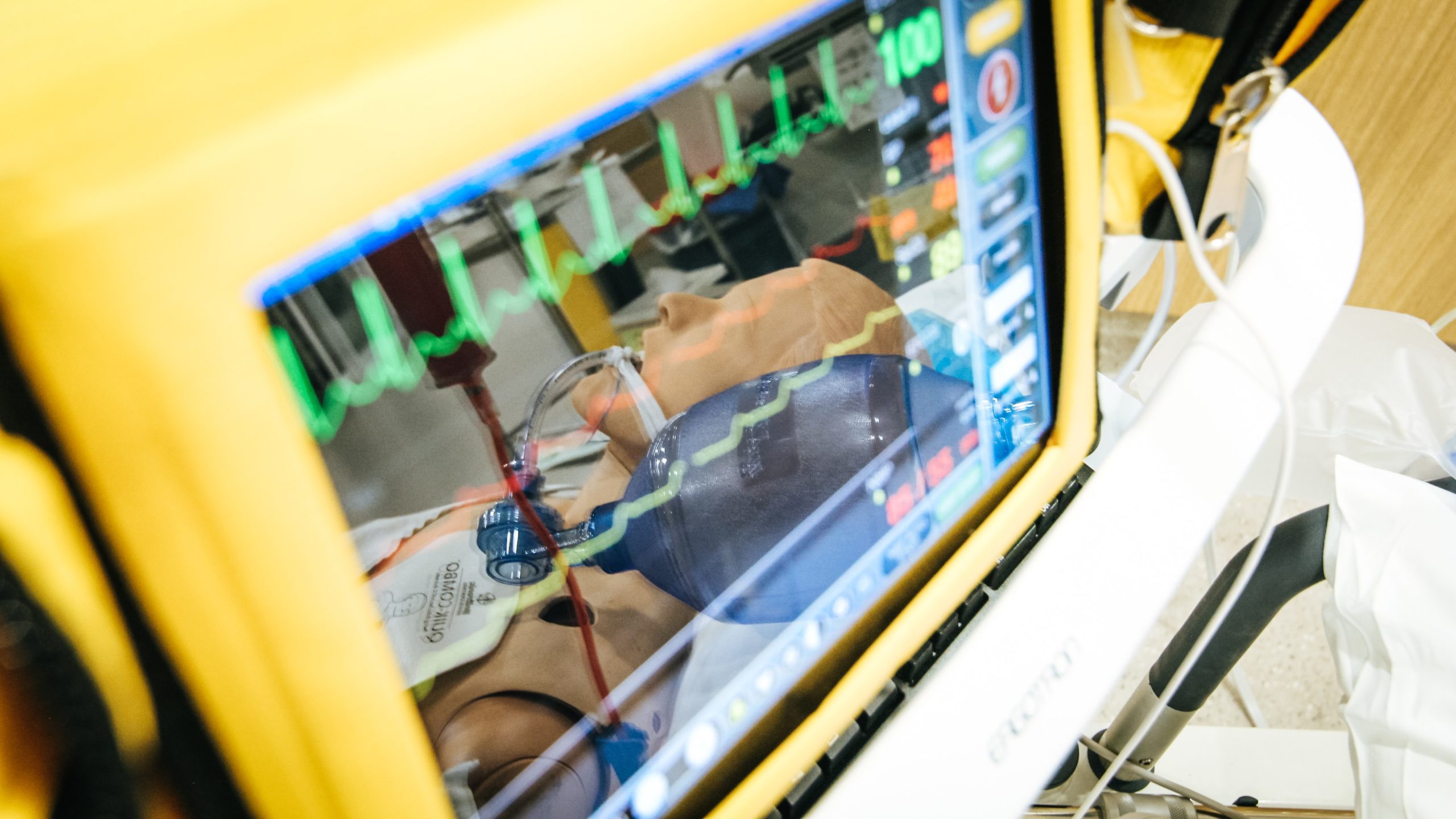
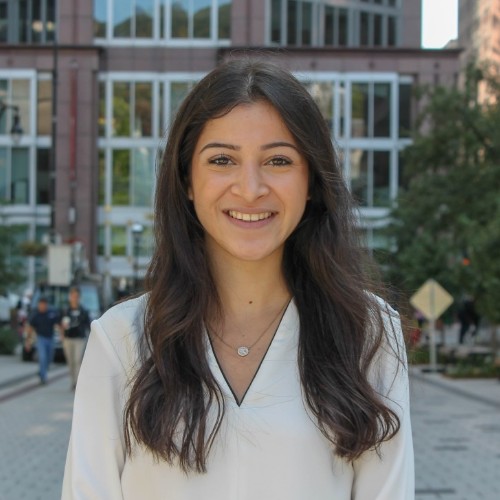
✍️ Column by Masa Sweidan, our Business Development Manager. This is her introductory piece to The New Heartbeat of Healthcare series.
