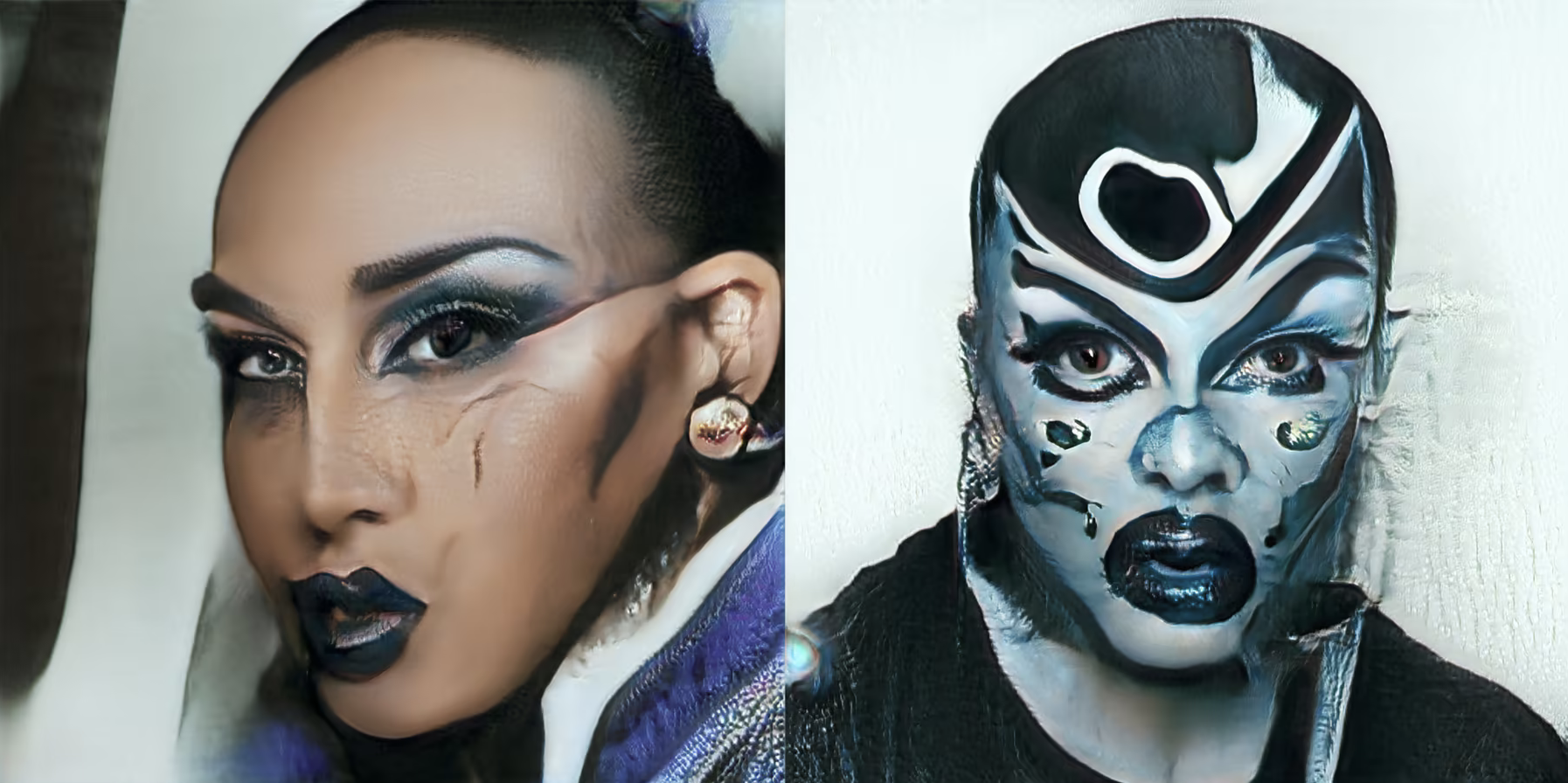
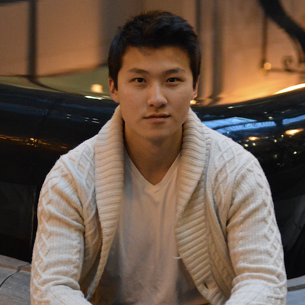
AI Application Spotlight by Jimmy Huang (@HuangWrites), AI Ethics Researcher and an innovation leader within the Financial Technology space, delivering ethical enterprise data systems for international banks and stock exchanges.
