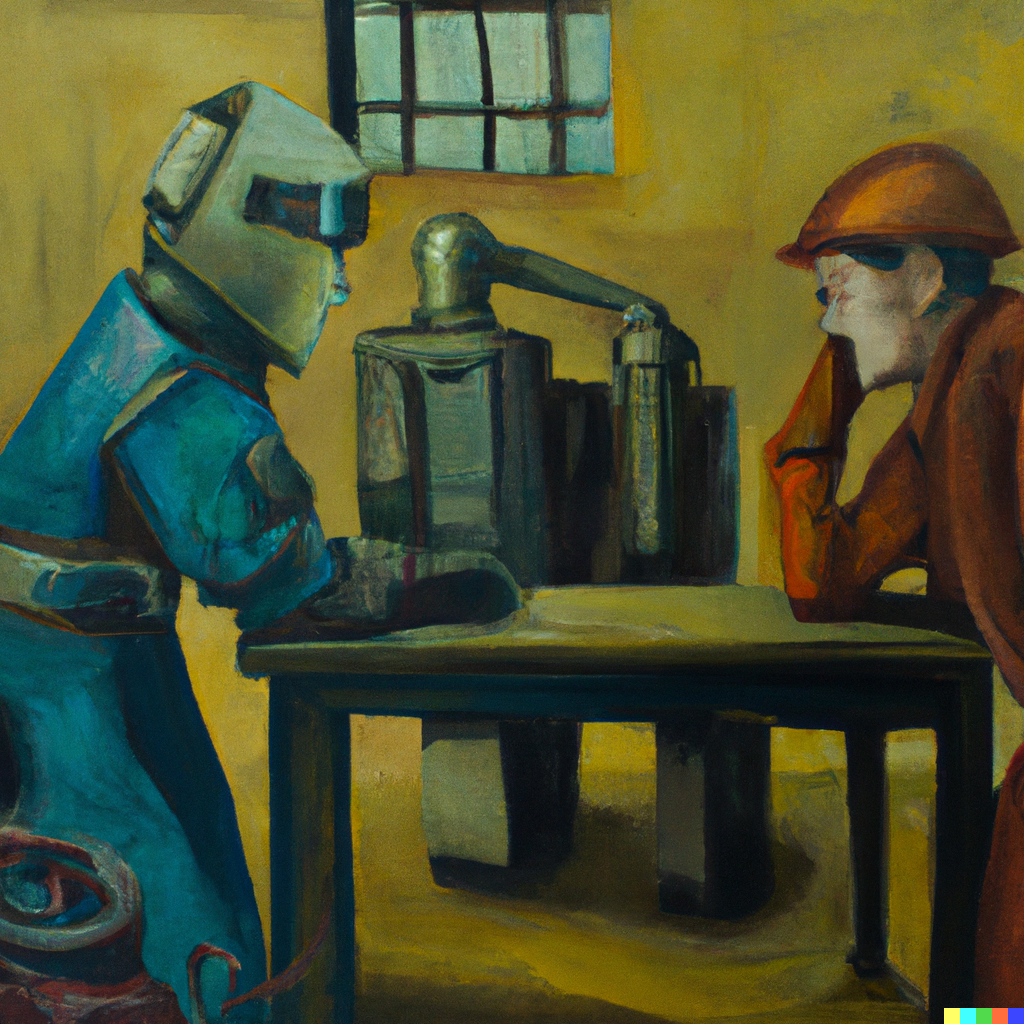
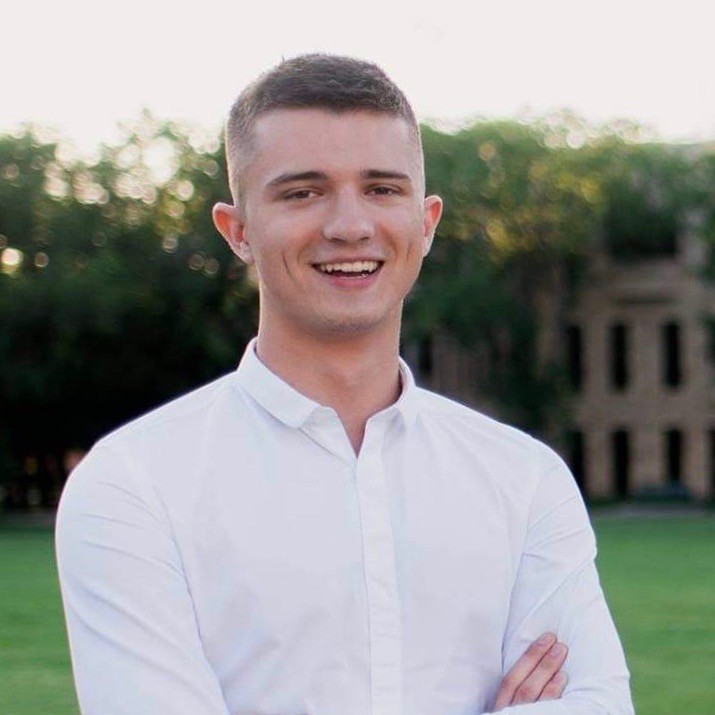
🔬 Research Summary by Joshua Brand, a PhD student at Télécom Paris-Institut Polytechnique de Paris with his thesis on Human-centred explainable artificial intelligence and the virtue of vulnerability.
[Original paper by Astrid Bertrand, Rafik Belloum, James R. Eagan, and Winston Maxwell]
Overview: The use of Explainable AI (XAI) technology is developing rapidly alongside claims that it will improve AI’s ethical and trustworthy characteristics in decision-making processes. This paper, however, reviews how human cognitive biases can affect, and be affected by, explainability systems. After a systematic review of the relationship between cognitive biases and XAI, the authors provide a roadmap for future XAI development that acknowledges and embraces human cognitive processes.
Introduction
Explainable AI (XAI) is a set of tools that helps users of AI systems understand and interpret algorithmic predictions used in decision-making processes. This is to primarily overcome the ‘black box’ issue of complex algorithmic systems whose predictions evade human comprehension. For example, in many high-stake situations, such as medical decisions or loan approvals, explanations for AI-assisted decisions are desirable—we ought to know that decisions are based on fair and non-discriminatory reasons. XAI, therefore, helps users and stakeholders justify AI-assisted decision-making procedures.