Health has been top of mind for many of us, especially during the last 2 years. It is no surprise that fears of catching COVID-19 during in-person medical visits have led to a greater interest in using technology to receive health care, with almost three-quarters of Americans stating that the pandemic has made them more eager to try virtual care. Increased consumer and provider willingness to use technology has meant that the use of telehealth has grown 38x from the pre-COVID baseline, with around 3X the level of venture capital investment in the area of digital health in 2020 compared to 2017.
Before exploring the role of AI in healthcare, it is important to point out the difference between the terms ‘telehealth’ and ‘telemedicine.’ Consider telehealth to be the larger umbrella term encompassing the broad scope of “electronic and telecommunications technologies and services used to provide care and services at-a-distance.” Telemedicine is a subset of telehealth that specifically refers to the practice of medicine using technology to deliver care at a distance. Essentially, the main difference lies in the fact that telemedicine solely pertains to remote clinical services, while telehealth can also include remote non-clinical services, such as provider training, patient education and administrative meetings.
For some time, telehealth simply referred to videoconferencing between a doctor and their patient. Now, the new wave of technology is being developed much quicker than expected due to the COVID-19 pandemic, and it has AI and IoT embedded in its DNA. Applying AI to telehealth has been empowering doctors to make real-time, data-driven choices, generating a better patient experience. Several creative solutions that have made a positive impact include AI providing prompts to take a patient’s history during a virtual visit, algorithms helping with diagnostics and AI-based tools offering personalized reminders for medication. With these exciting developments, the future of healthcare promises more accurate diagnoses, reduced burnout rates amongst doctors and simplified hospital visits.
More specifically, a compelling use case of AI in telehealth is remote patient monitoring (RPM). This is a healthcare delivery method that uses technology to monitor patient health outside of a traditional clinical setting. RPM targets several sub-group of patients, including those living with chronic illnesses and mobility issues, in addition to post-surgery patients, neonates and elderly patients. These individuals typically have conditions that would benefit from being monitored continuously. With RPM, technology is used to electronically collect and transmit information between patients and physicians. Wearable technologies, such as smartwatches and various wearable monitors, are equipped with heart rate, stress, and blood oxygen detectors, enabling healthcare professionals to accurately monitor vital signs in real-time.
The power of these technologies lies in the fact that patient generated health data (PGHD) can be continually collected to establish an accurate baseline measurement. This can help to avoid, for example, the “white coat effect,” which occurs when one’s blood pressure readings at their doctor’s office are higher than they are in other settings, such as their home. This phenomenon could occur simply due to the stress that often accompanies a visit to the doctor, but it could also signal one’s risk of developing high blood pressure as a long-term condition, affecting the risk of having a stroke or heart attack. With wearables providing a more complete picture, patients and healthcare professionals can better understand when measurements drastically vary from the norm that has been established at home.
However, from a statistical standpoint, PGHD can be noisy. Some data may be meaningless or difficult to interpret. To mitigate this, AI can be used for automated anomaly detection. With unsupervised machine learning, the model can discover patterns on its own from unlabeled datasets by grouping similar data together into clusters. In this context, the analysis would be used to build a patient’s profile based on grouped data, detect anomalies in real-time and remove invalid data. Due to the ability of a machine learning (ML) model to flag measurements that deviate from the mean, AI can help us better understand the PGHD.
Such insights prove to be particularly useful when determining if a patient is at a high risk of readmission. To begin with, RPM has the ability to minimize readmissions by 38%. With the incorporation of ML, predictions of readmission risk can be made by using both static and longitudinal variables in order to provide a more comprehensive analysis of the patient’s state and help clinicians use their time more effectively by directing their attention towards those who need it. It is clear that implementing ML techniques into RPM devices can promote preventative care and ultimately provide clinical decision support to healthcare professionals.
As is the case with the infusion of AI into any industry, there are often challenges hindering wide-scale adoption. How will regulators evaluate medical machine learning (MML)-based devices to ensure their safety and effectiveness? One of the main hurdles has been the fact that the regulatory frameworks are lagging behind as there are currently no harmonized laws that specifically regulate the use of MML-enabled devices, leading to uncertain risks for RPM device manufacturers. Topics including data privacy, training set bias and equitable access are vital to the development of robust regulatory frameworks that apply to RPM.
Nevertheless, it is promising to see that steps are being taken in the right direction. In January 2021, the FDA released their Artificial Intelligence/Machine Learning (AI/ML)-Based Software as a Medical Device (SaMD) Action Plan, which discussed their targets to incorporate Good Machine Learning Practice (GMLP), implement a patient-centered approach incorporating transparency and address algorithmic bias. These considerations are crucial, as the incorporation of ML means that RPM devices will not simply be used to collect data. As more innovative telehealth solutions continue to appear on the market, the next generation of RPM devices must overcome these regulatory challenges in order to reach their full potential of improving data-driven clinical decision making and enhancing the overall patient experience.