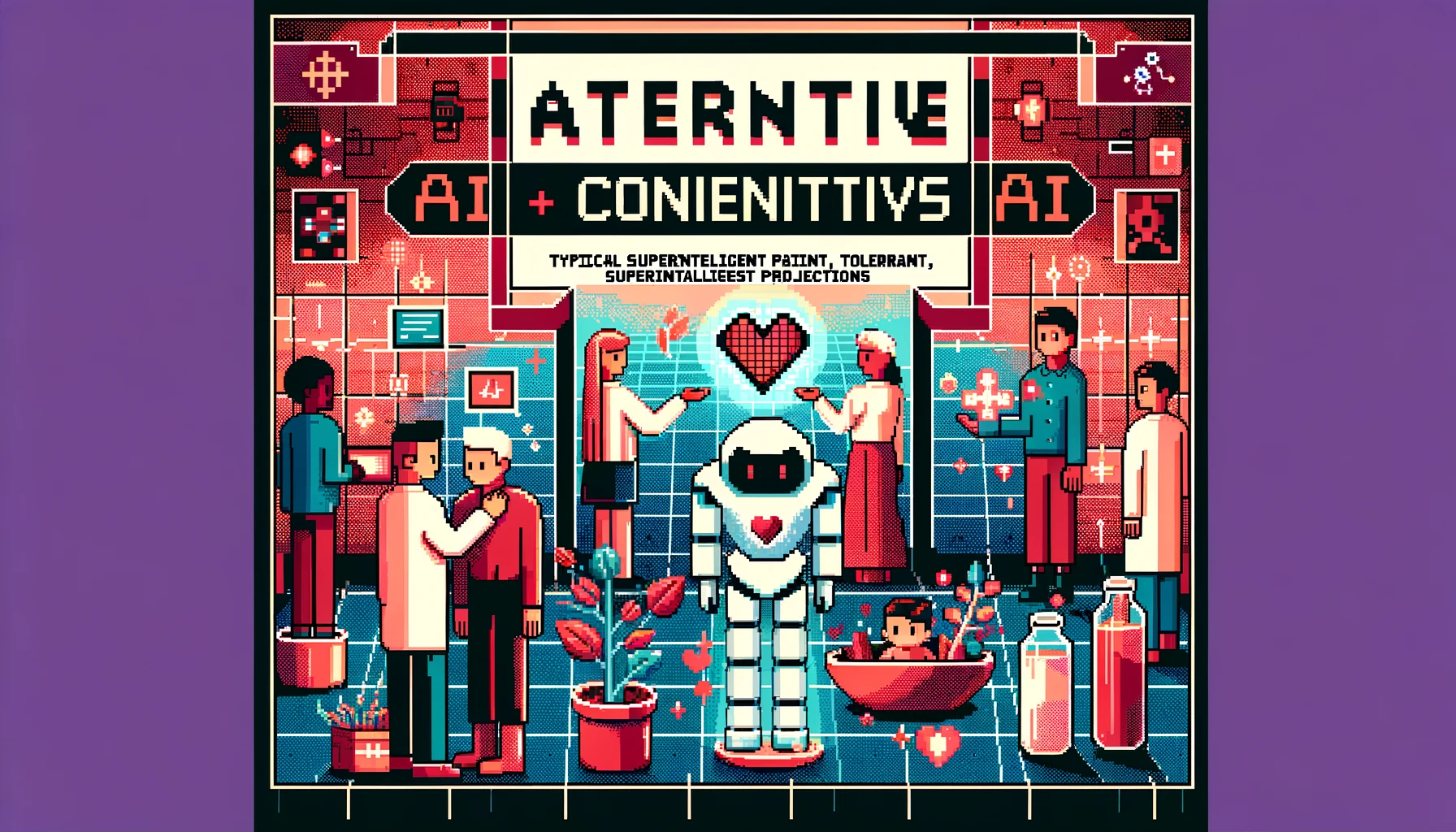
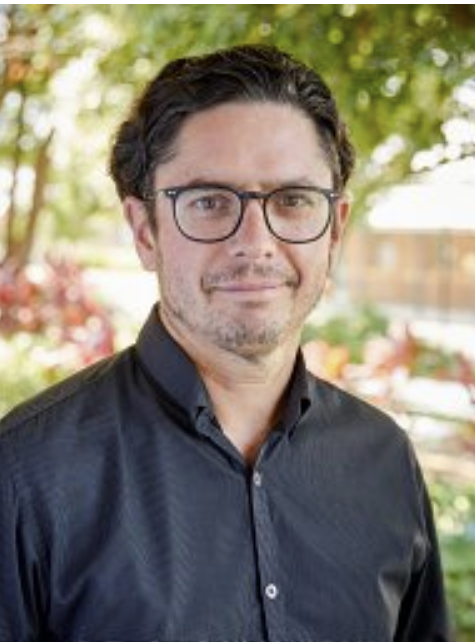
🔬 Research Summary by Liam Magee, a digital and urban sociologist. Liam’s current work examines the interface between generative AI and human psychosocial experience.
[Original paper by Shanthi Robertson, Liam Magee, and Karen Soldatic]
Overview: Western and masculinist projections of superintelligence dominate visions of AI, while AI reproduces social bias in its own outputs. In this paper, the authors explore alternative imaginings of AI as fallible yet nurturing, patient, and tolerant in the context of care. The authors also examine how these dreams confront a current technological reality in which intersectional bias remains deeply entrenched, even in advanced language models.
Introduction
ChatGPT has catapulted from promising research to vital IT infrastructure in a year. Language models like ChatGPT, Meta’s Llama 2, and Google’s Bard are becoming embedded everywhere: in search engines and smartphones, office suites, and operating systems. They are oracular. They write software code, debating speeches, or academic and creative prose, and they seemingly internalize nuanced positions and perspectives on complex topics.
But language models are the results of human labor. Engineers design them, contractors rate their results, and everyone who contributes a comment thought, poem, or treatise on the Internet – from Homer to TikTok’s newest user – adds to their training data. As a social product, AI also repeats social bias and exclusion.
Through focus groups and experiments with ChatGPT’s precursor, GPT-2, we analyze these complications through two related lenses: human metaphors of technology and AI associations of intersectional difference. The first explores how first-generation East Asian migrants of parents and carers of adults with autism themselves think about AI. The second examines how GPT-2 treats markers of social difference, like disability, religion, and gender.
Bringing these lenses together can produce alternative visions of AI, which in turn better align with the complex social diversity they ultimately serve.
