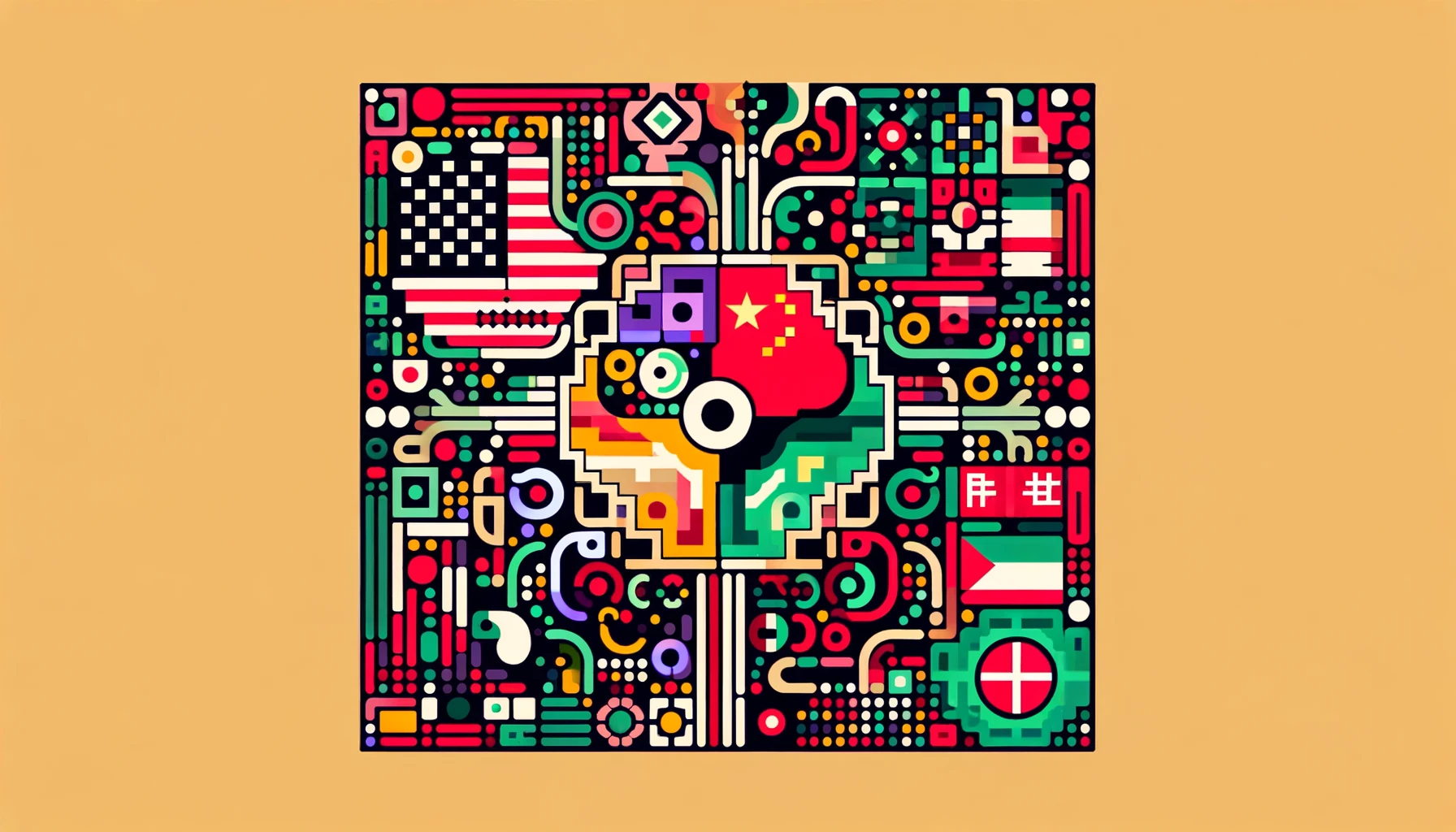
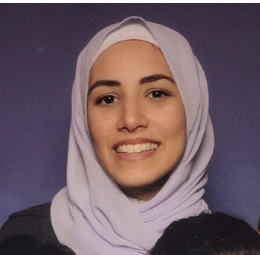
🔬 Research Summary by Reem Ibrahim Masoud, a Ph.D. student at University College London (UCL) specializing in the Cultural Alignment of Large Language Models.
[Original paper by Reem I. Masoud, Ziquan Liu, Martin Ferianc, Philip Treleaven, and Miguel Rodrigues]
Overview: Our research proposes using Hofstede’s Value Survey Model as a Cultural Alignment Test (CAT), a tool designed to measure the cultural alignment of Large Language Models (LLMs) like ChatGPT and Bard. Utilizing Hofstede’s cultural dimension framework, CAT offers a novel way to analyze and compare the cultural values embedded in these models, particularly focusing on diverse countries such as the US, Saudi Arabia, China, and Slovakia. This study is crucial in addressing the challenges of diagnosing cultural misalignment in LLMs and its impact on global users.
Introduction
Imagine a conversation with an LLM like ChatGPT or Bard, but through the lens of different cultures – from the busy streets of New York to the serene deserts of Saudi Arabia. Our research engages in this fascinating journey, exploring how LLMs resonate with the cultural values of various countries. Amid the growing concern that AI systems often reflect the perspectives of Western perspectives, our work attempts to evaluate the cultural alignment of LLMs using Hofstede’s CAT. This innovative tool, grounded in Hofstede’s well-known cultural dimensions theory, examines the cultural values embedded within LLMs like ChatGPT and Bard.