“The idea behind latent space is that there’s this continuous space between the classes. You have these multi-dimensional vectors which relate everything it [the artificial intelligence] has learned about, say, a female face as well as everything it has learned about a male face, and there’s this continuous space in between. It doesn’t actually have those gendered binaries anymore – it’s a continuation, and with unsupervised learning it doesn’t even have the gendered labels…”
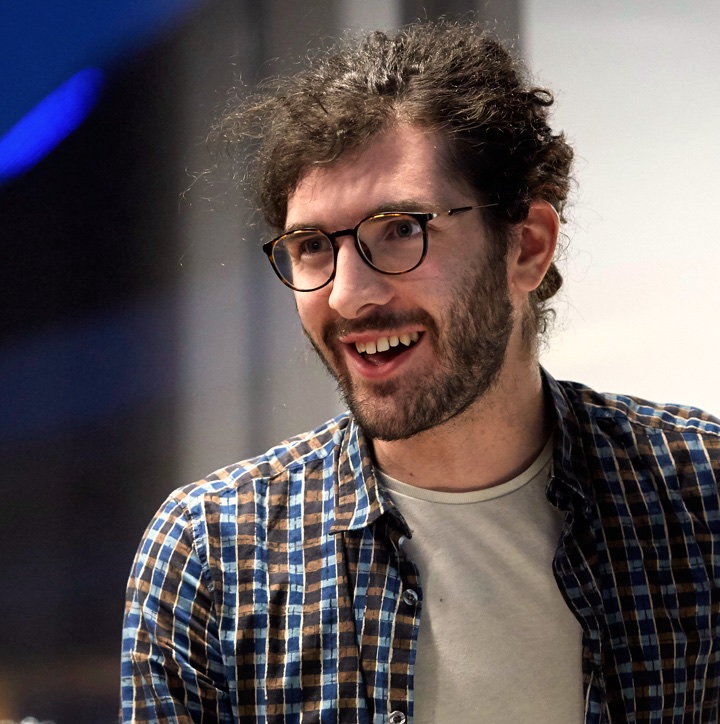
In the burgeoning field of artificial intelligence (AI) ethics, researchers at the Montreal AI Ethics Institute have been analyzing how AI applications frequently learn discriminatory behaviour from being fed biased training datasets. This could be, for example, from a lack of inclusion in the training set resulting in an application’s inability to detect the faces of minorities [1] to an overinclusion within other sets for the express purpose of surveilling certain minority groups. [2]
There are also statistically significant, yet, barely perceptible biases we can only uncover through careful research such as when using historical US mortgage data to predict creditworthiness. Using standard logistic regression and Random Forest models, Fuster et al.’s CEPR discussion paper concludes: “minority groups appear to lose, in terms of the distribution of predicted default propensities, and in our counterfactual evaluation, in terms of equilibrium rates…” [3]
All this is to say that without guidance from ethics, by the very nature of training sets requiring bias to perform, discriminatory behaviour will not only persist but increase in ubiquity enhanced with modern, far-reaching technology.
Enter London-based artist, Jake Elwes. Carrying a strikingly warm presence, Elwes takes a seat across from me at Ditto Coffee in Shoreditch for the interview.
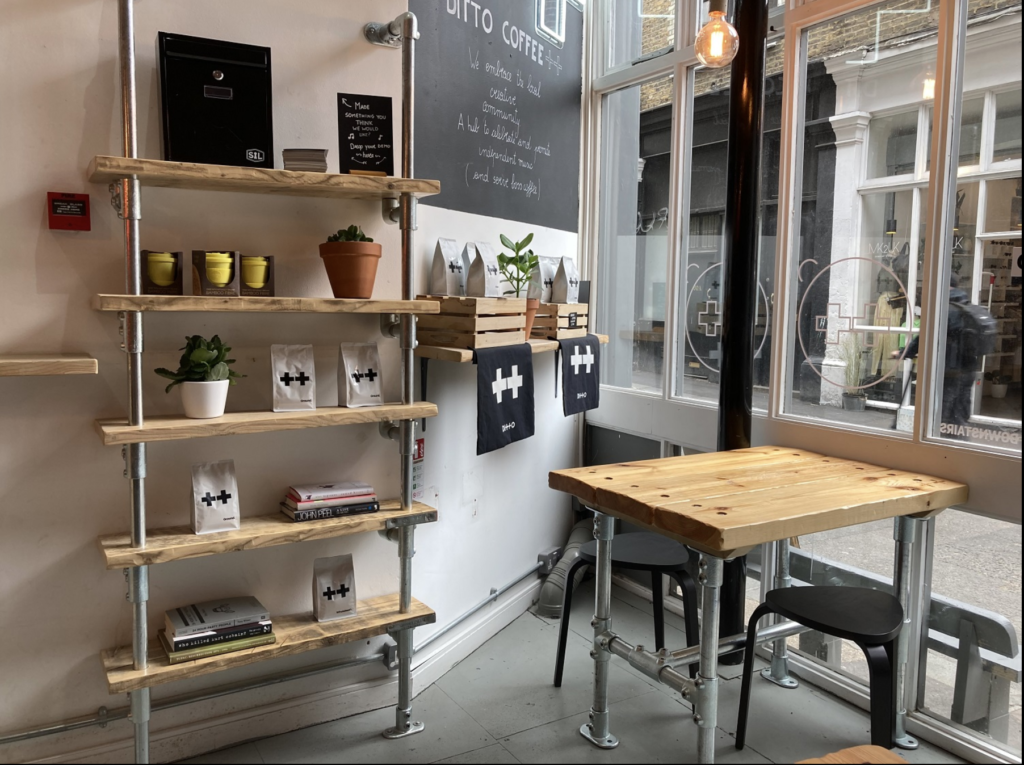
For the past half-decade, Elwes has been using various machine learning techniques to generate media art that compels us to consider our place in society giving a unique perspective in viewing human identity through the lens of modern technology.
