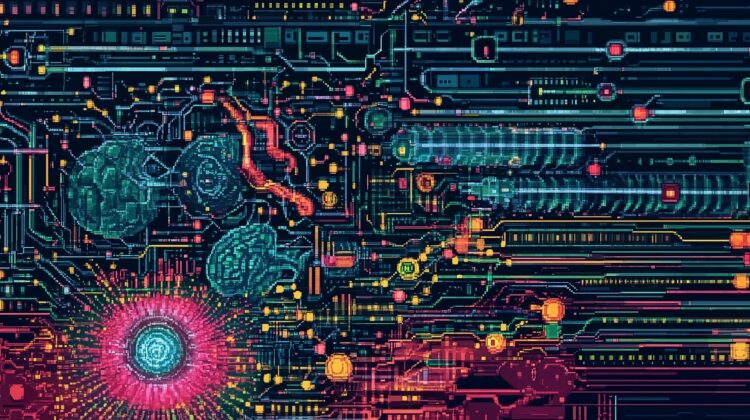
🔬 Original article by Le Thuy Duong Nguyen from Encode Canada.
📌 Editor’s Note: This article was originally written in February 2024 and is now being published as part of our Recess series, featuring university students from across Canada exploring ethical challenges in AI. Written by members of Encode Canada—a student-led advocacy organization dedicated to including Canadian youth in essential conversations about the future of AI—these pieces aim to spark discussions on AI literacy and ethics. As AI continues to evolve, the questions raised in this article remain highly relevant, and we’re excited to bring this perspective to a wider audience.
Introduction
In 1998, the editorial board of the renowned journal Nature Neuroscience highlighted the potential ramifications of neuroscience findings, suggesting that researchers in this field should be cognizant of the “profound and potentially unsettling implications of their work”.1 Today, those words resonate more than ever as neuroscience embraces artificial intelligence (AI) tools to explore the brain’s mysteries. This trend, fueled by an exponential increase in data volumes, holds tremendous promise for uncovering new insights and driving breakthroughs that were once unimaginable—predicting neurological disorders, restoring lost functions, and developing novel treatments that offer hope to millions of patients everywhere.
Yet, amidst this promise, uncertainties arise about the adherence of AI-driven approaches to rigorous scientific validity standards and their potential effects on established methodological and ethical norms. While many of these are intrinsic to any biomedical use of AI,2 3 4 some challenges gain distinct importance when applied to the human brain and clinical neuroscience, including scientific and clinical validity, considerations of agency, concerns regarding neuroprivacy, and the potential for neurodiscrimination.2
This article provides an overview of current AI-driven approaches in neuroscience and a brief assessment of the ethical challenges surrounding their implementation in clinical settings.
An Immense Potential for Breakthroughs & Innovation
Over the last decades, the use of AI techniques has received growing interest in brain imaging and computational neurosciences, as seen by the exponential growth of scientific publications.5 This growing interest, also observed in the media,6 is partly due to the advantages that computers hold over the human brain, such as precise memory and communication bandwidth. Innovative AI approaches have achieved remarkable results, offering new insights into brain function, diagnostic capabilities, treatment planning, and patient outcome prediction, among numerous other applications.
A perspective paper led by Professor Danilo Bzdok, Canada CIFAR AI Chair and professor at McGill University and Mila, suggests that deep learning models hold the key to unlocking breakthroughs in neuroscience research that human intelligence alone cannot.7 Amidst the burgeoning allure of AI research, neuroscientists are confronted with a similar dilemma faced by professionals across fields: either to harness these powerful technologies in their pursuit of knowledge or to grapple with the looming risk of stagnation as AI continues to gain momentum. The authors argue that large language models (LLMs) specialized in diverse areas of neuroscience could help dismantle academic siloes, leading to the discovery of insights that would otherwise be beyond the reach of human researchers working in isolation from experts in other subfields. These models, which can absorb vast amounts of neuroscientific research, have the potential to foster interdisciplinary dialogue and collaboration by synthesizing knowledge from various perspectives.7
While advancements in recording brain activity have led to vast datasets, translating these into actionable insights for clinical care has remained a challenge. With over 80 billion neurons and 100 trillion connections,8 the human brain processes information and controls behaviour in incredibly complex ways. AI offers a path forward by identifying patterns in intricate multimodal brain data, paving the way for innovative treatments and improved health outcomes. AI models used in neuroscience like LLMs transcend mere text summarization; they can quantify subjective text, resolve linguistic ambiguities, and standardize outputs, thus proving particularly advantageous in interpreting subjective phenomena, such as psychedelic experiences.7 They can help reframe traditional neuroscience research questions to unveil novel insights, facilitate the previously inconceivable integration of disparate information, and pinpoint the most useful features of the brain for investigating the phenomena under study.7 9 10 As for neuroscientists working on animal models, the advancements in AI also serve as a valuable supplement to the field of behavioural assessment.11 12 13 14 15
Other artificial neural networks (ANNs) have demonstrated remarkable success in computer vision tasks, particularly in processing and categorizing extensive image datasets. These include the classification of histopathological images,16 tumor segmentation in brain images,17 and various neuroimaging processing tasks.18 Research led by Nancy Kanwisher, Kalvi Prize winner and Walter A. Rosenblith Professor of Cognitive Neuroscience at the Massachusetts Institute of Technology (MIT), analyzed the patterns in our behaviour that reveal how our brains process facial information. Interestingly, ANNs, specifically convolutional neural networks (CNNs), were able to predict these patterns with impressive accuracy.19 This suggests that the way our brain works when we see faces is quite similar to the way computers process information in CNNs, highlighting the potential of AI in enhancing our understanding of complex cognitive processes.10
