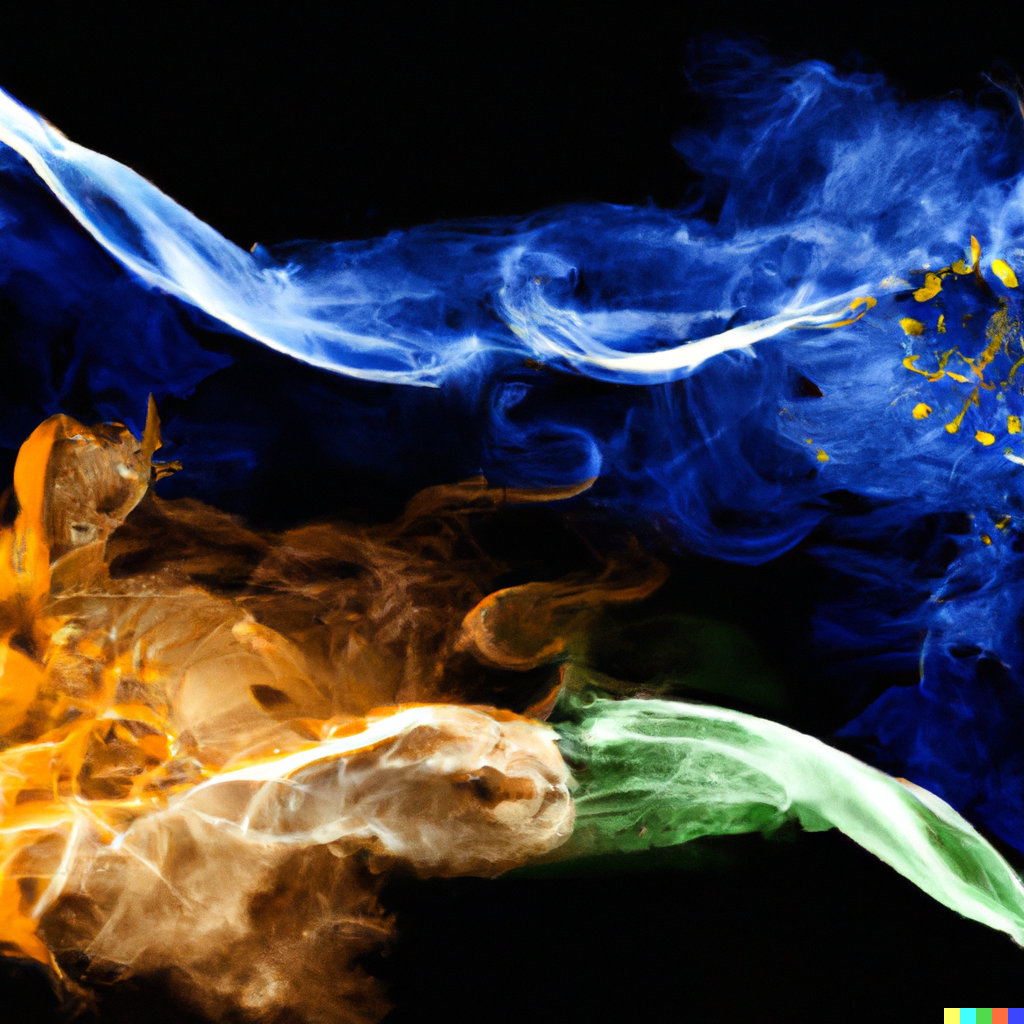
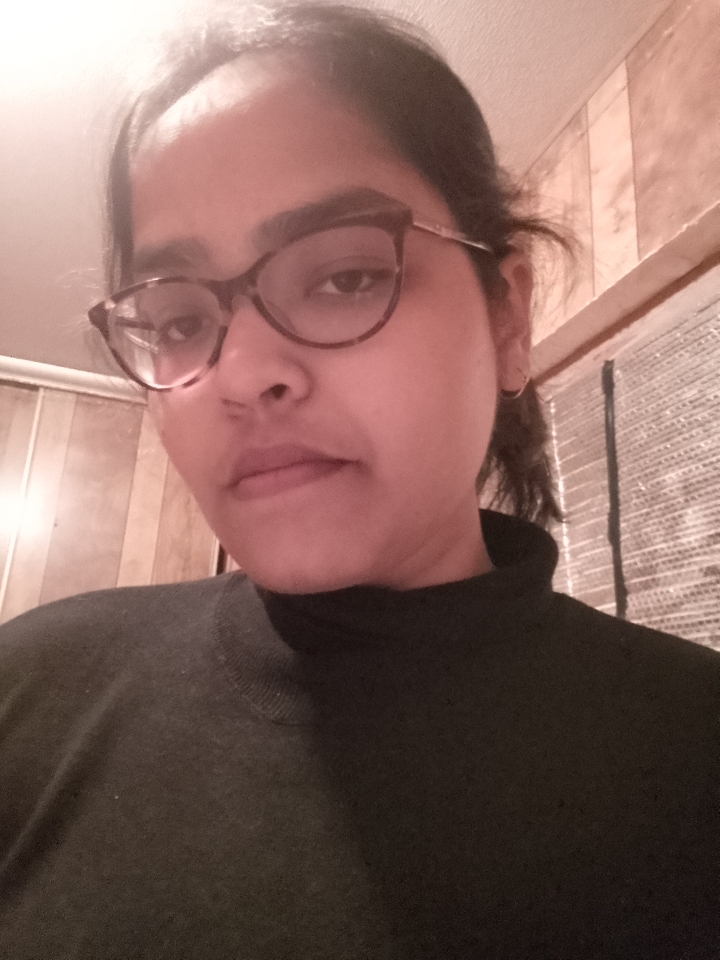
🔬 Research Summary by Vishakha Agrawal, an independent researcher interested in human-AI collaboration, participatory AI and AI safety.
