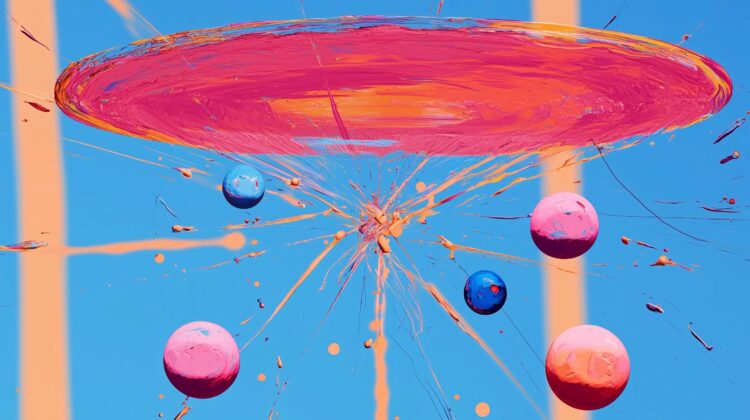
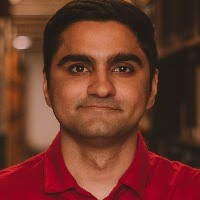
🔬 Research Summary by ✍️ Usman Gohar and Zeerak Talat.
Usman Gohar is a Computer Science Ph.D. candidate at Iowa State University studying AI safety and Algorithmic Fairness.
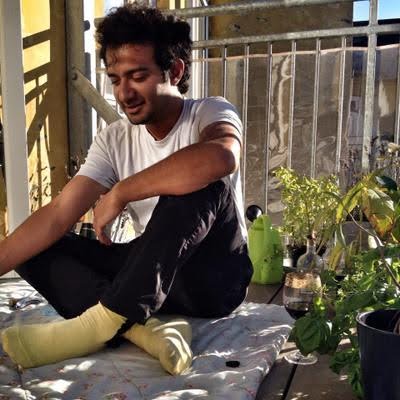
Zeerak Talat is a Chancellor’s Fellow in Responsible Machine Learning and Artificial Intelligence at the University of Edinburgh who studies the ethics and politics of machine learning.
[Original Paper by Irene Solaiman, Zeerak Talat, William Agnew, Lama Ahmad, Dylan Baker, Su Lin Blodgett, Canyu Chen, Hal Daumé III, Jesse Dodge, Isabella Duan, Ellie Evans, Felix Friedrich, Avijit Ghosh, Usman Gohar, Sara Hooker, Yacine Jernite, Ria Kalluri, Alberto Lusoli, Alina Leidinger, Michelle Lin, Xiuzhu Lin, Sasha Luccioni, Jennifer Mickel, Margaret Mitchell, Jessica Newman, Anaelia Ovalle, Marie-Therese Png, Shubham Singh, Andrew Strait, Lukas Struppek, Arjun Subramonian]
Overview: Generative AI models across text, image, and video modalities have advanced rapidly, and research has highlighted their wide-ranging social impacts. Yet, no standard framework exists for evaluating these impacts or determining what should be assessed. Our framework identifies various categories of social impacts, such as bias, privacy, and environmental costs, while discussing evaluation methods tailored to these concerns. By analyzing limitations in current approaches and providing actionable recommendations, we aim to lay the groundwork for standardized, context-sensitive evaluations of generative AI systems.
Introduction
Understanding the social impacts of AI systems—from conception to deployment—requires examining factors like training data, model design, infrastructure, and societal context. It also involves analyzing how these systems influence societal processes, institutions, and power dynamics. Yet, many existing evaluations of generative AI systems are narrow, often overlooking critical aspects. For instance, did you know that generating an image with AI consumes as much energy as charging your smartphone? We define social impact as the effect of a system on people and society with a focus on active, measurable, harmful impacts. With the increasing use of generative AI systems for diverse tasks, social impact evaluations have become essential, yet no widely accepted standard exists.
This work aims to advance a standardized approach by proposing a framework to evaluate the social impacts of generative AI systems across five modalities: text (including language and code), image, video, audio, and multimodal combinations. Convening in workshops, the authors propose a framework that distinguishes between “base systems” with no predetermined application and “people and society,” focusing on interactions with individuals and communities.