Computational experimental settings are particularly beneficial for research that cannot be performed on an actual living human brain, offering a painless way to form and test hypotheses that could be useful in clinical practice. The similarity of results obtained through computational models and those from clinical trials hints at the possibility of capturing fundamental operations of the human brain via ANNs. After all, AI historically owes much to neuroscience, with many significant AI achievements, such as CNNs and reinforcement learning, drawing inspiration from this discipline.9 And now, in turn, AI stands poised to catalyze advancements in neuroscience by providing powerful new models of brain computations, potentially serving as a critical driver for progress.13 Blake Richards, Canada CIFAR AI Chair and professor at McGill University and Mila, emphasizes the importance of understanding how computations in the brain malfunction to effectively treat neurological disorders that alter our thoughts and behaviours.19 He argues that the lessons learned from ANNs can guide us toward understanding the brain as a computational system rather than as a collection of indecipherable cells.20
In line with these ideas, another avenue in which AI serves as a remarkable tool is in prediction and diagnosis. It can enable predictions of treatment responses or the trajectories of mental disorders, assist in anticipating the onset of dementia, assess the likelihood or risk of epileptic seizures, and forecast the fluctuations of disabling movement symptoms in Parkinson’s disease, among other applications.18 In neuroimaging, AI facilitates the analysis of images from various modalities, including MRI and CT scans, supporting the identification of abnormalities, tumors, or structural changes in the brain.21 22
Leveraging deep learning could, therefore, open new avenues to create novel, precisely targeted pharmaceuticals to address neuroimmunological disorders.18 23 24 These new medicines show great promise in combating disorders like multiple sclerosis, which affects both the brain and the immune system. Furthermore, ANNs are advancing the way we handle epileptic seizures.25 By using these networks to continuously monitor brain activity and deliver targeted electrical impulses when needed, we may be able to better predict and manage seizures in real-time, offering a potentially life-changing treatment for epilepsy and related conditions.18 26
AI is further propelling advancements in neurotechnology and interactions between humans and machines. For instance, brain-computer interfaces (BCIs) create a direct connection between a person’s brain and external devices, such as robotic limbs, computers, or speech synthesizers.18 Modern BCIs can adjust their parameters automatically using AI algorithms and are particularly beneficial for patients needing rehabilitation, like those recovering from a stroke, by inducing neural plasticity and restoring lost functions.2 27
These applications highlight the positive impact of AI in neuroscience, from advancing knowledge, prediction, and diagnosis to treatment and neurotechnology. An AI’s ability to parse through vast amounts of data in a short time frame is broadening the scope of neuroscience research, offering new possibilities for understanding and treating neurological disorders worldwide.
Addressing Unanticipated Outcomes
As we explore these exciting avenues, it becomes increasingly important to address the ethical, legal, and social implications (ELSi) on the one hand and the methodological, epistemological, and political challenges that accompany these advancements on the other. This is especially true in clinical settings and contexts that demand sensitive decision-making—whether in the face of imminent and severe risk of harm or when an individual’s choice is to be systematized and applied on a broader scale.
Key concerns include handling incidental findings, consequences for our self-image as autonomous, self-effective agents, or problems of data protection and algorithmic biases,28 all of which can cause cascading effects and profound shifts in societal dynamics over time. While there is evidence that AI can enhance predictive and diagnostic accuracy, there is a lack of clear consensus on its role—whether it should serve as an assistive tool or be granted an autonomous decision-making role.2 The complexity of AI development, involving a broad network of actors—from engineers and developers to basic scientists who may not know each other or operate under the same laws—complicates the assignment of responsibility when negligence leads to patient harm and subsequent legal action against developers.29
AI-assisted decision-making, wherein AI systems provide recommendations and confidence levels while clinicians retain ultimate authority over decisions, represents a rapidly growing paradigm in human-AI collaboration.30 Despite AI’s potential to transform healthcare, clinicians’ perspectives on AI-assisted diagnostic decision-making remain underexplored. This gap is partly due to the infancy of such tools, mistrust from stakeholders, and perceived health risks.31 In high-stakes environments, effective collaboration between humans and AI is particularly challenging, as misaligned trust can compromise decision quality.32
To bridge this gap, it will be crucial to examine the extent of our understanding regarding how the AI reached a particular conclusion and prioritize explainable solutions. An emphasis on explainability might open doors for greater interdisciplinarity and procedural accountability. Just as clinicians rely on laboratory analyses to confirm their diagnoses, AI engineers could validate unexpected results produced by AI systems through a collaborative process, creating safeguards that ensure the systems operate as intended.
