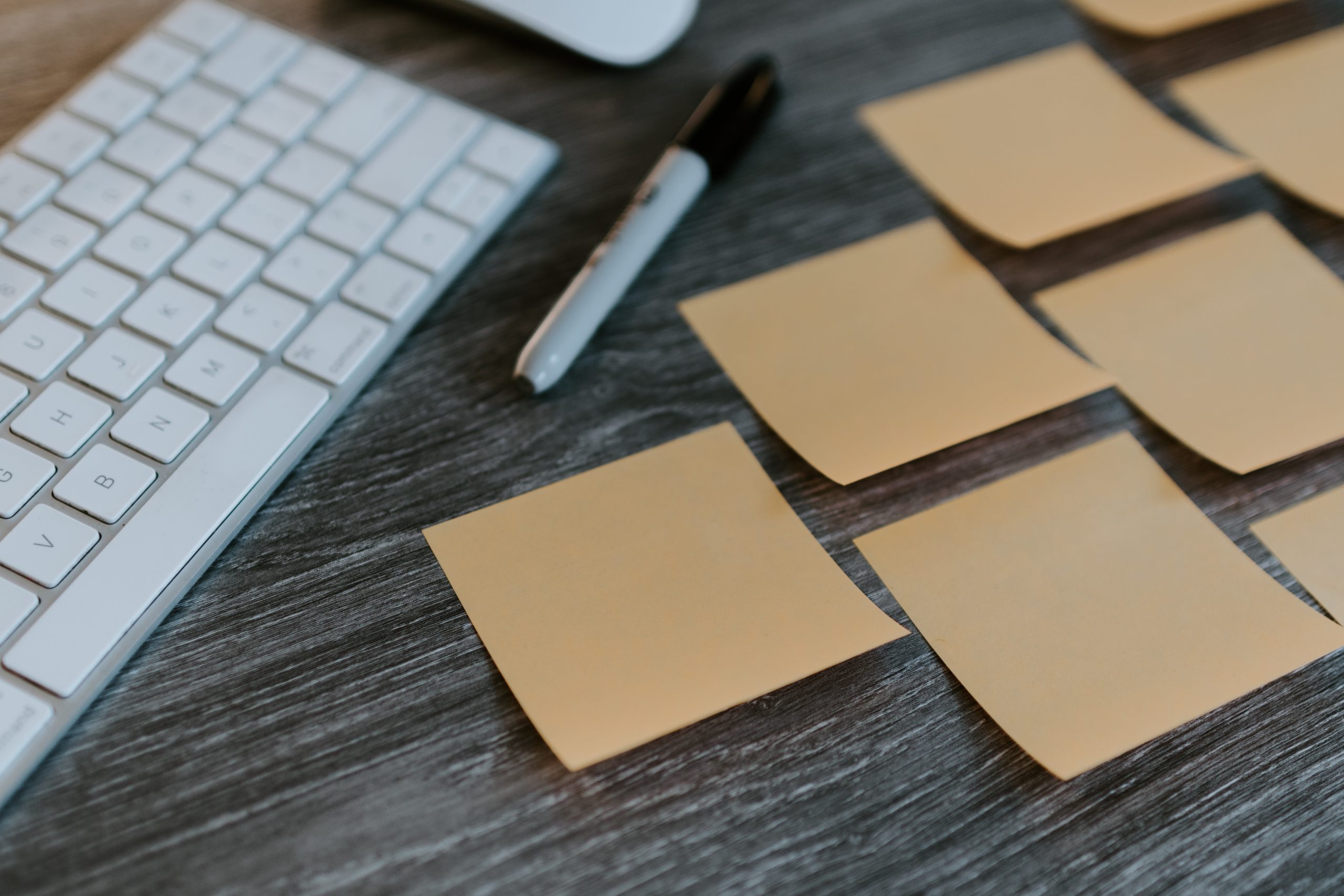
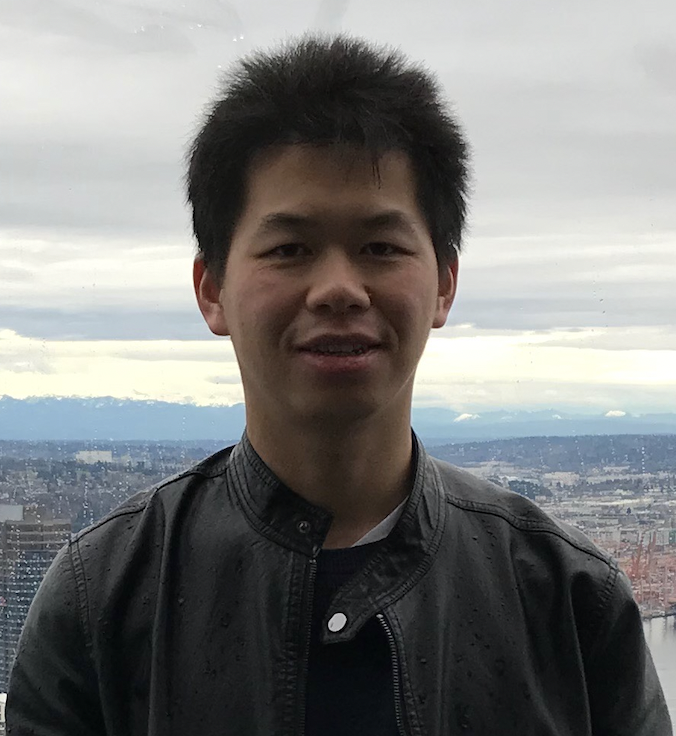
đŹ Research Summary by Wenbin Zhang, a Postdoctoral Researcher at Carnegie Mellon University tackling important social, environmental, and public health challenges that exist today using AI, a.k.a. AI for Social Good.
