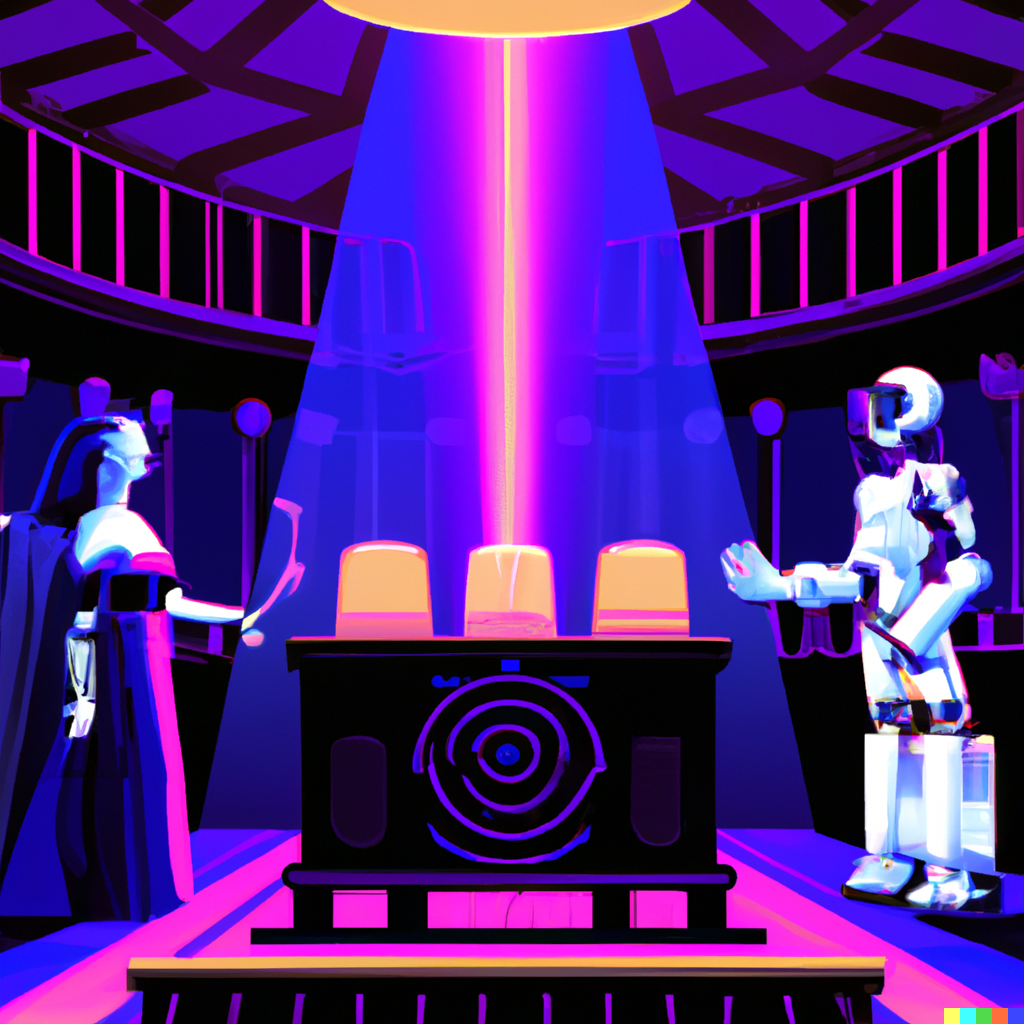
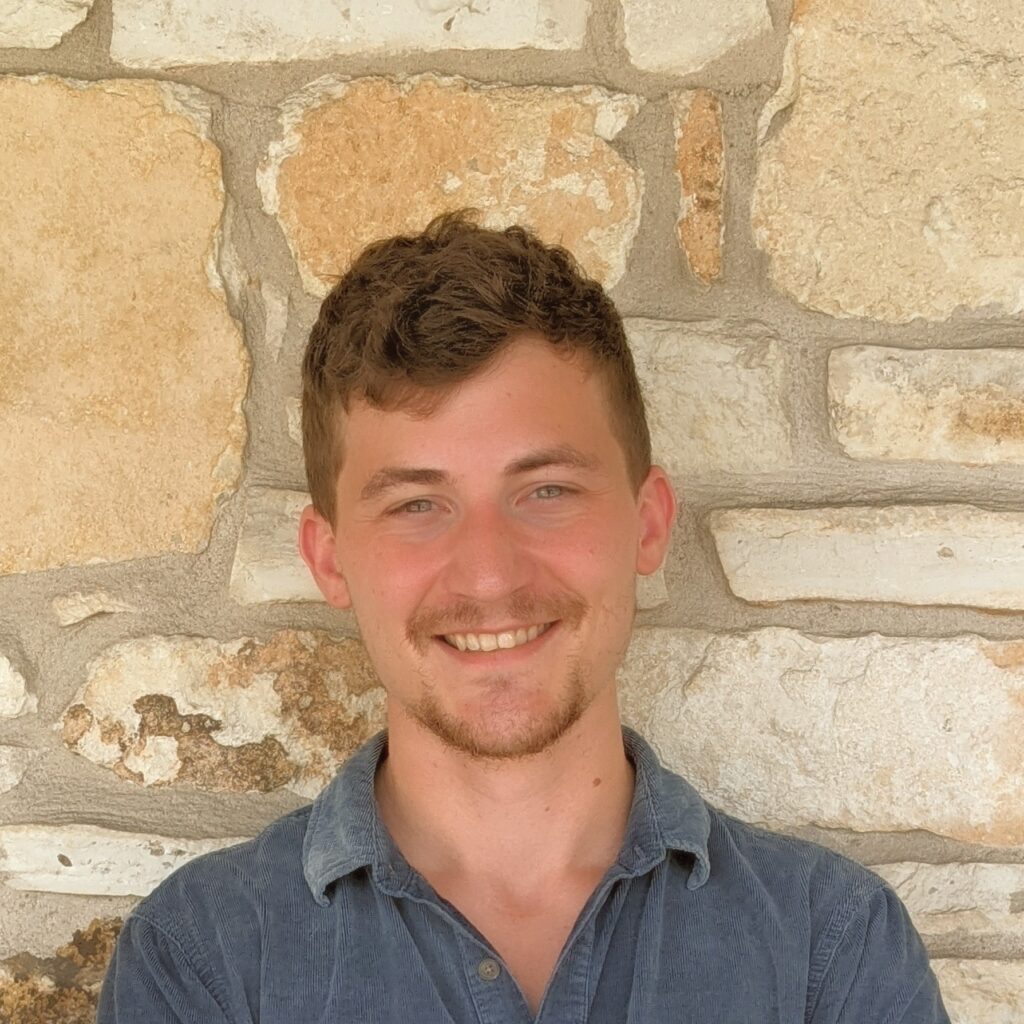
🔬 Research Summary by Terrence Neumann, a second-year PhD student researching ethical, behavioral, and engineered components of trustworthy AI systems, especially as it relates to misinformation detection and medicine.
[Original paper by Terrence Neumann, Maria De-Arteaga, and Sina Fazelpour]
Overview: Despite their adoption on several global social media platforms, the ethical and societal risks associated with algorithmic misinformation detection are poorly understood. In this paper, we consider the key stakeholders that are implicated in and affected by misinformation detection systems. We use and expand upon the theoretical framework of informational justice to explain issues of justice pertinent to these stakeholders within the misinformation detection pipeline.
Introduction
Misinformation and disinformation pose unique and widespread threats to institutions and individuals. In response to these threats, algorithmic detection tools have been adopted by some platforms. For instance, Facebook reportedly labeled 180 million messages as potentially misleading during the 2020 election season, while Twitter says it marked 300,000 during the same period. Given that no system is perfect, these systems will eventually err and mislead individuals or groups. How, then, should we consider the ethical consequences of errors made by these systems on platforms with billions of users? Who is likely to be affected by these errors? How do these errors arise?