While the probabilistic lens of AI may mean that scientists do not always fully grasp its mechanisms, valuable insights can still be generated, driving some form of clinical innovation.7 An example to parallel this trade-off is the creation of Deep Blue [30], the chess algorithm that beat Garry Kasparov for the world championship of chess. To make Deep Blue unbeatable, its creators had to forgo understanding its local, specific game behaviour. Instead, they focused on ensuring its moves would generally lead to winning outcomes, leaving the detailed tactics to the computer’s algorithms.33
However, the ramifications of neuroscience extend far beyond the confines of a mere game of chess, demanding a heightened level of scrutiny, care, and transparency. If an AI-driven discovery, diagnosis, or treatment recommendation cannot be explained, it becomes difficult to justify its use and evaluate its foreseeable risks against the potential benefits. Failing to anticipate risks might further obscure the lines of accountability, where in the absence of clear accountability, there lacks a strong foundation for developing effective countermeasures. Instances have been reported where advanced AI systems, such as LLMs, produce what are known as hallucinations:34 35 that is, they generate content that diverges from the user input, contradicts previously generated context, or misaligns with established world knowledge.36 Building an AI that performs effectively and safely across diverse domains while accounting for emergent risks that engineers never explicitly envisioned remains an ongoing pursuit.
Challenges may arise regarding the protection of brain data, mental privacy, and personal identity from potentially intrusive AI. Although mind-reading has historically been viewed as speculative and often relegated to the realm of science fiction,37 it is becoming increasingly evident that we are approaching a future where real-time decoding of information such as visual perception38 or speech perception39 from brain data is within reach. There is ongoing debate about whether brain data should be treated as a special class, akin to genetic data, and afforded the same protections. Some have proposed the concept of “neurorights” to align brain data with fundamental human rights.40 41 42 Researchers and technology developers should strive to accurately describe their brain-decoding findings to dispel unwarranted concerns and appropriately address those that are justified with precision and clarity to the public and stakeholders.
Crucially, it is important to recognize that the training data fed to AI algorithms may be fraught with limitations and inadvertently perpetuate biases. The majority of behavioural science research has focused primarily on Western, Educated, Industrialized, Rich, and Democratic (WEIRD) participants.43 Neuroscience data is no exception, having historically been biased both in terms of participant selection44 45 46 and research opportunities,44 which further constrains the types of questions that can be explored. In healthcare, some patient experiences and testimonies are given more importance than others. This is known as epistemic injustice, where a person is unfairly treated in their capacity as a knower or epistemic agent.47 48 This may manifest in the marginalization of people with psychiatric illnesses48 or neuroatypical cognitive profiles,49 where prejudices undermine their credibility to give informed consent or fully comprehend the research process. Unfortunately, such exclusions can limit our understanding of the effects of these conditions on the brain, potentially resulting in incomplete or flawed AI systems that fail to adequately account for the diverse range of patient experiences.
The large-scale AI systems we use today are powered by data based on human-derived knowledge, and the ANNs that drive deep learning often require input from human experts for data selection and labeling.18 Conscious and unconscious biases can significantly distort knowledge and have substantial consequences at both individual and societal levels.50 51 52 53 Although many computer scientists and data scientists are now recognizing the problem and working towards ways to mitigate it,54 55 it is essential for neuroscience researchers and clinicians to consider the presence of biases in the sampling data in automated AI systems and other biases that may arise during their design.18 Researchers must critically examine their assumptions, the influence of existing literature on these assumptions, and whether their analyses and reporting contribute to the marginalization or misrepresentation of underrepresented groups. This reflection is essential not only at the level of individual researchers but also at the institutional and societal levels. Although biases cannot be fully eliminated, they can be more effectively managed by acknowledging the limitations inherent in datasets and design processes.
The downstream impacts are particularly worrisome in clinical neuroscience since AI algorithms can process information related to brain function, potentially leading to discrimination based on neurocognitive characteristics. This phenomenon, which has been coined “neurodiscrimination”,2 underlines the need for careful data collection and selection to prevent biases from lowering standards of care for underrepresented patient groups, including those from ethnic minorities or with rare neurological disorders.2 A study revealed that when a clinical AI prediction tool is implemented in a setting with patient population characteristics differing from those in the training data, the AI often performs poorly due to several factors related to patient demographics, standards of care, treatment practices, disease prevalence, and technology use.56
The rush to conform to technology trends sometimes results in the rapid deployment of AI systems, often without conducting the necessary comprehensive testing and evaluation. This accentuates the importance of adopting a meticulous approach to generating high-quality datasets, particularly when dealing with socio-technical or health issues with human lives at risk. It further emphasizes the value of not merely relying on data patterns and ensuring that models are grounded in physical principles and subjective experiences of the affected.