[Original paper by Wenbin Zhang and Jeremy C Weiss]
Overview: AI fairness has gained attention within the AI community and the broader society beyond with many fairness definitions and algorithms being proposed. Surprisingly, there is little work quantifying and guaranteeing fairness in the presence of censorship. To this end, this paper rethinks fairness and reveals idiosyncrasies of existing fairness literature assuming certainty on the class label that limits their real-world utility.
Introduction
Recent works in artificial intelligence fairness attempt to mitigate discrimination by proposing constrained optimization programs that achieve parity for some fairness statistic. Most assume availability of the class label, which is impractical in many real-world applications such as precision medicine, actuarial analysis and recidivism prediction. In this paper, we consider fairness in longitudinal right-censored environments, where the time to event might be unknown, resulting in censorship of the class label and inapplicability of existing fairness studies. To this end, we devise applicable fairness measures, propose a debiasing algorithm, and provide necessary theoretical constructs to bridge fairness with and without censorship for these important and socially-sensitive tasks.Â
Key Insights
AI FairnessÂ
While artificial intelligence is increasingly permeating facets of life, significant concerns on the unfair and discriminatory manner of AI-based systems have been voiced and observed. Because AI-based decision-making can be as biased as human and can even exacerbate disparity, there is an urgent need to consider fairness in AI algorithms in order to maximize AI benefits for social good.
This has led to an active area of research into quantifying and mitigating AI unfairness for the sake of providing fairness-aware decision-making systems, i.e., systems that are not unduly biased for or against certain individuals or social groups. Note that most of the work in the existing literature tackles the fairness problem by assuming the presence of class label, in which the fairness notions are defined based on the class label either actual or predicted, and the same predictive model is trained contingent upon for new instance prediction.
Censorship PhenomenonÂ
In this work, we consider such a censorship setting where the true time to the event of interest, i.e., class label, might be unknown to the learner, while fulfilling the requirements of fair and accurate predictions. This censoring phenomenon can arise in various ways. For example, a study may end while an individual has not yet experienced the event of interest. This data is censored because each individual may eventually experience the event of interest but such information is not present. In another case, the studied individual can be lost to follow-up during the study period, withdraw from the study, or experience a competing event making further follow-up impossible.
Such a censoring phenomenon widely exists in many real-world applications as well as fairness benchmark datasets. For example in clinical prediction in which the patient’s true time to relapse or hospital discharge could be unknown for various reasons mentioned above. So are predicting reoffending in recidivism prediction, analyzing financial outcomes in actuarial analysis, and predictive maintenance in mechanical operations, to name a few. Due to the incapability of handling censorship information, existing fairness studies quantify and mitigate bias focusing on the certainty proportion of these fairness tasks by either dropping observations with uncertain class labels due to censorship or further getting rid of the censorship information of these instances. However, both of which contain important information and removing them would bias the results towards all observations in the study including instances with certainty on their class labels.
Quantifying and Mitigating Unfairness with CensorshipÂ
The aforementioned critical observations of AI unfairness reveal a significant idiosyncrasy of existing fairness studies that limits their real-world utility. Armed with this broader observation of AI unfairness and to fill the gap between AI fairness and real-world applications, this work proposed two first of its kind censored fairness notions, one new debiasing algorithms along with a theoretical framework to specifically account for fairness under censorship. More specifically:
- This work rethinks fairness and formulates a new problem of fair decision-making with censorship. Then, we devise corresponding fairness definitions to measure unequal treatments in the presence of censorship and attribute with non-binary representation and different semantic meanings, thus providing necessary complements to existing fairness definitions in the literature.
- One respective fairness-aware learner is developed for learning with censored data that are common in many real-world applications. The proposed learner specifically accounts for negligible censorship information in the model building so as to ensure accurate predictions while minimizing unfairness in censoring settings.
- A theoretical analysis to establish the connections of fairness in censored and uncensored settings, offering greater understanding and explanation for AI fairness. Such an analysis enables model-agnostic evaluation of fairness and helps practitioners navigate through their business needs.
Between the lines
Despite the increasing attention on AI fairness, existing studies have mainly focused on no censorship settings with certainty on the class label. This paper tackles fairness with censorship which is particularly prevalent in many real-world socially-sensitive applications. To accomplish this objective, we devised generalized censored-specific fairness notions to quantify unfairness along with a unified debiasing algorithm to mitigate discrimination in the presence of censorship. The proposed technique is expected to be versatile in alleviating bias in various socially-sensitive applications (e.g., the allocations of health resources, personalized marketing and recidivism prediction instrument). In addition, this work studies a new research problem and opens possibilities for future work on AI fairness with a broader applicability to practical scenarios concerning fairness.