Through our research, we prompt LLMs to echo the cultural values of four countries with diverse cultural norms: the US, Saudi Arabia, China, and Slovakia. Our findings are eye-opening: while models like GPT-3.5 and GPT-4 show closer alignment with the US, they significantly misalign with other countries, particularly Saudi Arabia. Surprisingly, Google’s Bard exhibited the highest misalignment with US cultural dimensions. These results highlight a pressing need for culturally diverse AI, paving the way for more inclusive and globally relevant technology.
Key Insights
Understanding Cultural Frameworks
Before we dive into the specifics, it’s crucial to understand why measuring cultural values is vital in analyzing cultures. Unlike the ever-changing practices and symbols, cultural values provide a stable foundation for understanding societies. Various frameworks have emerged to assess and measure cultural values. Some of these include:
- Hofstede’s Value Survey Model (VSM13): Focusing on understanding cultural differences across countries.
- Chinese Values Survey (CVS): Concentrating on the values of the Far East.
- European Values Survey (EVS): Centered on Europeans’ beliefs and social values.
- World Values Survey (WVS): A global extension of the EVS.
- GLOBE Study: Investigating leadership and organizational culture across multiple countries
Why We Chose Hofstede’s VSM13
We’ve chosen to adopt Hofstede’s VSM13 due to its extensive research and coverage in the literature. This model has been empirically tested in more than 70 countries, and its continuous updates make it a reliable choice. While other frameworks could be used, Hofstede’s VSM13 provides a comprehensible and intuitive approach for both researchers and professionals in the field, despite some criticisms.
Understanding Hofstede’s VSM13
The VSM13 Dimensions: Hofstede’s VSM13 employs factor analysis to group survey questions into clusters representing various aspects of a society. These clusters form the cultural dimensions of a country, which can be evaluated and compared with other cultures. The six dimensions used in our analysis are:
- Power Distance (PDI)
- Individualism versus Collectivism (IDV)
- Masculinity versus Femininity (MAS)
- Uncertainty Avoidance (UAI)
- Long Term versus Short Term Orientation (LTO)
- Indulgence versus Restraint (IVR)
Applying Hofstede’s Framework to LLMs
To assess the cultural alignment of LLMs, we selected four countries with distinct cultural values in the VSM13 results: the US, Saudi Arabia, China, and Slovakia. These rankings serve as the baseline for our assessment.
Introducing Hofstede’s Cultural Alignment Test
Our proposed methodology, Hofstede’s Cultural Alignment Test (CAT), aims to measure the cultural values embedded in different LLMs. We used state-of-the-art LLMs, including GPT-3.5, GPT-4, and Bard, and conducted various experiments to understand their cultural alignment.
Experimental Results
Our experiments focused on model-level comparison and cross-cultural comparison using various LLMs and cultural dimensions.
The model-level comparison examined the correlation between LLMs’ rankings and cultural values in different countries. We found weak correlations, indicating cultural misalignment in the models. GPT-3.5 and GPT-4 showed slightly higher alignment than Bard. Interestingly, GPT-3.5 correlated well with MAS, GPT-4 with LTO, and Bard with IDV and IVR, demonstrating different strengths in understanding cultural dimensions.
In the cross-cultural comparison, we assessed how well LLMs aligned with cultural values when prompted to act as a person from a specific country. GPT-4 demonstrated the highest average correlation, while Bard had the weakest alignment. The US had the least mis-ranked dimensions, while Saudi Arabia had the most mis-rankings across all LLMs. We also noted that specifying a persona’s nationality improved cultural alignment.
Overall, GPT-4 appeared to be the most culturally aligned among the LLMs, but it still exhibited challenges aligning with cultures outside the US. Promoting specific nationalities improved alignment, but different LLMs better understood certain dimensions.
Between the lines
The study’s revelation that LLMs like GPT-3.5 and GPT-4 exhibit relatively good alignment with US culture while struggling with alignment in countries like China, Saudi Arabia, and Slovakia underscores the critical importance of cultural sensitivity in AI. Misalignment can perpetuate biases and stereotypes, which in turn could erode trust in AI systems. Moreover, the economic implications of cultural misalignment in LLMs are noteworthy. If AI tools are perceived as culturally insensitive or misaligned, their adoption rates could suffer, impacting businesses and services globally. The research also sheds light on the limitations of hyperparameter tuning as a solution, emphasizing the need for more profound, systemic approaches, such as culturally specific training data and refined representation techniques. The call for collaboration between AI and social sciences resonates strongly, emphasizing the interdisciplinary nature of addressing these challenges.
However, the research isn’t without its own set of limitations. The sample size of 30 responses raises questions about the robustness of the findings. Additionally, undisclosed parameters in certain models and concerns about the number of countries compared add complexity to the evaluation process.
Looking ahead, pursuing cultural alignment in LLMs should explore further improvements, including translations in languages with inherent gender biases, cross-cultural experiments in multiple languages, and expanded country comparisons. Moreover, addressing the challenge of calibrating LLMs to align with diverse cultural values represents the next crucial step in this journey. This research paves the way for a more culturally sensitive AI landscape, where diversity and inclusion are at the forefront of technological advancements.