Balancing Progress and Responsibility
The ethical implications of AI remain highly divisive. Influential figures like Yoshua Bengio, a leading world expert in AI and Founder and Scientific Director of Mila, stress the existential risks posed by AI,57 while others adopt a more deflationary stance,58 suggesting that concerns may stem from overinflated optimism about its capabilities. In neuroscience, a targeted approach can help address domain-specific limitations, where the primary challenge lies in the data itself. Studies have found that socioeconomic status, race, ethnicity, and sex contribute to individual differences in neural structure, function, and cognitive performance, making findings from decades of cognitive neuroscience research potentially non-generalizable to a more diverse population.59 As cognitive neuroscience informs education policy and clinical practice, greater diversity in research is a scientific necessity to effectively serve the treatment needs of diverse populations. Equity, diversity, and inclusion (EDI) must be embedded in knowledge production as research disparities persist, with privileged communities often benefiting more from scientific advancements—even when studies focus on underserved groups. A more community-engaged neuroscience approach can help address these inequities by ensuring that the communities under study actively participate in shaping research questions, methodologies, and interpretations.60
An essential step toward inclusivity and reproducibility is through the dissemination of open datasets and code. Examples include those from the Alzheimer’s Disease Neuroimaging Initiative,61 the Human Connectome Project,62 the Cambridge Centre for Ageing and Neuroscience,63 or other open science initiatives, including resources for sharing of neuroscience data like OpenNeuro.64 Furthermore, increasing the accessibility of research findings, educational resources, and technologies—such as articles, courses, and tools—can help bridge gaps in knowledge and ensure equitable access for all communities.
Researchers have been advocating for a cultural shift toward a more data-driven scientific approach with AI, emphasizing its potential to inspire the next generation of neurological treatments, particularly in cases where traditional hypothesis-driven methods are less effective.7 However, data-driven approaches, if not interpretable and value-sensitive, can endanger patients when errors occur. Type I errors (false positives) might lead to false diagnoses, resulting in unnecessary treatments or interventions. Type II errors (false negatives), on the other hand, could lead to missed diagnoses, potentially allowing harmful conditions to go untreated. Given these concerns, until an AI’s statistical accuracy and error margin are satisfactory, it should be used in conjunction with human expertise, prioritizing the increased trustworthiness of AI rather than solely focusing on algorithm performance.5 Establishing a symbiotic relationship between humans and AI predictive algorithms, with human experts optimizing and fine-tuning them, appears to be a key objective.65 AI-based software would, therefore, ideally undergo thorough safety and efficacy assessments, and clear guidelines should be established for modifying the software post-approval to account for any unforeseen deviations in its functioning compared to its initial approval.66
Despite the high risks and rewards discussed, the expected productivity increase following the adoption of new technology has historically encountered delays, known as the “productivity paradox” in the medical field.56 The integration of AI clinical technologies is likely to disrupt workflows in unforeseen ways, introducing new types of human errors and system failures in the years to come. Navigating these challenges demands a unified effort: physicians, clinical support staff, management, and technical staff are all called to play a role in selecting, monitoring, and continuously maintaining clinical AI prediction tools.56 Fostering adaptive agility through critical thinking is indispensable and will require not only interdisciplinary teams but also the early incorporation of a diverse knowledge toolkit into AI and natural science curricula. Researchers believe we need the coming generations of neuroscientists, programmers, engineers, and other specialists to add ethical thinking and analysis into their methodological toolbox.18
There are still numerous topics left to explore with regard to AI in neuroscience, such as brain augmentation, risks of adversarial attacks, patient trust, social and cultural acceptance, the influence of conflicts of interest in shaping the trajectory of scientific discovery, shifting power dynamics, widening gaps in access to AI technologies, alignment with sustainable development goals (SDGs), and more. These highlight the multifaceted nature of the challenges and opportunities within this field. The wide-ranging applications of AI would need tailored regulations and ethical frameworks that acknowledge the various contexts in which it is used. By taking a contextualized, case-study, multi-stakeholder approach to analyze the issues of specific practices, we can ensure more equitable benefits and robust protocols tailored to the unique capabilities of AI models.29
Conclusion
AI holds great promise for fostering interdisciplinary dialogue and catalyzing advancements in neuroscience. Exploring the capabilities of AI and driving forward its development can vastly enhance our understanding of the brain and contribute to the diagnosis and treatment of neurological diseases. But in doing so, addressing ethical and methodological challenges is critical for its responsible and beneficial use.
