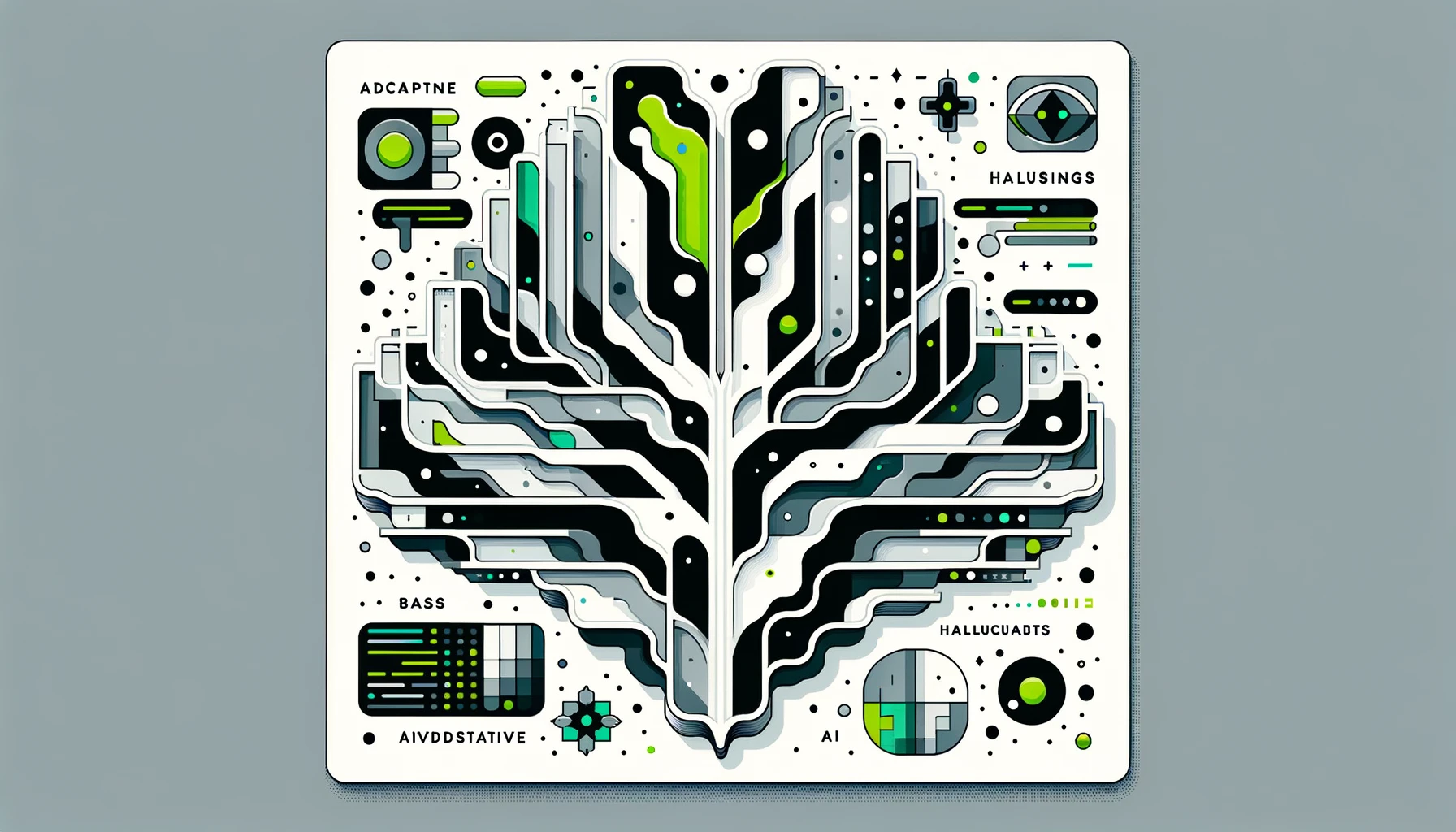
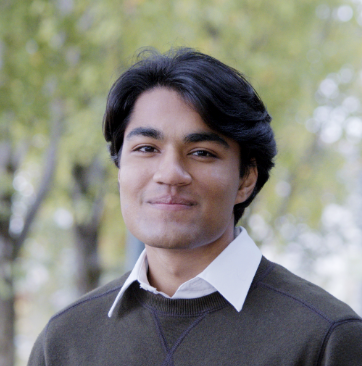
🔬 Research Summary by Rishab Jain, a neuroscience & AI researcher at Massachusetts General Hospital and a student at Harvard College.