For base systems, we identify seven categories of social impact, including bias and privacy, while for societal context, we highlight five categories, such as trustworthiness and creativity. Our framework (see Figure 1) aims to improve understanding of social impact, informing appropriate use in diverse contexts. By offering both quantitative and qualitative insights, we seek to make social impact evaluations more accessible for researchers, developers, auditors, and policymakers.Â
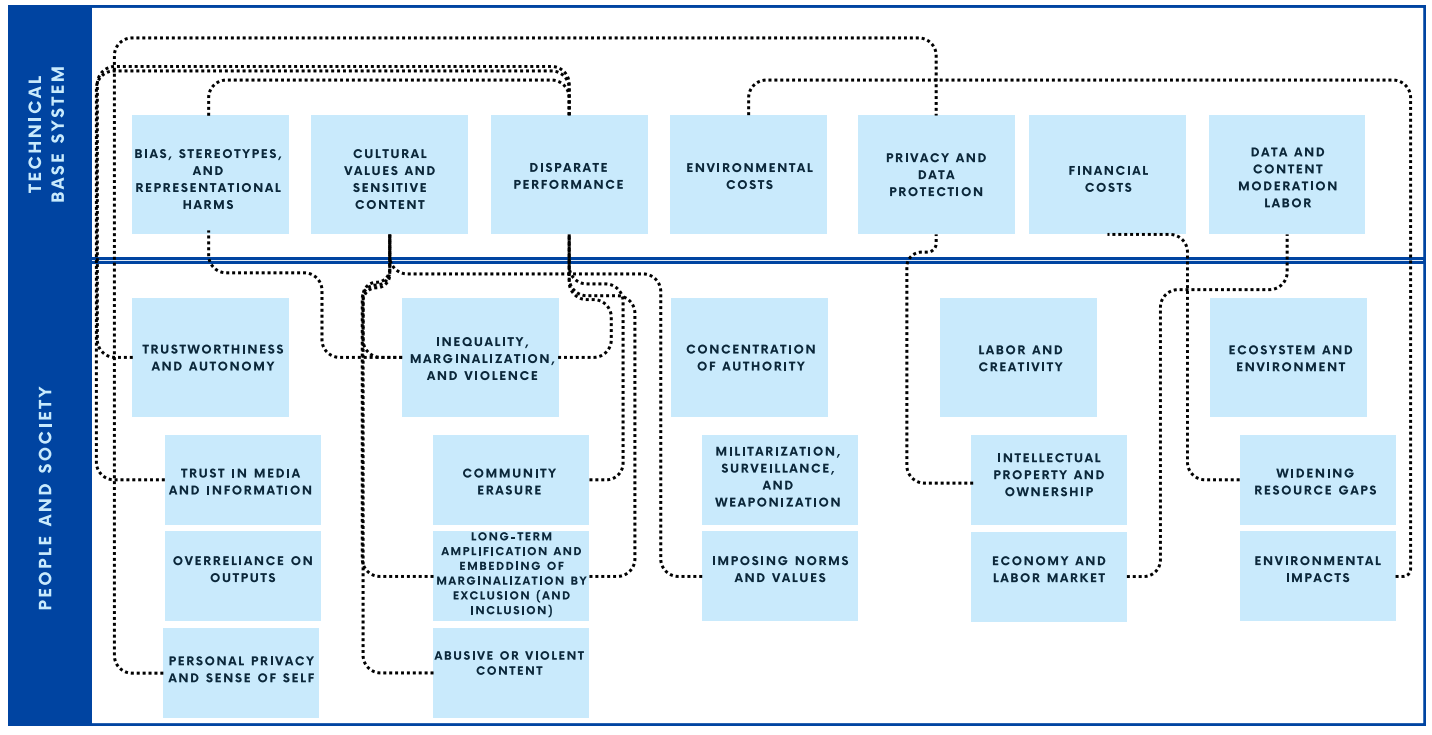
Impacts: Technical Base Systems
Technical Base Systems refer to AI systems that have no predetermined application, including models and their components. This makes it harder to evaluate them as the scope of social impact harms can vary vastly and cannot be comprehensively captured. This category evaluates the various social harms the development of such systems have regardless of what they are used for. However, our framework is designed to be extensible and flexible and is proposed as a living document for this purpose.