As AI continues to advance data processing, responsibilities may include upholding the highest standards in data collection and management and building trustworthy and reliable AI algorithms. This can be achieved through an ethics-by-design approach,67 68 supported by adaptive and continuous evaluation mechanisms and a unified framework for large-scale AI in neuroscience that integrates insights from all the involved disciplines. Addressing these challenges will require interdisciplinary collaboration among neuroscientists, ethicists, computer scientists, social scientists, policymakers, and affected communities.18 56 Despite the magnitude of these concerns, they can be managed and should not deter progress, as AI-driven approaches have the potential to positively impact lives4 and shed light on crucial areas for further research.
While daunting, these challenges present neuroscientists with an opportunity to contribute meaningfully to their field and society. Through collective and concerted efforts, we can forge a path that minimizes the possibility of missteps in AI implementation. Rather than relying solely on legislation to keep pace with innovation, it is within our ability to contribute to safe and equitable outcomes. This begins with active engagement in raising awareness about the challenges and potential limitations in research and innovation, ensuring that these considerations are integrated at every stage of development to mitigate the risks and reap the benefits these tools offer.
Sources
- “Does neuroscience threaten human values?,” Nat. Neurosci., vol. 1, no. 7, pp. 535–536, Nov. 1998, doi: 10.1038/2878. ↩︎
- M. Ienca and K. Ignatiadis, “Artificial Intelligence in Clinical Neuroscience: Methodological and Ethical Challenges,” AJOB Neurosci., vol. 11, no. 2, pp. 77–87, Apr. 2020, doi: 10.1080/21507740.2020.1740352. ↩︎
- D. S. Char, N. H. Shah, and D. Magnus, “Implementing Machine Learning in Health Care – Addressing Ethical Challenges,” N. Engl. J. Med., vol. 378, no. 11, pp. 981–983, Mar. 2018, doi: 10.1056/NEJMp1714229. ↩︎
- E. J. Topol, “High-performance medicine: the convergence of human and artificial intelligence,” Nat. Med., vol. 25, no. 1, Art. no. 1, Jan. 2019, doi: 10.1038/s41591-018-0300-7. ↩︎
- A. Segato, A. Marzullo, F. Calimeri, and E. De Momi, “Artificial intelligence for brain diseases: A systematic review,” APL Bioeng., vol. 4, no. 4, p. 041503, Oct. 2020, doi: 10.1063/5.0011697. ↩︎
- H. Devlin and H. D. S. correspondent, “AI makes non-invasive mind-reading possible by turning thoughts into text,” The Guardian, May 01, 2023. Accessed: Feb. 10, 2024. [Online]. Available: https://www.theguardian.com/technology/2023/may/01/ai-makes-non-invasive-mind-reading-possible-by-turning-thoughts-into-text ↩︎
- D. Bzdok, A. Thieme, O. Levkovskyy, P. Wren, T. Ray, and S. Reddy, “Data science opportunities of large language models for neuroscience and biomedicine,” Neuron, Feb. 2024, doi: 10.1016/j.neuron.2024.01.016. ↩︎
- S. Herculano-Houzel, “The remarkable, yet not extraordinary, human brain as a scaled-up primate brain and its associated cost,” Proc. Natl. Acad. Sci., vol. 109, no. supplement_1, pp. 10661–10668, Jun. 2012, doi: 10.1073/pnas.1201895109. ↩︎
- A. Zador et al., “Catalyzing next-generation Artificial Intelligence through NeuroAI,” Nat. Commun., vol. 14, no. 1, Art. no. 1, Mar. 2023, doi: 10.1038/s41467-023-37180-x. ↩︎
- A. Doerig et al., “The neuroconnectionist research programme,” Nat. Rev. Neurosci., vol. 24, no. 7, pp. 431–450, Jul. 2023, doi: 10.1038/s41583-023-00705-w. ↩︎
- S. W. Flavell, N. Gogolla, M. Lovett-Barron, and M. Zelikowsky, “The emergence and influence of internal states,” Neuron, vol. 110, no. 16, pp. 2545–2570, Aug. 2022, doi: 10.1016/j.neuron.2022.04.030. ↩︎