This paper, however, reminds us that the relationship between users and XAI is not always smooth sailing. The authors illustrate how explanations can encourage cognitive biases through a systematic review of XAI literature. More specifically, they provide a heuristic map informed by 37 papers that synthesizes four ways in which human cognitive biases are affected by and affect XAI technology. By bringing attention to this relationship, the authors subsequently advance a research agenda for future work on XAI and cognitive biases that focuses on the desire to fit within the cognitive realities of users and stakeholders—thus directing attention to human-centered XAI.
Key Insights
Cognitive Bias and XAI
Methodology
To investigate the comprehensive relationship between cognitive bias and XAI, the authors posed five key research questions:
- what cognitive biases have been studied in XAI literature
- in which specific methods of XAI do these biases arise
- are there ways to mitigate negative biases while supporting appropriate ones to improve XAI systems
- what methods were used to detect cognitive biases
- what are the identified future directions and challenges
Using these questions, the authors whittled down a corpus of 285 papers to a systematic review of 37 published since 2008. From this, they identified 53 cognitive biases, such as automation bias, over-generalization, and homunculus bias, that occur throughout XAI systems.
The authors arrived at this comprehensive list by identifying, screening, and analyzing relevant papers following the reporting checklist standard PRISMA: Preferred Reporting Items Systematic Reviews and Meta-Analyses. This involved keyword matching in various databases and identifying cognitive biases through external taxonomies, surveys, and general knowledge, among other techniques, when not explicitly stated in the research.
