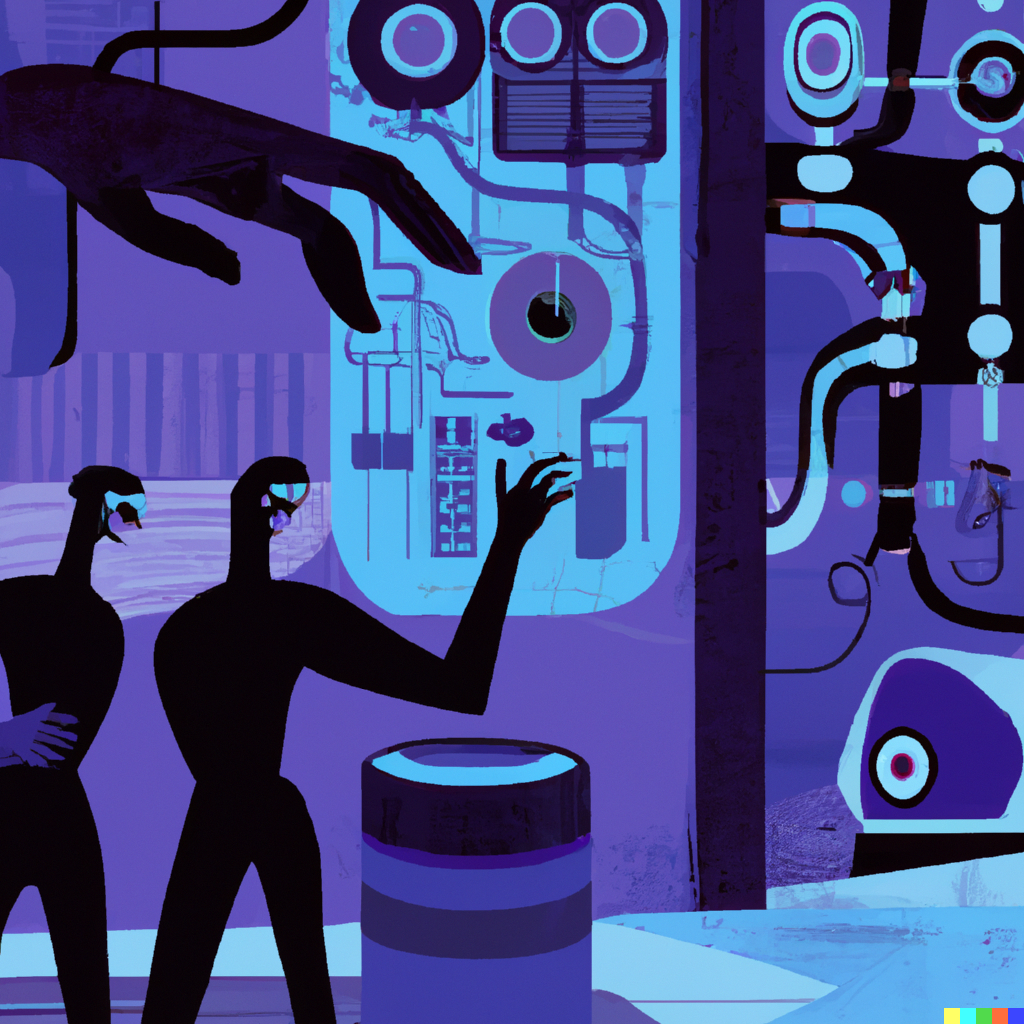
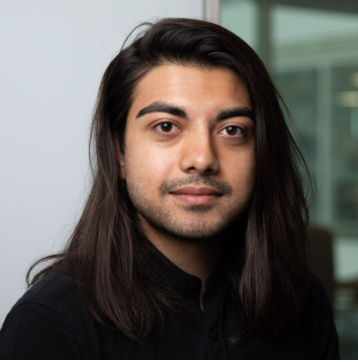
🔬 Research Summary by Advait Sarkar, an affiliate lecturer at the University of Cambridge, and honorary lecturer at UCL.
[Original paper by Advait Sarkar]
Overview: The term “human-AI collaboration” is misleading and inaccurate. It erases the labor of AI producers and obscures the often exploitative relationship between AI producers and consumers. Instead of viewing AI as a collaborator, we should view it as a tool or an instrument. This is more accurate and ultimately fairer to the humans who create and use AI.
Introduction
The term “human-AI collaboration” is everywhere these days. We hear it in the tech industry, in the media, and even in our everyday conversations. But what does it really mean? Does the “labor” we attribute to AI really represent work done by the machine?
The term “human-AI collaboration” obscures the often exploitative relationship between AI producers and consumers. To do what they do, AI systems require careful training and annotation by thousands of data workers. The majority of this AI labor is done by people in the Global South, who are often paid very little for their work. The term “collaboration” implies that AI producers and consumers are working together on equal footing when in reality, the relationship is often one-sided and unfair.