There has been no systematic study of the types and sources of injustice that can plague automated misinformation detection systems. This gap is problematic not only because of what is at stake in how we devise and evaluate sociotechnical responses to misinformation; it is troublesome mainly because the frameworks of justice that have been widely applied in the context of algorithmic justice and that are suitable for allocation settings (such as the provision of medical care and hiring, for instance) are not sufficient to capture all dimensions of justice in information ecosystems.
In this paper, we introduce a multi-dimensional framework to understand (1) the stakeholders better and (2) the sources of injustice involved in misinformation detection by building on the notion of informational justice, first proposed by philosopher Kay Mathiesen. As we discuss, this framework allows us to adequately understand the variety of stakeholders that may be affected by the quality of a misinformation detection system.
Key Insights
An Illustrative Example of Informational Justice
Imagine that you have traveled back in time, only to scroll through 2016 Twitter for some reason, and you see a particular tweet that is spreading rapidly across the site: “Pope Francis endorses Donald Trump for President.”
The informational justice framework can be used to understand the players affected by this (mis)information. The seeker of information is anyone actively searching for information regarding a given topic. In this example, that may be American voters or Catholics looking for information about an upcoming election. The subject of the information is the individual or group represented within the tweet: Pope Francis and Donald Trump. It might also incorporate particular identities, such as Catholics (in relation to Pope Francis) and Republicans (regarding Donald Trump). The source of information is the user that tweets or otherwise posts information. These would be the accounts responsible for the spread of (mis)information. Finally, misinformation detection involves a fourth party – the source of evidence – which is likely a fact-checker or primary source that can refute or verify a claim with supporting evidence. In the context of the tweet above, reporters from Snopes and other fact-checking websites debunked this claim. Figure 1 summarizes and generalizes these relationships between stakeholders and two informational items (a claim and evidence regarding the claim).
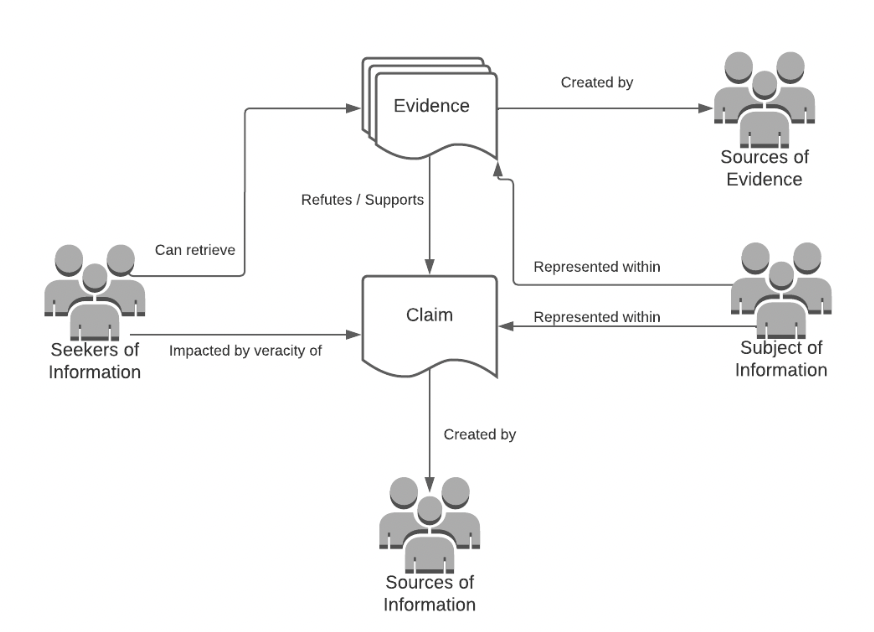
Knowing the stakeholders helps us to distinguish and clearly articulate the sense of justice to these stakeholders. Mathiesen proposed three of these notions in her original work in relation to (mis)information, but the context of misinformation detection requires revision. First, if a group of seekers of information systematically receive a lower quality of information than others due to a biased misinformation detection system, then these seekers would face a distributive injustice in the quality of information they receive. If certain groups of sources of information are unfairly moderated, having their content blocked at higher rates, then this forms a participatory injustice rooted in notions of “free speech.” Suppose certain groups of subjects of information (or those identifying with subjects of information based on their identity or experiences) are the targets of damaging misinformation at higher rates than others. In that case, this constitutes a recognitional injustice, as their reputation or identity is unfairly diminished. Finally, if some sources of evidence regarding a claim are systematically and unduly deemed less credible than other sources of evidence, this constitutes an epistemic injustice.