For base systems, we identify the following seven high-level non-exhaustive categories:
- Bias, Stereotypes, and Representational Harms
- Cultural Values and Sensitive Content
- Disparate Performance
- Environmental Costs and Carbon Emissions
- Privacy and Data Protection
- Financial Costs
- Data and Content Moderation Labor
For each of these categories, we present a synthesis of the findings across different modalities in the paper, including what needs to be evaluated and the current limitations of Generative AI evaluation practices. For example, some current evaluations overfit certain lenses and geographies, such as evaluating a multilingual system only in the English language (see the full paper for further discussion and nuances of social impact).
Limitations:
In the paper, the authors find that several overarching challenges emerge when evaluating generative AI systems across these categories, reflecting common issues. One of the consistent issues plaguing current evaluations is the lack of transparency. Across all categories, there is insufficient documentation, whether it pertains to labor conditions, resource use, or privacy practices.
For example, accurate estimation of environmental costs is currently hamstrung by a lack of information on energy consumption from equipment manufacturers and data/hosting centers. This lack of clarity hinders accountability and informed evaluations.
Next, it was commonly identified that there is insufficient participation of marginalized communities, often the ones most affected by AI systems, in the design and evaluation processes. This leads to frameworks that inadequately reflect their needs and perspectives.
Finally, the contextual nature of AI systems—spanning cultural, societal, and technical environments—makes standardized evaluations difficult. Nuances such as intersectionality, cultural diversity, and regional differences often require localized approaches, which are underdeveloped. We refer the readers to the full paper for contextualized and technical limitations of current practices for individual categories.
Impacts: People and Society
In contrast to the technical base system, the social impact evaluations focus on the impact of AI systems and what can be evaluated in the interactions of generative AI systems with people and society. These impacts cannot be measured in isolation as these are born out of the interaction of society with these systems. Such evaluations examine the systems in the broader societal context, e.g., trust in model outputs (fact-checking), loss of jobs, etc. The scale and scope of generative AI technologies necessarily mean they interact with national and global social systems, including economies, politics, and cultures.
The following non-exhaustive categories were identified, which are heavily influenced by the deployment environment:
- Trustworthiness and Autonomy
- Inequality, Marginalization, and Violence
- Concentration of Authority
- Labor and Creativity
- Ecosystem and Environment
Summary of Major Concerns:
A broad range of contextual concerns were identified across the five categories (see full paper). Here, we discuss some common threads.
Equity and Access: Generative AI’s advantages, such as enhanced productivity and innovation, are not evenly distributed. Wealthier nations, industries, and individuals gain the most, leaving marginalized and low-resource groups at a disadvantage. Secondly, high resource costs (e.g., compute power) exclude underrepresented communities, researchers, and smaller organizations from meaningful participation.
Transparency and Accountability: The lack of clarity about training data, ownership, and operational decisions raises concerns about fairness and intellectual property violations, such as reputational damage and economic loss.
Concentration of Authority: Generative AI can enhance cyberattacks, disinformation campaigns, and surveillance, concentrating power among a few actors, often under non-transparent terms. Moreover, models trained in one cultural context may impose external norms, marginalizing local languages, values, and identities.
Between the lines
Evaluating generative AI systems requires more than technical assessments—it demands understanding their societal impacts and the context of their deployment. Our findings emphasize the critical need for inclusive, context-specific evaluations considering diverse cultural values, marginalized communities, and overlooked regions.
Despite progress, gaps remain. Social impact evaluations often lack depth and fail to adequately center the least powerful in society. Moreover, the absence of standardized, universally applicable evaluation frameworks leaves critical questions unanswered: How do we define harm across contexts? How can evaluations adapt to evolving cultural norms? Other open-ended questions also remain unanswered: How distinct should frameworks and suites be on specific components of AI safety? How can we incentivize progress when we’ll never “solve” many of these categories? How should conflicting values be reckoned? What should be prioritized? The authors echo calls for a community-driven approach that involves the breadth of the stakeholders.
These gaps point to urgent directions for future research. There is a need to create standardized methods that balance technical rigor with ethical considerations, ensuring transparency and inclusivity. Collaboration across stakeholders is essential to craft evaluations that measure performance and promote fairness and equity in AI deployment.