[Original paper by Rishab Jain and Aditya Jain]
Overview: The widespread adoption of artificial intelligence (AI) in various research fields is driven by specialized AI models designed for specific tasks. Still, their biases are influenced by limited data and parameters. The use of large language models (LLMs) and generative AI tools like ChatGPT in research is less clear; these models, trained on vast datasets and human feedback, face challenges in bias identification, goal misgeneralization, hallucinations, and vulnerability to adversarial attacks. Incorporating these tools in writing research manuscripts introduces context-induced algorithmic biases and other unintended negative consequences for academia and knowledge dissemination.
Introduction
Have you ever found LLM-based tools catering their answers to you based on the information you provide them? What about catering to demographic information you inadvertently leave in your prompt?
Our research paper “Generative AI in Writing Research Papers: A New Type of Algorithmic Bias and Uncertainty in Scholarly Work” delves into the consequences of employing large language models (LLMs) like ChatGPT in academic writing. We scrutinize the biases introduced by these generative AI tools, which stem from their training on vast, diverse datasets combined with human feedback.
Such biases are harder to detect and address, potentially leading to issues like goal misgeneralization, hallucinations, and susceptibility to adversarial attacks. This study conducts a systematic review to quantify the influence of generative AI in academic authorship and highlights the emerging types of biases, particularly context-induced biases.
Incorporating generative AI in academic writing introduces unique challenges and biases, adversely impacting the integrity and development of scholarly work.
Key Insights
Our research paper comprehensively examines the implications of using generative AI, specifically large language models (LLMs), in academic writing.
Overview of Generative AI in Academic Writing
There is an increasing incorporation of generative AI tools, like ChatGPT, in the academic writing process. These tools, powered by sophisticated algorithms and vast datasets, offer notable benefits. They significantly boost productivity by generating ideas, drafting sections of papers, and providing quick information synthesis. Additionally, they inspire creativity by suggesting diverse perspectives and novel approaches to research problems.
However, we must underscore critical concerns. The primary issue is the inherent biases and uncertainties in these AI models. Since these models are trained on large and varied datasets, including content from the internet and user interactions, they may inadvertently learn and replicate biases present in the training data. These biases could be related to language, culture, or specific subject matter, and they skew the AI’s outputs in ways that are not immediately apparent.
Furthermore, the paper points to the uncertainty in the reliability of information provided by these AI tools. Given their programming to generate plausible content based on patterns in their training data, there’s a risk of generating content that seems accurate but may be factually incorrect or misleading. This raises concerns about the integrity and accuracy of academic work that relies heavily on AI-generated content.
Algorithmic Bias and Uncertainty
Unlike traditional biases, which are often overt and easier to identify, the biases in AI systems are more insidious. They often manifest subtly, making detection and correction challenging. We categorize these biases, emphasizing context-induced biases – a phenomenon where the AI’s output is skewed based on the specific context it has learned from its dataset. This section of our work is dedicated to unraveling the layers of these complex biases and exploring strategies to mitigate their impact.
Impact on Scholarly Work
We examine the profound impact of these biases on academic research and writing. We highlight two key issues: goal misgeneralization and hallucinations. Goal misgeneralization occurs when the AI deviates from the intended research objective, leading to outputs that may seem relevant but are actually misaligned with the research goals. Hallucinations are another critical issue, where the AI generates factually incorrect or misleading content, posing serious threats to the integrity of academic work. Our analysis here aims to bring these issues to light and encourage a more cautious approach to using AI in scholarly contexts.
Methodological Approach
We conduct a systematic review to understand the influence of generative AI on academic authorship. This review involves analyzing diverse cases and examples to illustrate how biases from AI tools can significantly affect the quality and integrity of research work. Our methodological approach is meticulous, ensuring the study comprehensively covers the various dimensions of AI-induced biases in academic writing. This part of the paper is crucial in providing empirical evidence to support the theoretical concerns raised in earlier sections.
Conclusions and Recommendations
There is a need for awareness and careful management of the use of generative AI in academic writing. While these tools have significant potential, they also pose unique challenges that must be addressed to maintain the integrity and development of scholarly work. There is further research in this area, especially in developing more transparent and accountable AI systems and guidelines for their use in academia. More empirical studies are needed to understand the real-world impact of AI-induced biases across different academic disciplines.
Between the lines
The findings of this research on generative AI’s impact on academic writing are crucial as they spotlight the subtleties and complexities of integrating AI tools in scholarly work. While these tools can enhance productivity and creativity, the embedded biases and uncertainties they introduce are significant concerns. Our work, however, leaves open questions regarding how these biases can be mitigated or managed in the academic context.
This gap prompts further exploration into developing more transparent, accountable AI systems and guidelines for their use in academic writing. Additionally, there’s a need for more empirical studies to understand the real-world impact of these biases on various academic disciplines. Our research thus opens the door to a vital, ongoing conversation about the role and limitations of AI in scholarly endeavors.