Embedding Informational Justice in the Pipeline
A recent literature review identified three key machine learning tasks that organizations may use to automate misinformation detection. It’s worth noting that systems in practice may only implement one or two elements of this pipeline and keep human workers for the other segments. First, a “check-worthiness” algorithm scans a vast array of user content and determines which content is most “worthy” of fact-checking. Second, if a claim is prioritized for fact-checking, evidence that either supports or refutes the claim is retrieved. This evidence can either be a direct survey regarding the claim or by passively gathering previously generated evidence. Third, after a claim has been prioritized for fact-checking and evidence is retrieved, a predicted verdict (false, mostly false, etc.) can be generated.
Let’s focus now on the first stage in the pipeline – checkworthiness. As I previously mentioned, these algorithms help prioritize scarce human fact-checking resources for the most “important” claims made on social media. Drawing a connection to our example tweet, this is likely very check-worthy for Americans, especially if it was released with the intent to influence an election. Large social media platforms like Meta use check-worthiness to prioritize fact-checking. Several different methods have been proposed to predict the check-worthiness of a claim. However, they all involve estimating a function dependent on the content of the claim and the content metadata of a claim, such as likes, retweets, the source of information, etc.
At this stage of the pipeline, we see that the most salient harms stem from false negatives (i.e., when something is check-worthy but isn’t prioritized for fact-checking). In this case, subjects of information can harm their reputation (i.e., recognitional injustice) if misinformation or rumor isn’t prioritized for fact-checking, and seekers of information interested in high-quality information will face a distributive injustice in allocating fact-checking resources. This is especially important in high-stakes scenarios, such as elections and humanitarian crises. Interestingly it’s not clear if false positives (i.e., prioritizing a claim for fact-checking that isn’t particularly relevant) harm stakeholders as long as false positives in this stage are uncorrelated to errors further down the pipeline.
So where might these harmful false negatives come from in the check-worthiness pipeline? In the context of supervised check-worthiness algorithms, they can be at least partially attributed to measurement bias in crowd-sourced labels. For instance, there is evidence in research and practice that people ask questions about the relevance of the claim to the “general public” when surveying the crowd for check-worthy information. This definition might bias the labels to systematically down-weight check-worthiness of claims made about marginalized groups. To alleviate measurement bias in the labels, we see some guidance from the automated toxicity scoring literature which states that “using raters that self-identify with the subjects of comments can create more inclusive machine learning models, and provide more nuanced ratings than those by random raters.”
In subsequent sections of the full paper, we analyze senses and sources of harm relevant to stakeholders in the other two stages of the misinformation detection pipeline: evidence retrieval and verdict prediction.
Between the lines
I want to discuss some implications of this work. First, on the more theoretical side, this work highlights that sometimes, to benefit one player within a misinformation detection system, a burden is placed on another. These tradeoffs can be encoded as organizational values. What values are encoded in real-world systems, and who is most often valued/devalued? For instance, on Facebook, conservative sources of information claimed that they were scrutinized more closely than liberal sources of information. In response, Facebook changed its standard of detection only for conservative sources. However, this seems to imply that their claim to justice is more pertinent than that of conservative information seekers, who will now face higher levels of misinformation in their newsfeeds. Future theoretical work could examine these issues in more detail.
Finally, more practically, this analysis provides a strong ground for algorithmic fairness audits of misinformation detection systems in relation to one or more stakeholders. By providing a coherent, multi-dimensional taxonomy and drawing connections to sources of algorithmic bias, we hope those conducting these audits will examine fairness as it relates to all stakeholders and not focus exclusively on myopic notions of justice, such as freedom of speech.