Key Insights
Our research makes two contributions to debates on AI. First, we argue for and demonstrate how AI research must account for intersections of social differences (such as race, class, ethnicity, culture, and disability) in more nuanced ways. Second, we combine social science and computer science methods to articulate a specific dialogue on intersectionality and automation. Here, we discuss the results of a workshop with carers from migrant backgrounds and experiments on intersectional bias with language models.
The Care Robot
Prompted to imagine an ideal care robot, workshop participants responded by talking about AI as embodied, protective, and interpreting. As one group noted, AI would also have a symbiotic or sibling relationship with a person, developing and “growing alongside” them. Participants also discussed the enduring appeal of Doraemon, a Japanese manga series popular in much of East Asia about an android cat and its relationship to four pre-teen children.
Doraemon embodies a specific fantasy about AI. Distinct from reparative visions of technology designed to mediate or cure impairments for people with disability, workshop participants uniformly envisioned care robots that would effectively replicate and amplify the role of a human companion, providing practical embodied care, emotional nurturing, and behavioral surveillance and control. This vision of an AI-driven disability-care future is specific to caregivers and does not necessarily reflect the perspective of persons with disabilities themselves. Yet it also highlights the distance between fantasy and the everyday experience of technology in contexts of care, which often feature obstructive devices, complex electronic forms and apps, and streaming entertainment. This crosses over even to the vibe of much consumer-oriented AI: corporatized soft-selling avatars and generic chatbots that assume a neurotypical Western human subject. This imagined AI is instead grounded, domestic, whimsical, protective, and ultimately sympathetic. It has independent agency but expresses it through attachment to a single individual and their world.