- P. Bao, L. She, M. McGill, and D. Y. Tsao, “A map of object space in primate inferotemporal cortex,” Nature, vol. 583, no. 7814, pp. 103–108, Jul. 2020, doi: 10.1038/s41586-020-2350-5. ↩︎
- B. Richards, D. Tsao, and A. Zador, “The application of artificial intelligence to biology and neuroscience,” Cell, vol. 185, no. 15, pp. 2640–2643, Jul. 2022, doi: 10.1016/j.cell.2022.06.047. ↩︎
- A. Mathis et al., “DeepLabCut: markerless pose estimation of user-defined body parts with deep learning,” Nat. Neurosci., vol. 21, no. 9, pp. 1281–1289, Sep. 2018, doi: 10.1038/s41593-018-0209-y. ↩︎
- D. Lin, A. Z. Huang, and B. A. Richards, “Temporal encoding in deep reinforcement learning agents,” Sci. Rep., vol. 13, no. 1, p. 22335, Dec. 2023, doi: 10.1038/s41598-023-49847-y. ↩︎
- G. Litjens et al., “Deep learning as a tool for increased accuracy and efficiency of histopathological diagnosis,” Sci. Rep., vol. 6, no. 1, Art. no. 1, May 2016, doi: 10.1038/srep26286. ↩︎
- S. Pereira, A. Pinto, V. Alves, and C. A. Silva, “Brain Tumor Segmentation Using Convolutional Neural Networks in MRI Images,” IEEE Trans. Med. Imaging, vol. 35, no. 5, pp. 1240–1251, May 2016, doi: 10.1109/TMI.2016.2538465. ↩︎
- P. Kellmeyer, “Artificial Intelligence in Basic and Clinical Neuroscience: Opportunities and Ethical Challenges,” Neuroforum, vol. 25, no. 4, pp. 241–250, Nov. 2019, doi: 10.1515/nf-2019-0018. ↩︎
- K. Dobs, J. Yuan, J. Martinez, and N. Kanwisher, “Behavioral signatures of face perception emerge in deep neural networks optimized for face recognition,” Proc. Natl. Acad. Sci., vol. 120, no. 32, p. e2220642120, Aug. 2023, doi: 10.1073/pnas.2220642120. ↩︎
- “Designing artificial brains can help us learn more about real ones,” The Neuro. Accessed: May 21, 2024. [Online]. Available: https://www.mcgill.ca/neuro/article/research/designing-artificial-brains-can-help-us-learn-more-about-real-ones ↩︎
- R. Mehrotra, M. A. Ansari, R. Agrawal, and R. S. Anand, “A Transfer Learning approach for AI-based classification of brain tumors,” Mach. Learn. Appl., vol. 2, p. 100003, Dec. 2020, doi: 10.1016/j.mlwa.2020.100003. ↩︎
- R. F. Mansour, J. Escorcia-Gutierrez, M. Gamarra, V. G. DĂaz, D. Gupta, and S. Kumar, “Artificial intelligence with big data analytics-based brain intracranial hemorrhage e-diagnosis using CT images,” Neural Comput. Appl., vol. 35, no. 22, pp. 16037–16049, Aug. 2023, doi: 10.1007/s00521-021-06240-y. ↩︎
- R. Bonacchi, M. Filippi, and M. A. Rocca, “Role of artificial intelligence in MS clinical practice,” NeuroImage Clin., vol. 35, p. 103065, Jan. 2022, doi: 10.1016/j.nicl.2022.103065. ↩︎
- M. Popova, O. Isayev, and A. Tropsha, “Deep reinforcement learning for de novo drug design,” Sci. Adv., vol. 4, no. 7, p. eaap7885, Jul. 2018, doi: 10.1126/sciadv.aap7885. ↩︎
- S. Saminu et al., “Applications of Artificial Intelligence in Automatic Detection of Epileptic Seizures Using EEG Signals: A Review,” Artif. Intell. Appl., vol. 1, no. 1, Art. no. 1, 2023, doi: 10.47852/bonviewAIA2202297. ↩︎
- A. Berényi, M. Belluscio, D. Mao, and G. Buzsáki, “Closed-Loop Control of Epilepsy by Transcranial Electrical Stimulation,” Science, vol. 337, no. 6095, pp. 735–737, Aug. 2012, doi: 10.1126/science.1223154. ↩︎
- W. Jaber, H. A. H. Jaber, R. Jaber, and Z. Saleh, “The Convergence of AI and BCIs: A New Era of Brain-Machine Interfaces,” in Artificial Intelligence in the Age of Nanotechnology, IGI Global, 2024, pp. 98–113. doi: 10.4018/979-8-3693-0368-9.ch006. ↩︎
- W. Wiese and K. J. Friston, “AI ethics in computational psychiatry: From the neuroscience of consciousness to the ethics of consciousness,” Behav. Brain Res., vol. 420, p. 113704, Feb. 2022, doi: 10.1016/j.bbr.2021.113704. ↩︎
- A. D. Saenz, Z. Harned, O. Banerjee, M. D. Abrà moff, and P. Rajpurkar, “Autonomous AI systems in the face of liability, regulations and costs,” Npj Digit. Med., vol. 6, no. 1, Art. no. 1, Oct. 2023, doi: 10.1038/s41746-023-00929-1. ↩︎