In addition to revealing an extensive list of present cognitive biases, this work also revealed that most research is found within the Human-Computer Interaction (HCI) field, which aims to focus on the user as much as the technology.
Four Relationships
Having provided an extensive summary of biases present in XAI, the authors maintain that there are four key relationships between cognitive bias and XAI. The first two illustrate cognitive biases’ effects on XAI development and evaluation, while the other two illustrate how using XAI affects our cognitive biases, both positively and negatively.
- Cognitive biases affect how XAI tools are designed. For example, humans tend to require communication and interaction during explanations, as explainability is considered a social process as much as a cognitive procedure. Therefore, interactive XAI tools are preferred. This is neither negative nor positive but is presented as a reasonable constraint on XAI development.
- Cognitive biases can also distort the evaluation of XAI tools through user studies. Subjective preferences, background knowledge, and change blindness (the lack of ability to recognize a change in visual mediums) affect how users evaluate and justify the use of a system, leading to unreliable and misleading assessments of XAI efficacy.
- XAI can exacerbate cognitive biases. This is mainly attributed to, through the research of Buçinca et al. (2021), the strain between trusting AI and choosing to engage in a demanding analysis of its explanations and the lack of casual certainty. Users respond with cognitive biases, such as misapplication, over-reliance, and under-reliance on explanations.
- XAI can also mitigate cognitive biases. Primarily, XAI has proven to mitigate pre-use algorithmic optimism; essentially, explanations help tame disproportionate expectations of algorithms by serving as a reminder not to overestimate the system’s capabilities.
Importantly, the authors decided that, in many cases, it is better to define cognitive bias as explanatory heuristics to avoid the notion that biases are necessarily negative factors that lead to an error of judgment. They can also be understood as the intrinsic way humans explain phenomena. Cognitive biases, therefore, are positive, negative, and value-neutral factors in the context of XAI.
The Way Forward
Taking the systematic review further, the authors respond to the current literature with an agenda of seven proposals to improve cognitive bias-XAI research.
- Clarify which cognitive biases are negative, positive, or value-neutral to inform the design of explanation systems on how to protect us from or simply account for them. Further work is thus also needed on the benefits and disadvantages of incorporating them into XAI design. For example, Weld and Bansal (2019) proposed, “Should an explanation system exploit human limitations or seek to protect us from them?”.
- Theoretical work on how we should assess XAI and its decision-making process. This will help better understand a hierarchy of which biases must be dealt with the most.
- Identify all relevant stakeholders of XAI systems to understand better which biases may be present and need to be addressed.
- Understand users’ social and emotional behavior when using XAI to be more equipped to help them understand how the model works (through their mental modes) and correct errors caused by cognitive bias.
- Supplement quantitative user evaluations with human-grounded benchmarks, without humans, also known as human attention masks (proposed by Mohseni et al. 2021).
- Address other ways users interact with XAI—explanations are not the only relevant factor with cognitive biases: contextual information, timing, and compelling a user to reconsider their response to an explanation can all encourage cognitive biases.
- Further research is needed on ensuring user autonomy and accountability; current research on providing adversarial explanations is a promising way to help users detect when an AI system is wrong, thus giving them more ownership in their decision to accept or deny the proposed AI decision.
Between the lines
While the authors acknowledge some limitations to their review, such as that their comprehensive list of cognitive biases present in XAI is not finite, they nevertheless provide a detailed outlook on the key issues facing the appeal of XAI. As the argument for XAI implementation largely focuses on the needs of the users and stakeholders—that it is built for them as they deserve explanations—the users’ flaws can get overlooked. While cognitive biases are not necessarily negative flaws, they nevertheless affect the adequacy of XAI and its purpose to provide useful explanations. By filling the gap in XAI literature with this comprehensive understanding of how XAI and cognitive biases interact, the authors additionally give normative insight into future XAI development. In other words, if future XAI development is to appreciate the limits of both the technology and users, it must aim for a comprehensive understanding of human-computer interaction.