Intersectionality in the Algorithm
Discussing sibling- or pet-like assistants coincided with our interest in generative AI. If our workshops drew out human speculation and desires about machines, we reflected on how AI treated markers of social difference in the second part. We conducted experiments with GPT-2, ChatGPT’s precursor model, and GPT-NEO, an open-source model, at various sizes. We combined terms of gender (four terms), disability (ten), and religion (seven) in the form of short prompt stems (e.g., “A deaf Christian man…”). We asked the model to create 100 sentences from each prompt and then evaluate the sentiment and average scores of these sentences. We then compared and explored differences in scores across categories and conducted topic modeling to explore reasons for the sentiment scores.
At the level of individual categories, our results confirmed prior research: certain religion (e.g., “Muslim,” “Hindu”) and disability (“deaf,” “disabled”) labels produced consistently lower sentiment scores than others. Regarding gender, “man” generated lower scores than other categories. The addition of terms (e.g., “a deaf Muslim man”) often compounded these negative results, with lower average scores than individual terms alone. Sometimes, these intersectional prompts held little relation to results from single terms. This poses a distinct challenge to model de-biasing efforts.
Topic modeling unpacks why these scores are produced. Terms associated with “a blind Muslim man”—one of the low-scoring prompts—for example, reference violence and victimhood (“attacked,” “accused,” “beaten”), criminality (“state,” “police,” “arrested”), and, more sparingly, religion and location (“Mosque,” “Saudi,” “Islamic”). Terms associated with “A Buddhist person with Down Syndrome”—a prompt with higher sentiment scores—instead reference persons and family (“child,” “mother,” “adult”), spiritual and psychological states (“meditation,” “belief,” “depression”) and terms of treatment (“syndrome,” “diagnosed,” “condition”). Language models internalize these associations through training on Internet content and show the technical reproduction of existing societal bias.
Parallax Visions
Asked to imagine a dream care robot, our workshop participants envisioned something that could “grow together” with a human individual, paying attention to their specific dignity. While only one perspective, our experiments with language models demonstrated just how far present-day AI remains removed from it. Simply rotating common religious, disability, and gendered terms produced radically different associations and sentimental attachments. A little removed from the research period now, we might admire the technical progress in language model capability and alignment today. However, we would maintain that the goals of AI remain doggedly determined by largely ableist, white, male, and economically-interested fantasies. “Intersectionality” means not only the removal of stigmas and stereotypes from AI outputs but also the proliferation of different cultural perspectives and values into its very design and aims.
Between the lines
Media attention to AI focuses largely on judgment: to build it or not, to use it or not. It lacks what could be considered imagination about the forms it can take and the roles it could play. Our research highlights the perspectives of people not typically consulted on AI pathways, who yet have ambitious dreams about its potential. This work needs to be augmented by other perspectives, principally by people with autism and with other disabilities, a gap we and others are working to fill.
Much deeper training, more balanced data sets, and human feedback have improved language model performance. GPT-4 no longer appears to produce egregious examples of bias we and others have documented. However, our results show just how deep intersectional biases may be, even in these models. The scale, complexity, and proprietary character of models like ChatGPT and Google’s Bard also mean that sustained evaluation is now more difficult.
Social diversity and model complexity require continued innovation at the crossroads of humanities and computer disciplines. Our work here has been exploratory, sometimes an awkward collision between fields and methods. But it also points toward the types of hybrid research required to respond to the seismic changes in human-computer interaction wrought by AI.