[Original paper by Vishakha Agrawal, Serhiy Kandul, Markus Kneer, and Markus Christen]
Overview: AI-based decision support systems are increasingly being incorporated in various applications. In certain contexts, people seem to trust AI more than humans, while in others, the acceptability of AI-generated advice remains low. In this paper, we make cross-cultural comparisons of trust, responsibility, and reliance of people on a human vs. an AI system upon receiving advice for making high-stakes decisions.
Introduction
What factors influence people’s preferences for and against AI decision support? One line of research links the variation in attitudes towards AI to cultural differences such as mistrust in algorithms due to historical discrimination, different levels of exposure and public image of the technology, attitudes towards risk and uncertainty, differences across Hofstede’s individualism-collectivism dimension[1], and so on. The research on AI perceptions predominantly relies on Western samples, and sample sizes from the Global South continue to remain low. Furthermore, insights emerging from general attitude scales are limited if not combined with a task-based assessment and might not be applicable in human-robot interaction (HRI) contexts.
We devised an interactive, task-based experimental paradigm complemented by a series of state-of-the-art scales. We consider decisions involving minimizing casualties (defense domain) or maximizing lives saved (search and rescue domain) with AI or human support to compare an OECD and an Indian sample. We explore three key variables of recent research in HRI: trust in the capacities of the AI-based application, reliance as a behavioral measure capturing whether people rely on the recommendations of a human or AI-driven advisor system, and the extent to which people assume moral responsibility for their actions and the consequences they engender.
We find that OECD participants consider humans less capable but more morally trustworthy and responsible than AI. In contrast, Indian participants trust humans more than AI but assign equal responsibility to both types of experts.
Key Insights
Experimental Design
The study consisted of an experiment on the crowdsourcing platforms Prolific for OECD participants (n=351) and Mechanical Turk for Indian participants (n=302). The flow of the experiment was as follows:
- After participants accepted the task on these crowdsourcing platforms, they were sent to play a simulation on a web app.
- They first received training and then were sent to perform four missions. There were four scenarios, and each participant was randomly assigned one of these scenarios: maximize lives saved or minimize lives lost. Each of these scenarios had human-in-the-loop or human-on-the-loop conditions.
- The participants were asked to complete a consent form, enter their crowdsource platform worker ID and pass an attention test.
- Then, they went through a training phase to ensure they understood their role and task; failure to understand the simulation mechanics led to exclusion.
- Collecting demographic information and measuring risk preference, cognitive thinking skills, and statistical thinking skills were integrated in the training narrative. Cognitive and statistical thinking skills served as controls.
- After the training, the participants were confronted with two missions with four decision problems each, once advised by a human expert and once by an AI in a random order. For every decision problem, we presented three available options. The participant had 30 seconds to decide. The choices presented a conflict between maximizing the expected value or maximizing the probability of helping at least somebody (or minimizing the probability of hurting somebody). The experts’ recommendations were balanced with respect to those two types of choices.
- Reliance was measured by analyzing whether the participants followed the expert’s advice or chose a different option.
- At the end of each mission, the participants answered questions about how much they thought they, the AI, the human expert, the programmer of AI, and the seller of AI were responsible for the outcome on a seven-point Likert scale.
- After the missions, we presented the participants with two engagement questions.
- We also measured their affinity with technology interaction, utilitarian preference, and trust in the AI and the human expert using a 16-item version of the self-reported Multi-Dimensional Measure of Trust scale (MDMT) comprising the capacity trust and moral trust subscale.
- The participants were then sent back to the crowdsourcing platforms for payment.
Results
We compare the results on trust, perceived responsibility, and reliance on the experts. For each dependent variable, we start with two-way mixed ANOVA for the sample (OECD vs. India, between-group) and expert type (AI vs. Human, within-group). We report effect sizes followed by random effect regressions with or without participants’ characteristics as control variables.
Trust: We find small, though significant, differences in overall and moral trust across populations. The difference in capacity trust seems the most pronounced, with participants from India vesting more trust in humans, whereas participants from OECD countries more in AI advisors.
Responsibility: The responsibility assumed by participants was high in both conditions and did not differ significantly across samples. However, whereas OECD participants were relatively unwilling to attribute responsibility to an AI advisor, its programmer, or producer, the mean responsibility attributions for all three were high in India.
Reliance: For reliance on expert advice, we found an interaction between scenario type and culture. However, there was little difference in advice preference for either type of expert (human or AI) in either culture.
Between the lines
Overall, there is considerable convergence across cultures, except that Indians hold AI programmers and producers and the AI itself responsible to much higher degrees than OECD participants. One hypothesis could be that the collective that is deemed responsible includes AI, too. Considering the lack of previous research with Indian participants, it is difficult to assess how plausible this hypothesis is. Responsibility attribution in human-AI teams across the East/West divide does, however, constitute an interesting avenue for further research.
References
[1] https://www.hofstede-insights.com/country-comparison-tool