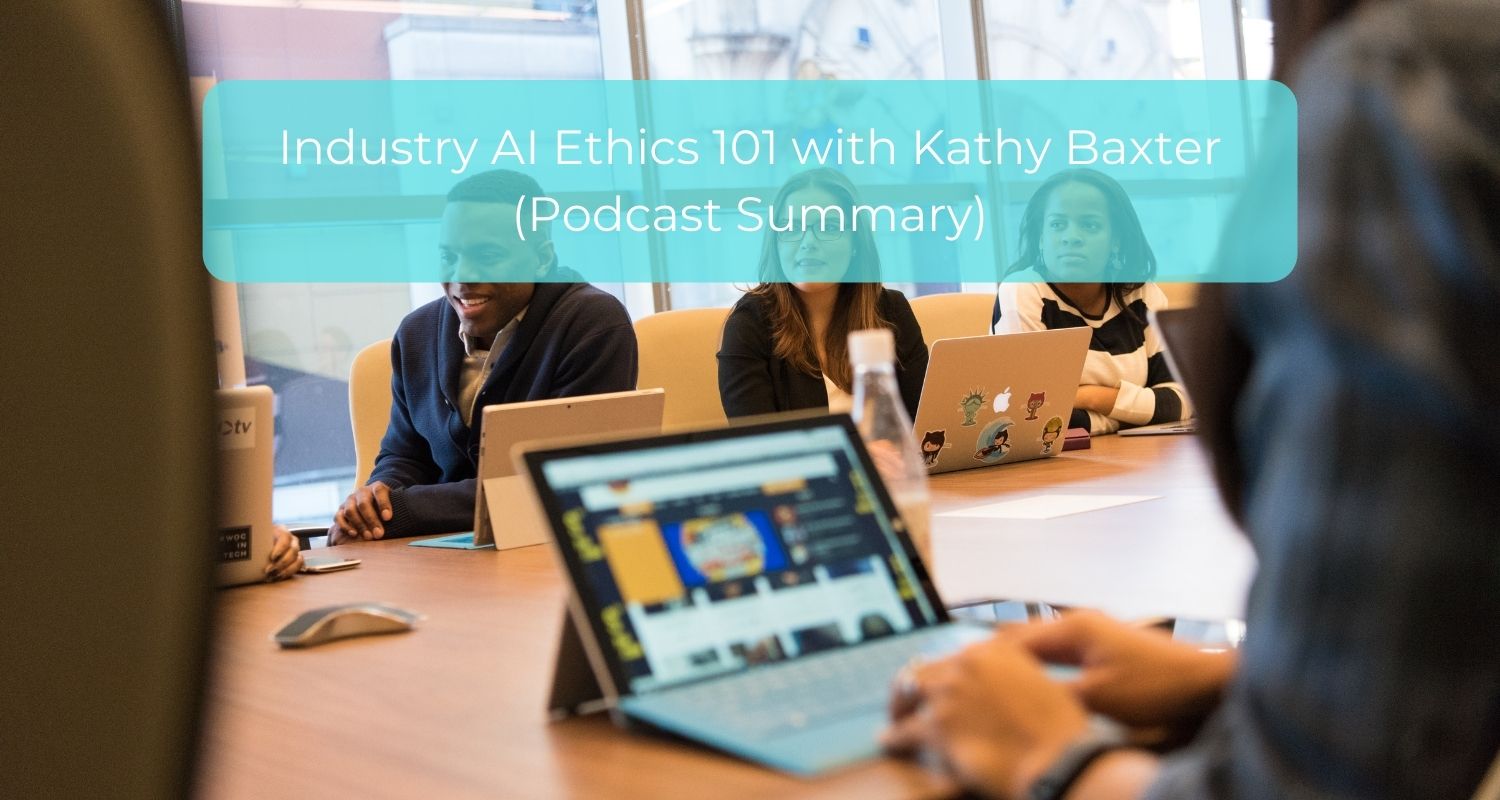
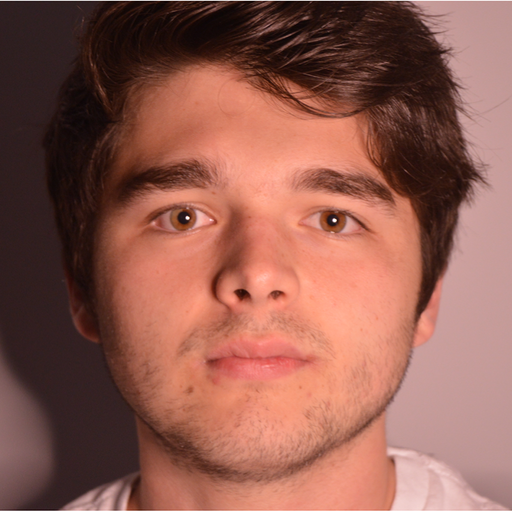
Summary contributed by our researcher Connor Wright (Philosophy, University of Exeter)
*Link to podcast at the bottom.
Overview: The design of ethical AI is no longer solely concerned with the technical, but is now intertwined with the ethical. Kathy Baxter (Principal Architect of Ethical AI Practice at Salesforce) on the Radical AI podcast takes us through how we should now navigate this relationship, and reveals her experience of how to best do this in the business environment.