This is a cautionary tale about the dangers of misusing language. The term “human-AI collaboration” is a convenient metaphor, but it is also a dangerous one. It can lead us to overlook the real-world implications of our interactions with AI systems.
We need to be careful about how we talk about AI. The language we use shapes the way we think about AI, and the way we think about AI shapes the way we structure our societal divisions of labor, credit, attribution, and reward. If we want to use AI in a fair and equitable way, we need to start by using accurate and honest language.
Key Insights
Behind AI, a hidden workforce toils away, largely overlooked and underappreciated. Meet the data labelers – the unsung heroes behind the scenes of artificial intelligence. As we marvel at the magic of AI, we often forget that it’s not just the work of programmers and users but also the expertise of data labelers that fuels these intelligent systems.
AI systems learn from carefully labeled training data provided by human experts. But these data labelers, often from the Global South, are like ghost workers, hidden from view by those who seek to maintain their illusions of scalability and technological “magic.”
In an industry where the demand for labeled data is exploding, the grueling work of data annotation falls on the shoulders of those earning less than $30 a week in countries around the world, such as Kenya, India, and the Philippines, their creativity and skills reduced to mere datasets. Rather than being acknowledged as knowledge partners, they are treated as disposable commodities, subject to surveillance and punitive measures to improve data quality.
The consequences of this oversight are far-reaching. Despite promises of social inclusion and mobility, the reality is a dead-end for these workers, with little room for advancement beyond the hierarchical structures of the industry.
The result of work has often been separated from its source, with far-reaching consequences. The commodities which drove the era of colonialism: sugar, coffee, cocoa, cotton, and tobacco – were all packaged and presented sanitized for European consumers to distance the product from their horrifying origins. In artificial intelligence, we see this labor distancing at play again as AI systems replay human judgments made on the other side of the world.
The middlemen of AI software development profit from labor arbitrage, echoing past exploitations. As AI promises productivity, we must heed the call to agitate against labor distancing, recognizing the value and agency of those whose knowledge drives the machines.
Synthetic or open datasets won’t magically solve AI’s labor exploitation problem. While generating synthetic data or using freely accessible information from the internet seems like a remedy, it’s not foolproof. Synthetic datasets work best when there are clear mathematical models, like in computer graphics, but this is not the case for every scenario. Similarly, automatic feedback is efficient in constrained settings like games but lacks the flexibility needed for broader applications. Human-labeled data remains crucial for accurately representing desired behaviors. Open data, while seemingly harmless, conceals its own exploitation. Social media platforms profit from users who unwittingly become digital laborers, providing content without realizing they’re the product. The issue extends beyond labor, as these platforms capture behavioral information, anticipating and influencing future interests.
Using data from underrepresented communities can diversify AI, but it raises ethical questions of consent and representation.
Is AI truly a collaborator or just a tool? AI, after all, is not just any ordinary hammer or scalpel; it can write stories, generate stunning images, and now perform tasks beyond imagination just a few short years ago.
Instead, some call AI a “supertool” or “cognitive extender”. Yet, our desire to elevate AI to a higher status than other tools may stem from our own pride and human exceptionalism. We tend to value intelligence that resembles our own, overlooking the unique capabilities of AI and other forms of intelligence in the animal kingdom. The question remains: does AI truly understand the tasks we collaborate on? For now, the answer is no. AI lacks the rich sensorimotor context that humans have for acquiring language and meaning. But the media, academia, and industry are vested in presenting AI as something more than a tool.
It’s time to challenge this narrative and recognize that AI is indeed a tool, not a collaborator or partner. Let’s empower users without ignoring the labor behind AI and acknowledge that human-AI collaboration is, in fact, human-human collaboration distanced and disguised. The path to a fair and equitable future in AI begins with recognizing the true nature of this powerful tool.
Between the lines
The metaphor of human-AI collaboration suggests that we work together with AI systems as equals. In reality, intelligence in AI systems requires the labor of countless data annotators to work.
The article raises some important questions that warrant further research. For example, how can we ensure that AI systems are used in a way that is fair and equitable? How can we credit the humans who work behind the scenes to create AI systems? And how can we ensure that AI systems do not degrade the craftsmanship inherent in knowledge work?
The article suggests that we must be more careful about how we talk about human-AI collaboration. We need to be aware of the power imbalances that exist and find ways to ensure that AI systems are used in a way that benefits everyone.
Here are some additional directions for further research:
- How do people from different cultures and backgrounds understand the metaphor of human-AI collaboration?
- How does the metaphor of human-AI collaboration affect how people interact with AI systems?
By paying attention to the metaphors we use with AI systems, we can design systems that are more inclusive and beneficial to everyone.