Elwes’ latest venture, The Zizi Project, is an ongoing series of works applying diverse drag and gender fluid identities as training sets for positive AI outcomes. The project started with the “Zizi – Queering the Dataset” installation in 2019 where an AI program attempts to constantly generate, shift, and regenerate non-binary faces in a work that celebrates difference and ambiguity. In 2020, Elwes produced the “Zizi & Me” installation, a double act between London drag queen Me [4] and a deep fake (AI) as well as “The Zizi Show” [5], a deep fake drag cabaret featuring a number of acts.
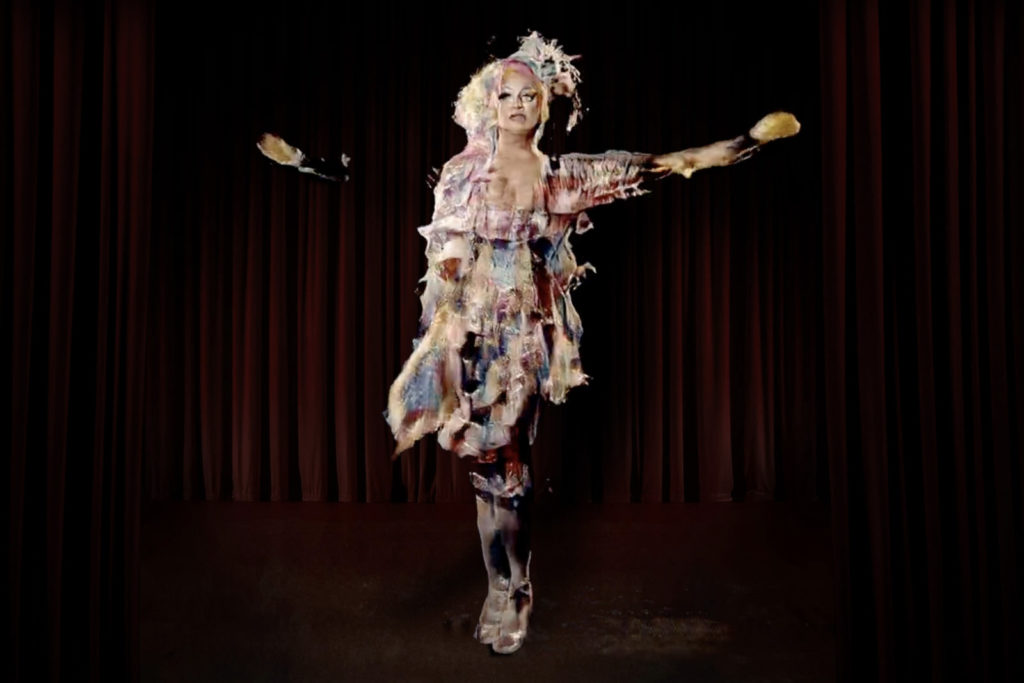
Elwes has taken a look at all emerging Generative Adversarial Networks (GAN) techniques and wonders ‘how can we use this as a performance tool?’. “The Zizi Project” explores the effects of technology on gender identity through performance and in the process of creating the show, a variety of ethical topics are brought to light within the confines of a safe environment. Elwes leans forward over the table between us and, with passion, explains the discourse within both the drag and transgender communities around data consent, namely, how an individual’s image may be used as well as what the use would be for.