- X. Wang, Z. Lu, and M. Yin, “Will You Accept the AI Recommendation? Predicting Human Behavior in AI-Assisted Decision Making,” in Proceedings of the ACM Web Conference 2022, Virtual Event, Lyon France: ACM, Apr. 2022, pp. 1697–1708. doi: 10.1145/3485447.3512240. ↩︎
- H. Hah and D. S. Goldin, “How Clinicians Perceive Artificial Intelligence–Assisted Technologies in Diagnostic Decision Making: Mixed Methods Approach,” J. Med. Internet Res., vol. 23, no. 12, p. e33540, Dec. 2021, doi: 10.2196/33540. ↩︎
- M. Steyvers and A. Kumar, “Three Challenges for AI-Assisted Decision-Making,” Perspect. Psychol. Sci., vol. 19, no. 5, pp. 722–734, Sep. 2024, doi: 10.1177/17456916231181102. ↩︎
- M. Campbell, A. J. Hoane, and F. Hsu, “Deep Blue,” Artif. Intell., vol. 134, no. 1, pp. 57–83, Jan. 2002, doi: 10.1016/S0004-3702(01)00129-1. ↩︎
- N. B. Yudkowsky Eliezer, “The Ethics of Artificial Intelligence,” in Artificial Intelligence Safety and Security, Chapman and Hall/CRC, 2018. ↩︎
- J. Kaddour, J. Harris, M. Mozes, H. Bradley, R. Raileanu, and R. McHardy, “Challenges and Applications of Large Language Models,” arXiv.org. Accessed: Feb. 22, 2024. [Online]. Available: https://arxiv.org/abs/2307.10169v1 ↩︎
- “Large language models and the perils of their hallucinations | Critical Care | Full Text.” Accessed: Feb. 22, 2024. [Online]. Available: https://ccforum.biomedcentral.com/articles/10.1186/s13054-023-04393-x ↩︎
- M. J. Farah, “Emerging ethical issues in neuroscience,” Nat. Neurosci., vol. 5, no. 11, pp. 1123–1129, Nov. 2002, doi: 10.1038/nn1102-1123. ↩︎
- Y. Benchetrit, H. Banville, and J.-R. King, “Brain decoding: toward real-time reconstruction of visual perception,” Oct. 18, 2023, arXiv.org. arXiv:2310.19812. doi: 10.48550/arXiv.2310.19812. ↩︎
- A. Défossez, C. Caucheteux, J. Rapin, O. Kabeli, and J.-R. King, “Decoding speech perception from non-invasive brain recordings,” Nat. Mach. Intell., vol. 5, no. 10, pp. 1097–1107, Oct. 2023, doi: 10.1038/s42256-023-00714-5. ↩︎
- J. C. Bublitz, “Novel Neurorights: From Nonsense to Substance,” Neuroethics, vol. 15, no. 1, p. 7, Feb. 2022, doi: 10.1007/s12152-022-09481-3. ↩︎
- M. Ienca, “On Neurorights,” Front. Hum. Neurosci., vol. 15, 2021, Accessed: Feb. 18, 2024. [Online]. Available: https://www.frontiersin.org/articles/10.3389/fnhum.2021.701258 ↩︎
- N. Hertz, “Neurorights – Do we Need New Human Rights? A Reconsideration of the Right to Freedom of Thought,” Neuroethics, vol. 16, no. 1, p. 5, Nov. 2022, doi: 10.1007/s12152-022-09511-0. ↩︎
- J. Henrich, S. J. Heine, and A. Norenzayan, “The weirdest people in the world?,” Behav. Brain Sci., vol. 33, no. 2–3, pp. 61–83, Jun. 2010, doi: 10.1017/S0140525X0999152X. ↩︎
- T. R. Will et al., “Problems and Progress regarding Sex Bias and Omission in Neuroscience Research,” eNeuro, vol. 4, no. 6, Nov. 2017, doi: 10.1523/ENEURO.0278-17.2017. ↩︎
- A. K. Beery and I. Zucker, “Sex bias in neuroscience and biomedical research,” Neurosci. Biobehav. Rev., vol. 35, no. 3, pp. 565–572, Jan. 2011, doi: 10.1016/j.neubiorev.2010.07.002. ↩︎
- T. C. Parker and J. A. Ricard, “Structural racism in neuroimaging: perspectives and solutions,” Lancet Psychiatry, vol. 9, no. 5, p. e22, May 2022, doi: 10.1016/S2215-0366(22)00079-7. ↩︎
- M. Fricker, Epistemic injustice: Power and the ethics of knowing. Oxford University Press, 2007. Accessed: Feb. 22, 2024. [Online]. Available: Google Books ↩︎
- I. J. Kidd, L. Spencer, and H. Carel, “Epistemic injustice in psychiatric research and practice,” Philos. Psychol., vol. 0, no. 0, pp. 1–29, 2022, doi: 10.1080/09515089.2022.2156333. ↩︎
- M. Legault, J.-N. Bourdon, and P. Poirier, “From neurodiversity to neurodivergence: the role of epistemic and cognitive marginalization,” Synthese, vol. 199, no. 5, pp. 12843–12868, Dec. 2021, doi: 10.1007/s11229-021-03356-5. ↩︎