In this conversation with Kathy Baxter, the Radical AI Podcast aims to get into what designing ethical AI actually entails. Conversing with Baxter, who is an Architect of AI Ethical Practice at Salesforce, the conversation meanders through Baxterâs experience with AI, touching on 3 main areas which I will mention in my piece today. Those are, firstly, what is to be taken into account when designing ethical AI, secondly, how to best land these suggestions in a corporate environment and thirdly, what Kathyâs current âdiscomfortâ is and whyâs that the case, before concluding with my final thoughts. Letâs start with designing an ethical AI system.
Nowadays, AI design no longer takes place in an ethical vacuum, with the environments of both the ethical and the technological being intertwined. Hence, ethical considerations are now having to be introduced into the formerly purely technical design of AI systems. In this way, Baxter advocates for the consideration of who is impacted being by the system being key in not only designing an ethical AI system, but also a more equitable society. In order to do this, considerations on what factors the AI is using to come to a decision need to be taken into account. For example, an AI system using the quantity of steps taken daily by the average American to assess the fitness of the nation, which then would exclude those in a wheelchair. In this way, questions about how the factors are being applied (such as how they are measured), whom is it being applied to (such as solely those with enough income to afford a step-monitor) and whether such application is equal as a result, need to be asked in the design process.
Nonetheless, it needs to be acknowledged, as Baxter does, that there will always be some form of bias present in the design process. So, Baxter elaborates on three different aspects within the design in order to establish guardrails against the potential harms created from the presence of bias. The first, is to locate where the responsibility lies. Often, those designing the AI system are not those implementing it. Hence, the second guardrail is to identify whoâs implementing the technology, helping to identify the audience who are to benefit from knowledge about what to look out for in order to identify bias. A third and final guardrail is to start conversations about the role of society and policymakers in the AI design process, with more and more communities now coming forward and drawing their own âred linesâ. In this way, these guardrails will contribute to understanding the negative impact of what the AI product being implemented is having over certain parts of the population.
Accompanying this, sustains Baxter, is the crucial notion of a change of mindset in the industry, which I believe can be summed up by the â90% fallacyâ. Here, the fallacy centres around how anything being above 90% accurate is falsely assumed to be completely accurate. In this way, rather than the industry being content with implementing an AI system that is 98% accurate and figuring out the details later, the industry should rather decide to tackle the 2% inaccuracy itself. To illustrate this, Baxter mentions how if this is not to be done, the same 2% of the population (affected by this accuracy) will be repeatedly marginalised over and over again. In this sense, by leaving this 2% outside of the realm of consideration, companies develop what Baxter terms âethical debtâ. Here, companies will eventually have to ârepayâ the ethical debt being accumulated through not tackling the release of such systems, which will manifest itself in the suffering of those being marginalised by the system.
To prevent this, a change of incentive structure will need to take place. Rather than centring the success of workers towards click-through stats and revenue, the incentive should be to minimise the potential social harm being caused by the AI system. In this way, AI is to rather be seen to empower clientele, rather than to exacerbate the current divide. In order to best land this radical and generally unwanted change, Baxter emphasises the role of context.
In her exposure to the business environment, Baxter initially started out by explaining the dangers of AI at Salesforce with the most shocking examples (such as facial recognition and predictive policing). She was met will accolades along the lines of âThat was a fantastic talk!â, however, she was not met with the impact she wanted. Here, workers would not follow up with her on her talk and how to implement it within the business as they had nothing to do with the dangers that Baxter had mentioned. In this way, Baxter realised the value of using examples that actually pertained to the current business context. To quote Baxter themselves, âPeople will be on board, but you have to tell them how to come on boardâ. People do care about the impacts of AI in the business environment, but implementing the changes that get them fully on board requires the use of context to show them how to make their own impact on the debate.
Baxter was also able to mention how the implementation of such changes also requires a whole village, rather than solely an âethics boardâ. All different parts of the design process are to be influenced by the changes required to tackle bias in AI, so effectively communicating such changes and cultivating the enthusiasm to do so requires the efforts of all involved. Effectively communicating is an especially important aspect, given how as Baxter acknowledges, ethics touches on peopleâs values. Hence, going about creating the village required to implement the desired changes in the AI process requires a calm and understanding approach in order not to provoke any visceral responses.
Baxter was then asked about the importance of discomfort, and what sheâs currently uncomfortable about within the AI space. As her answer, Baxter presented the disinformation debate as being comparable to the game of whack-a-mole. Given the multitude of ways to spread disinformation on social media, content moderators are having to sift through thousands and thousands of publications daily in order to appropriately filter out fake news, whacking the mole as soon as they come up. However, being exposed to some of the more popular conspiracy theories has meant that some of the content moderators start to be persuaded by these conspiracy theories. In this way, the whack-a-mole game becomes ever-more difficult in order to avoid personal persuasions when tackling disinformation. As a potential way to combat this, Baxter talked about trying to create a common base of agreed-upon facts about the world we live in, but she admitted how she doesnât quite know how to go about achieving this. Hence, her main discomfort resides in trying to tackle disinformation, with the whack-a-mole method becoming ever harder to keep up.
This podcast episode provides some much-needed insight into how AI pans out in the business environment. With the need to give much more thought to the AI design process about the systemâs impact, Baxter illustrated practical and justified reasoning as to how to meet this challenge. Her lessons taken from her business experiences serve to highlight the need for a collectivist rather than individualist approach to designing ethical AI, especially when trying to tackle Baxterâs particular discomfort around disinformation. Baxter will play a pivotal role in influencing the AI design process in the future, and deservedly so.
Episode of the Radical AI Podcast with guest Kathy Baxter: https://www.radicalai.org/industry-ai-ethics