On the data consent side, Elwes ensures that the performers who contributed visual content to “The Zizi Show” and “Zizi & Me” will have control over their image. They can retract their likeness from training sets and have derived performances from their likeness taken down. However, a much more interesting concern is brought forth by whether the inclusion of queer identities in training datasets have inherent issues. Some may posit that since we live in a technology-driven world, real harm could arise due to, for example, doctors not having the proper data points to adequately come up with treatments for transgender physiologies. On the other side, Elwes explains, “there is a real pride to being queer and having this otherness” and, historically speaking, marginalized communities are right to be wary of how changing technological and societal landscapes affects them. Given this pride in othernness, some members of the queer community feel hesitant to be included in training datasets or in having their identities assimilated to an extent.
Elwes aims to honour underrepresented and historically marginalized non-binary groups while also creating a uniquely charming cabaret show. In creating the show, from a technological standpoint, Elwes was largely inspired by the idea behind latent space.
“There’s a queerness to latent space”
In simple terms, latent space is this hidden world, opaque to human intuition, of compressed data where similar features are mapped closer together.
Data is only useful insofar as there is bias in the set. Without bias, data would either be completely random or, on the other side, uniform and therefore largely useless. Machine learning applications find similarities in features by first compressing data into latent space, a vector space represented mathematically, and then in grouping similar data points closer together according to meaningful features.
There’s ambiguity and nigh-infinite spectrums of data groupings that may be applied in a variety of contexts hidden within latent space. In this way, the vague, unlabelable inner-mechanism of how deep learning works have profound parallels to the gender fluidity of non-binary identities. It is especially fascinating how a non-binary group of identities is, in turn, grouped within latent space on a previously undefined spectrum.
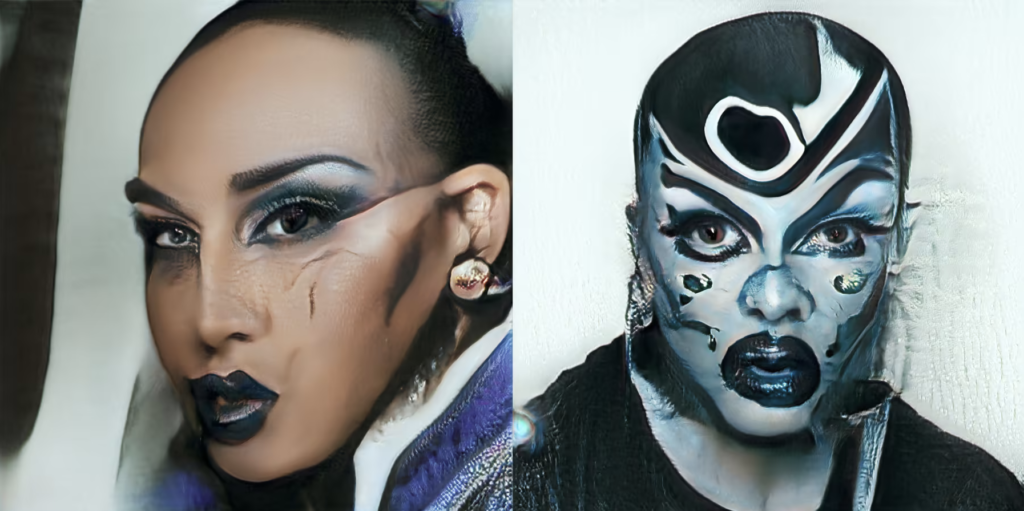
Elwes’ works aim, in part, to deconstruct gender and then reconstruct the features in an ever-transitory state. The gender-fluid appearances are distilled into groupings hidden within latent space and then constantly reconstructed becoming an evolving spectrum of an input set that is already a spectrum of gender identities.
In a world where we’re constantly inundated with articles on the negative effects that AI applications may have on society at-large, seeing a positive outcome for a historically marginalized group, if only for cultural and artistic insight, is a breath of fresh air. Elwes works at the frontier of this innovative space, using emerging generative adversarial networks and deep fake techniques as they’re discovered to create thoughtful, ethical art.
References
[1] https://news.mit.edu/2018/study-finds-gender-skin-type-bias-artificial-intelligence-systems-0212
[2] https://www.nature.com/articles/d41586-020-03187-3
[3] https://papers.ssrn.com/sol3/papers.cfm?abstract_id=3072038
[4] https://www.instagram.com/methedragqueen/?hl=en
[5] https://zizi.ai/