- J. Stone and G. B. Moskowitz, “Non-conscious bias in medical decision making: what can be done to reduce it?,” Med. Educ., vol. 45, no. 8, pp. 768–776, 2011, doi: 10.1111/j.1365-2923.2011.04026.x. ↩︎
- J. R. Marcelin, D. S. Siraj, R. Victor, S. Kotadia, and Y. A. Maldonado, “The Impact of Unconscious Bias in Healthcare: How to Recognize and Mitigate It,” J. Infect. Dis., vol. 220, no. Supplement_2, pp. S62–S73, Aug. 2019, doi: 10.1093/infdis/jiz214. ↩︎
- C. FitzGerald and S. Hurst, “Implicit bias in healthcare professionals: a systematic review,” BMC Med. Ethics, vol. 18, no. 1, p. 19, Mar. 2017, doi: 10.1186/s12910-017-0179-8. ↩︎
- B. R. Newell and D. R. Shanks, “Unconscious influences on decision making: A critical review,” Behav. Brain Sci., vol. 37, no. 1, pp. 1–19, Feb. 2014, doi: 10.1017/S0140525X12003214. ↩︎
- I. Y. Chen, “Machine Learning Approaches for Equitable Healthcare,” Thesis, Massachusetts Institute of Technology, 2022. Accessed: May 29, 2024. [Online]. Available: https://dspace.mit.edu/handle/1721.1/147451 ↩︎
- R. Courtland, “Bias detectives: the researchers striving to make algorithms fair,” Nature, vol. 558, no. 7710, pp. 357–360, Jun. 2018, doi: 10.1038/d41586-018-05469-3. ↩︎
- S. Monteith, T. Glenn, J. R. Geddes, E. D. Achtyes, P. C. Whybrow, and M. Bauer, “Challenges and Ethical Considerations to Successfully Implement Artificial Intelligence in Clinical Medicine and Neuroscience: a Narrative Review,” Pharmacopsychiatry, vol. 56, no. 6, pp. 209–213, Nov. 2023, doi: 10.1055/a-2142-9325. ↩︎
- Y. Bengio, “AI and Catastrophic Risk,” J. Democr., vol. 34, no. 4, pp. 111–121, 2023. ↩︎
- J. Maclure, “The new AI spring: a deflationary view,” AI Soc., vol. 35, no. 3, pp. 747–750, Sep. 2020, doi: 10.1007/s00146-019-00912-z. ↩︎
- V. M. Dotson and A. Duarte, “The importance of diversity in cognitive neuroscience,” Ann. N. Y. Acad. Sci., vol. 1464, no. 1, pp. 181–191, 2020, doi: 10.1111/nyas.14268. ↩︎
- S. La Scala, J. L. Mullins, R. B. Firat, Emotional Learning Research Community Advisory Board, and K. J. Michalska, “Equity, diversity, and inclusion in developmental neuroscience: Practical lessons from community-based participatory research,” Front. Integr. Neurosci., vol. 16, Mar. 2023, doi: 10.3389/fnint.2022.1007249. ↩︎
- C. R. Jack Jr. et al., “The Alzheimer’s disease neuroimaging initiative (ADNI): MRI methods,” J. Magn. Reson. Imaging, vol. 27, no. 4, pp. 685–691, 2008, doi: 10.1002/jmri.21049. ↩︎
- D. C. Van Essen, S. M. Smith, D. M. Barch, T. E. J. Behrens, E. Yacoub, and K. Ugurbil, “The WU-Minn Human Connectome Project: An overview,” NeuroImage, vol. 80, pp. 62–79, Oct. 2013, doi: 10.1016/j.neuroimage.2013.05.041. ↩︎
- J. R. Taylor et al., “The Cambridge Centre for Ageing and Neuroscience (Cam-CAN) data repository: Structural and functional MRI, MEG, and cognitive data from a cross-sectional adult lifespan sample,” NeuroImage, vol. 144, pp. 262–269, Jan. 2017, doi: 10.1016/j.neuroimage.2015.09.018. ↩︎
- C. J. Markiewicz et al., “The OpenNeuro resource for sharing of neuroscience data,” eLife, vol. 10, p. e71774, Oct. 2021, doi: 10.7554/eLife.71774. ↩︎
- M. Pedersen, K. Verspoor, M. Jenkinson, M. Law, D. F. Abbott, and G. D. Jackson, “Artificial intelligence for clinical decision support in neurology,” Brain Commun., vol. 2, no. 2, p. fcaa096, Jul. 2020, doi: 10.1093/braincomms/fcaa096. ↩︎
- T. J. Hwang, A. S. Kesselheim, and K. N. Vokinger, “Lifecycle Regulation of Artificial Intelligence– and Machine Learning–Based Software Devices in Medicine,” JAMA, vol. 322, no. 23, pp. 2285–2286, Dec. 2019, doi: 10.1001/jama.2019.16842. ↩︎
- S. Koseki, S. Jameson, G. Farnadi, D. Rolnick, C. Régis, and J. L. Denis, “AI and Cities: Risks, Applications, and Governance.” UN-Habitat Nairobi, Kenya, 2022. ↩︎
- P. Brey and B. Dainow, “Ethics by design for artificial intelligence,” AI Ethics, vol. 4, no. 4, pp. 1265–1277, Nov. 2024, doi: 10.1007/s43681-023-00330-4. ↩︎